Fight Fire with Fire: Combating Adversarial Patch Attacks using Pattern-randomized Defensive Patches.
CoRR(2023)
摘要
Object detection has found extensive applications in various tasks, but it is also susceptible to adversarial patch attacks. Existing defense methods often necessitate modifications to the target model or result in unacceptable time overhead. In this paper, we adopt a counterattack approach, following the principle of "fight fire with fire," and propose a novel and general methodology for defending adversarial attacks. We utilize an active defense strategy by injecting two types of defensive patches, canary and woodpecker, into the input to proactively probe or weaken potential adversarial patches without altering the target model. Moreover, inspired by randomization techniques employed in software security, we employ randomized canary and woodpecker injection patterns to defend against defense-aware attacks. The effectiveness and practicality of the proposed method are demonstrated through comprehensive experiments. The results illustrate that canary and woodpecker achieve high performance, even when confronted with unknown attack methods, while incurring limited time overhead. Furthermore, our method also exhibits sufficient robustness against defense-aware attacks, as evidenced by adaptive attack experiments.
更多查看译文
关键词
adversarial patch attacks,defensive patches,pattern-randomized
AI 理解论文
溯源树
样例
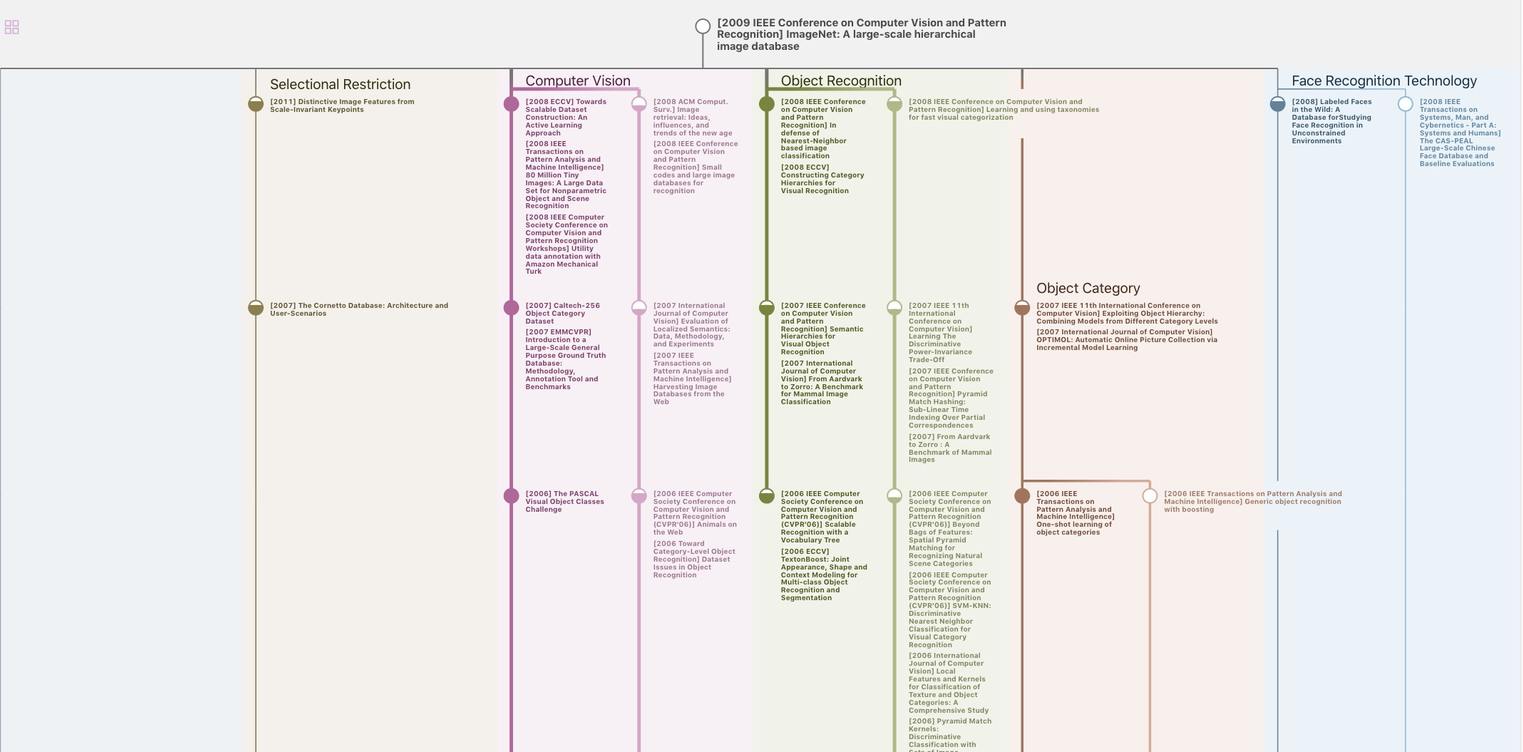
生成溯源树,研究论文发展脉络
Chat Paper
正在生成论文摘要