Generative Modeling of Residuals for Real-Time Risk-Sensitive Safety with Discrete-Time Control Barrier Functions
ICRA 2024(2024)
摘要
A key source of brittleness for robotic systems is the presence of model uncertainty and external disturbances. Existing approaches to robust control either seek to bound the worst-case disturbance (which results in conservative behavior), or to learn a deterministic dynamics model (which is unable to capture uncertain dynamics or disturbances). This work proposes a different approach: training a state-conditioned generative model to represent the distribution of errors between the nominal dynamics and the actual system. This learned disturbance model can be used in conjunction with probabilistic safety methods such as Discrete-Time Control Barrier Functions (DTCBFs) to ensure the safety of the system up to some level of risk. For this work, we focus on learning the dynamics uncertainties of a quadrotor drone, which is subject to complex aerodynamic interactions between the aircraft and the environment or which is carrying an unmodeled payload. We use a conditional variational autoencoder (CVAE) to learn a state-conditioned disturbance distribution, and find the resulting probabilistic safety controller exhibits less conservative behavior while retaining theoretical safety properties.
更多查看译文
关键词
Robot Safety,Machine Learning for Robot Control
AI 理解论文
溯源树
样例
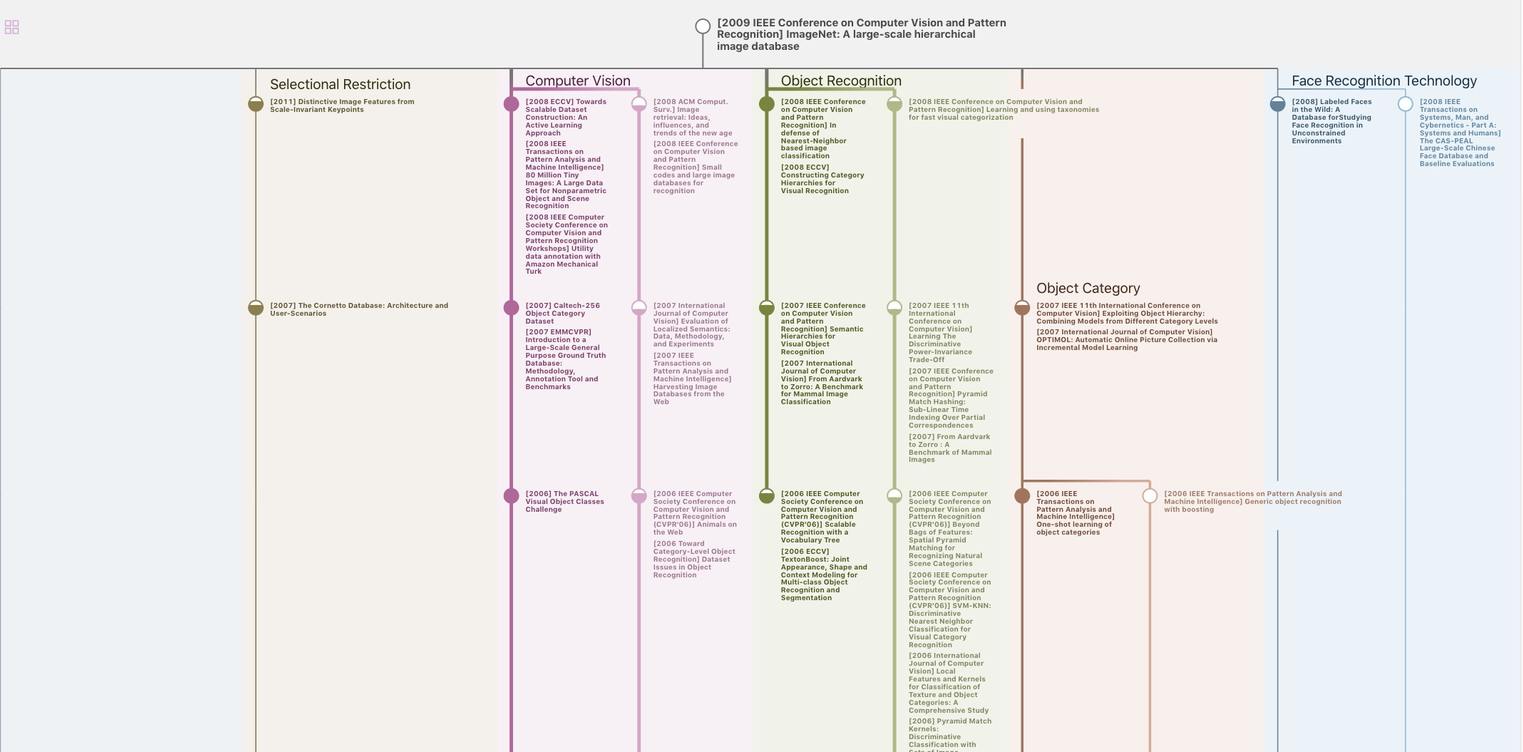
生成溯源树,研究论文发展脉络
Chat Paper
正在生成论文摘要