Retention Time Prediction through Learning from a Small Training Data Set with a Pretrained Graph Neural Network
ANALYTICAL CHEMISTRY(2023)
摘要
Graph neural networks (GNNs) have shown remarkable performance in predicting the retention time (RT) for small molecules. However, the training data set for a particular target chromatographic system tends to exhibit scarcity, which poses a challenge because the experimental process for measuring RT is costly. To address this challenge, transfer learning has been used to leverage an abundant training data set from a related source task. In this study, we present an improved transfer learning method to better predict the RT of molecules for a target chromatographic system by learning from a small training data set with a pretrained GNN. We use a graph isomorphism network as the architecture of the GNN. The GNN is pretrained on the METLIN-SMRT data set and is then fine-tuned on the target training data set for a fixed number of training iterations using the limited-memory Broyden-Fletcher-Goldfarb-Shanno optimizer with a learning rate decay. We demonstrate that the proposed method achieves superior predictive performance on various chromatographic systems compared with that of the existing transfer learning methods, especially when only a small training data set is available for use. A potential avenue for future research is to leverage multiple small training data sets from different chromatographic systems to further enhance the generalization performance.
更多查看译文
关键词
retention,small training data set,prediction,learning,graph
AI 理解论文
溯源树
样例
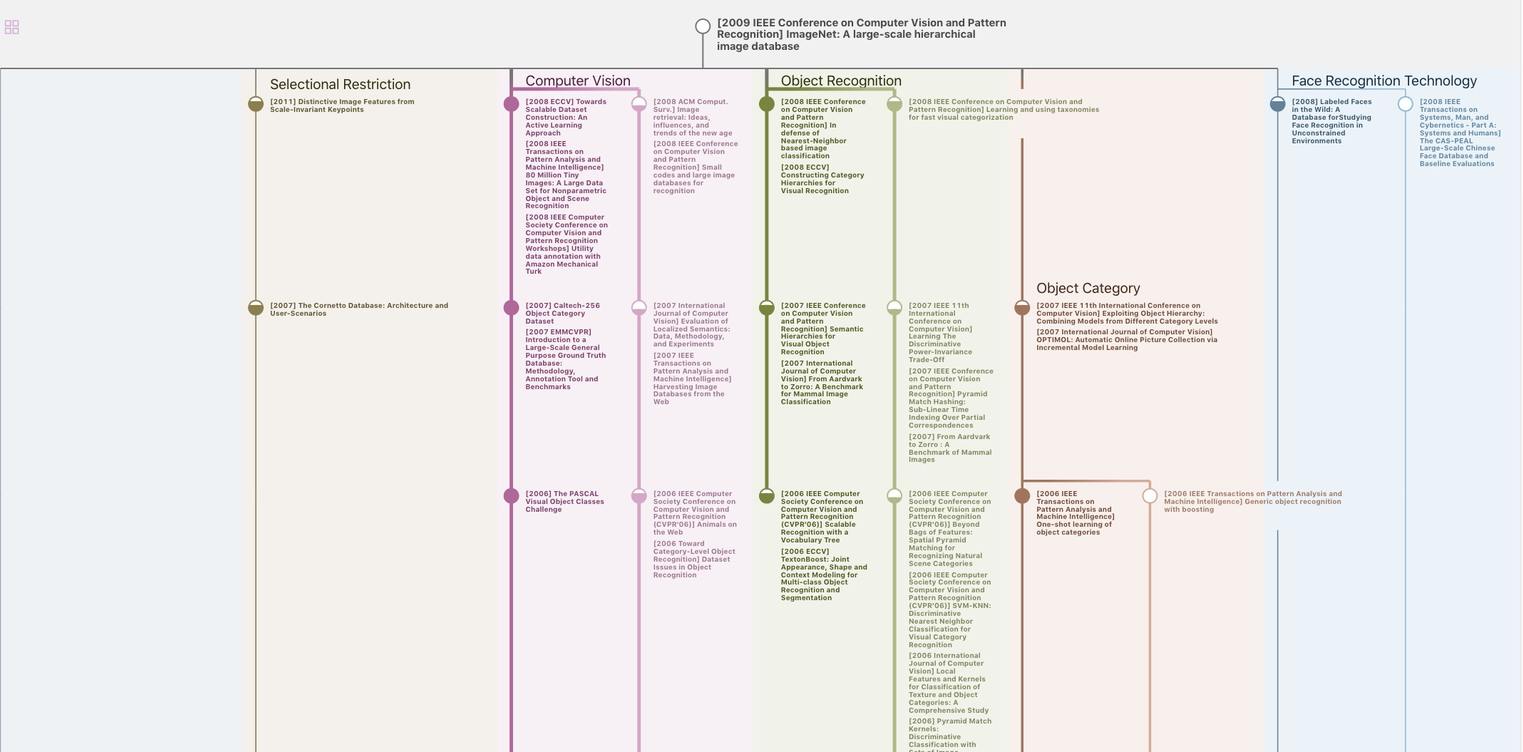
生成溯源树,研究论文发展脉络
Chat Paper
正在生成论文摘要