Conditional Optimal Transport on Function Spaces
arXiv (Cornell University)(2023)
摘要
We present a systematic study of conditional triangular transport maps in function spaces from the perspective of optimal transportation and with a view towards amortized Bayesian inference. More specifically, we develop a theory of constrained optimal transport problems that describe block-triangular Monge maps that characterize conditional measures along with their Kantorovich relaxations. This generalizes the theory of optimal triangular transport to infinite-dimensional Hilbert spaces with general cost functions. We further tailor our results to the case of Bayesian inference problems and obtain regularity estimates on the conditioning maps from the prior to the posterior. Finally, we present numerical experiments that demonstrate the computational applicability of our theoretical results for amortized and likelihood-free Bayesian inverse problems.
更多查看译文
关键词
transport,optimal
AI 理解论文
溯源树
样例
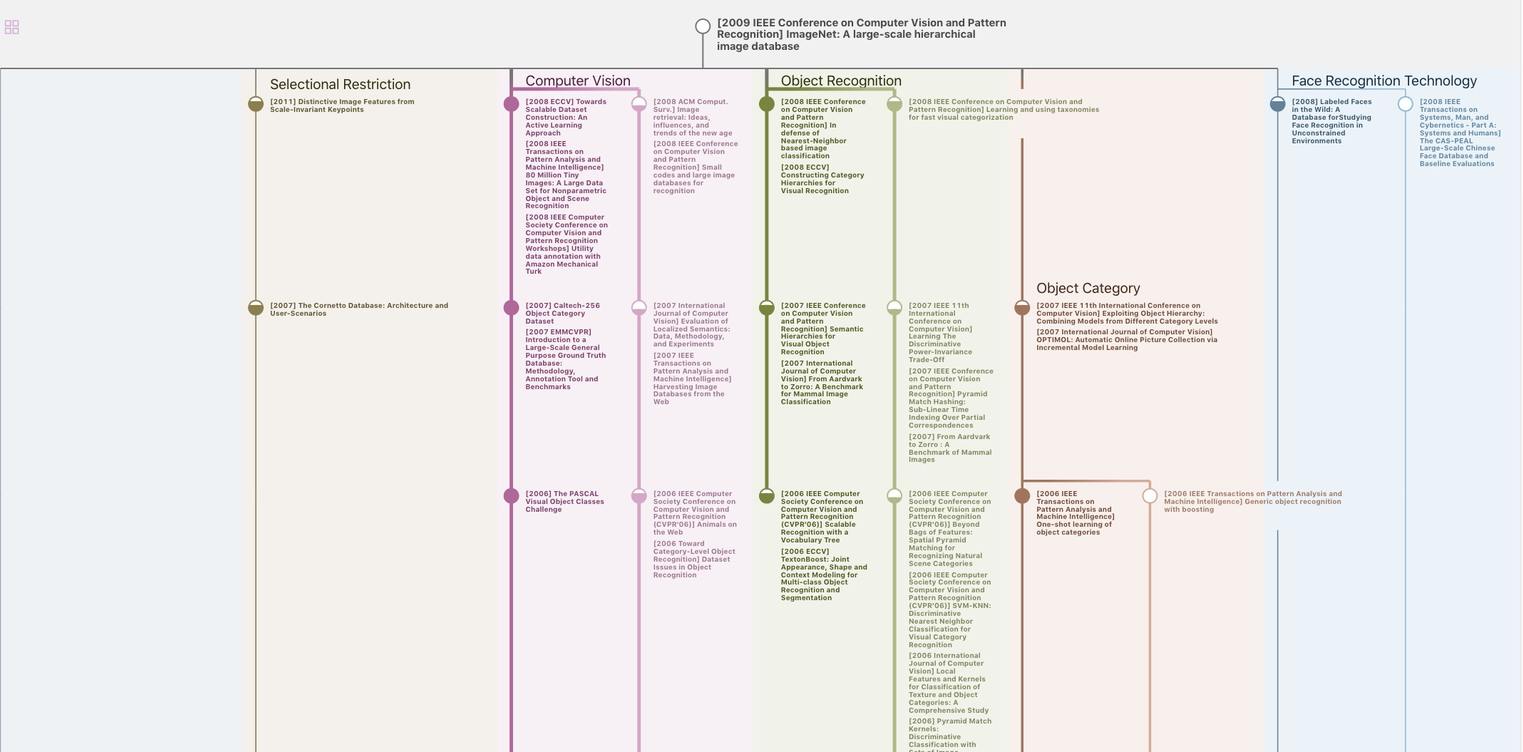
生成溯源树,研究论文发展脉络
Chat Paper
正在生成论文摘要