SPREd: a simulation-supervised neural network tool for gene regulatory network reconstruction
BIOINFORMATICS ADVANCES(2024)
摘要
Reconstruction of gene regulatory networks (GRNs) from expression data is a significant open problem. Common approaches train a machine learning (ML) model to predict a gene's expression using transcription factors' (TFs') expression as features and designate important features/TFs as regulators of the gene. Here, we present an entirely different paradigm, where GRN edges are directly predicted by the ML model. The new approach, named "SPREd," is a simulation-supervised neural network for GRN inference. Its inputs comprise expression relationships (e.g. correlation, mutual information) between the target gene and each TF and between pairs of TFs. The output includes binary labels indicating whether each TF regulates the target gene. We train the neural network model using synthetic expression data generated by a biophysics-inspired simulation model that incorporates linear as well as non-linear TF-gene relationships and diverse GRN configurations. We show SPREd to outperform state-of-the-art GRN reconstruction tools GENIE3, ENNET, PORTIA, and TIGRESS on synthetic datasets with high co-expression among TFs, similar to that seen in real data. A key advantage of the new approach is its robustness to relatively small numbers of conditions (columns) in the expression matrix, which is a common problem faced by existing methods. Finally, we evaluate SPREd on real data sets in yeast that represent gold-standard benchmarks of GRN reconstruction and show it to perform significantly better than or comparably to existing methods. In addition to its high accuracy and speed, SPREd marks a first step toward incorporating biophysics principles of gene regulation into ML-based approaches to GRN reconstruction.Availability and implementation Data and code are available from https://github.com/iiiime/SPREd.
更多查看译文
关键词
neural network tool,gene,neural network,simulation-supervised
AI 理解论文
溯源树
样例
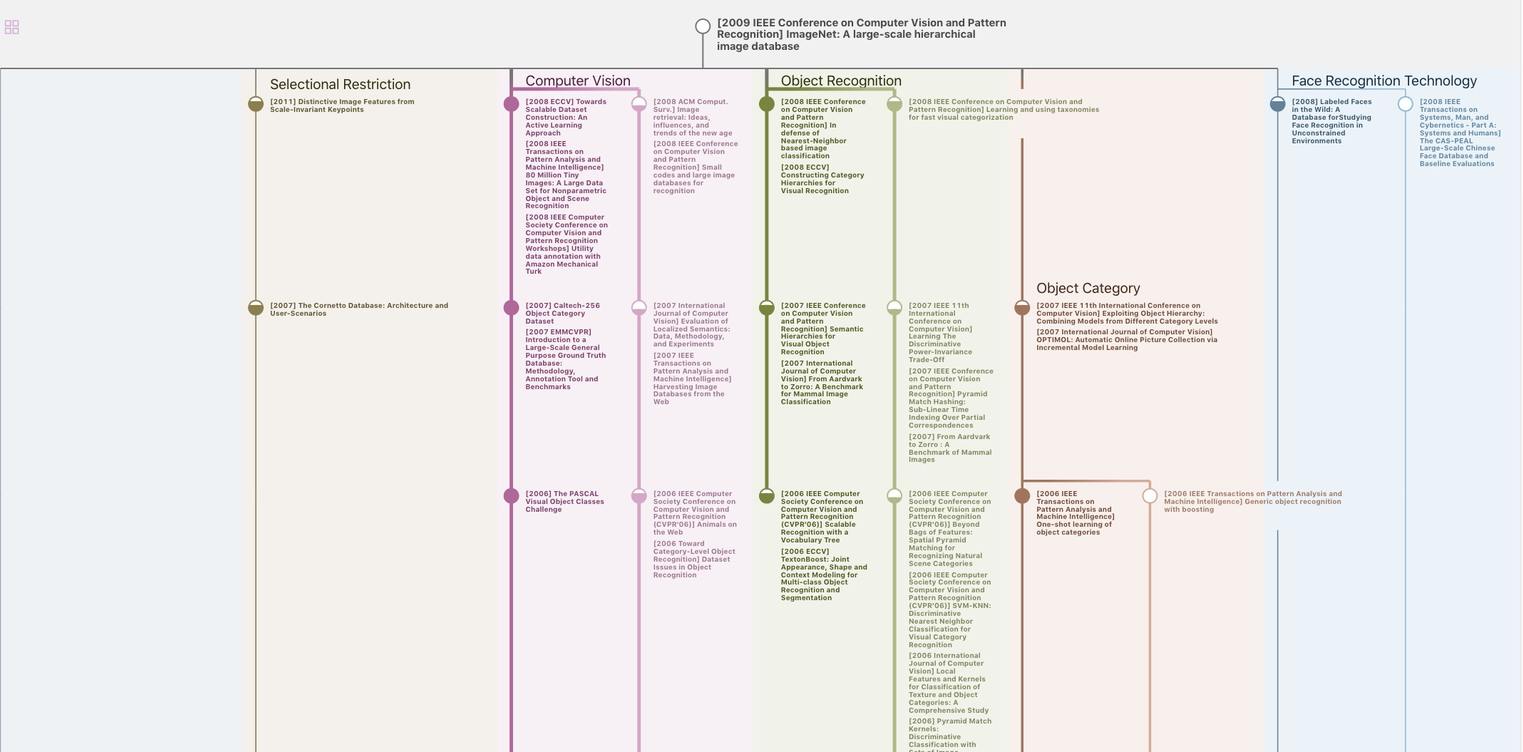
生成溯源树,研究论文发展脉络
Chat Paper
正在生成论文摘要