Learning characteristics of graph neural networks predicting protein–ligand affinities
Nature Machine Intelligence(2023)
摘要
In drug design, compound potency prediction is a popular machine learning application. Graph neural networks (GNNs) predict ligand affinity from graph representations of protein–ligand interactions typically extracted from X-ray structures. Despite some promising findings leading to claims that GNNs can learn details of protein–ligand interactions, such predictions are also controversially viewed. For example, evidence has been presented that GNNs might not learn protein–ligand interactions but memorize ligand and protein training data instead. We have carried out affinity predictions with six GNN architectures on community-standard datasets and rationalized the predictions using explainable artificial intelligence. The results confirm a strong influence of ligand—but not protein—memorization during GNN learning and also show that some GNN architectures increasingly prioritize interaction information for predicting high affinities. Thus, while GNNs do not comprehensively account for protein–ligand interactions and physical reality, depending on the model, they balance ligand memorization with learning of interaction patterns.
更多查看译文
关键词
protein–ligand affinities,graph,neural networks
AI 理解论文
溯源树
样例
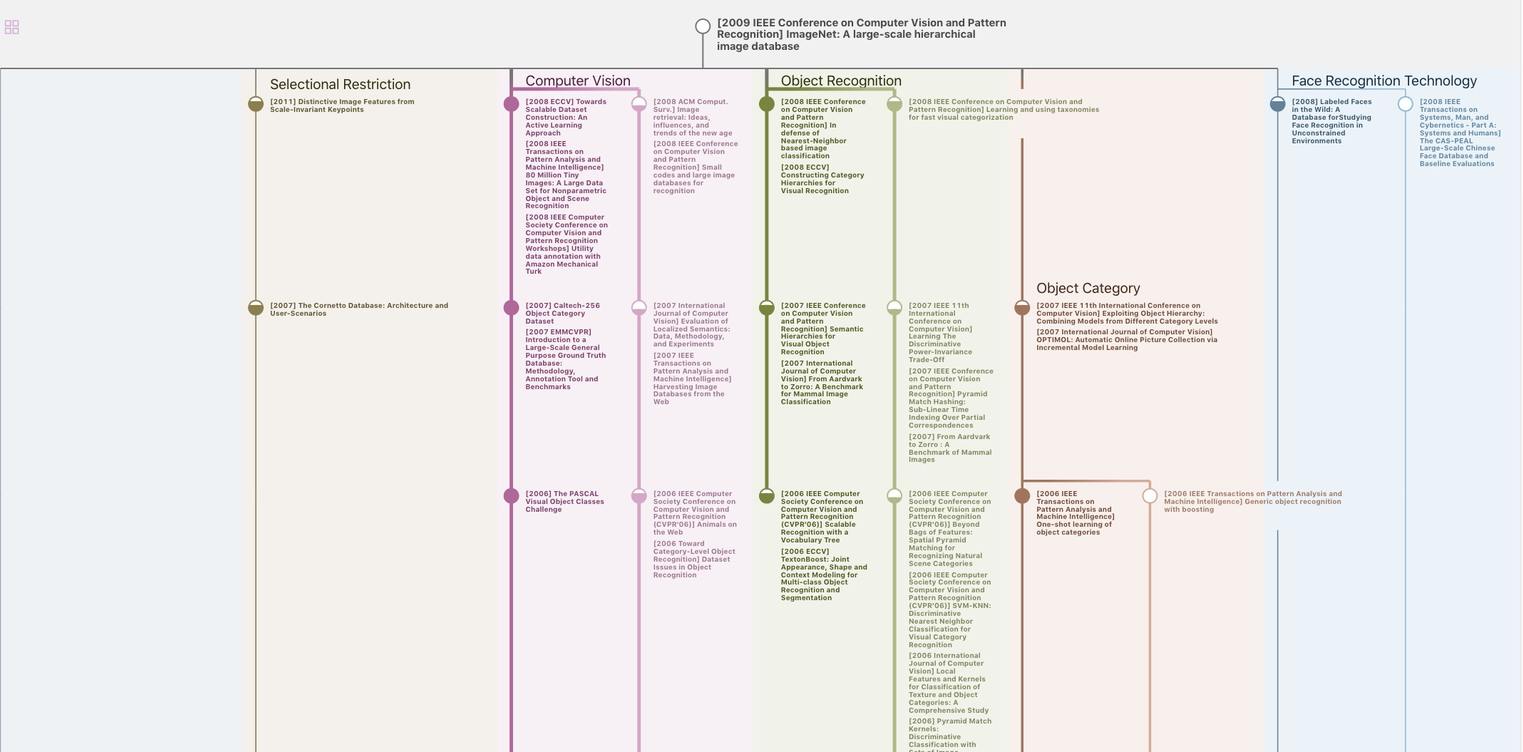
生成溯源树,研究论文发展脉络
Chat Paper
正在生成论文摘要