Computational and Statistical Thresholds in Multi-layer Stochastic Block Models
arXiv (Cornell University)(2023)
摘要
We study the problem of community recovery and detection in multi-layer stochastic block models, focusing on the critical network density threshold for consistent community structure inference. Using a prototypical two-block model, we reveal a computational barrier for such multi-layer stochastic block models that does not exist for its single-layer counterpart: When there are no computational constraints, the density threshold depends linearly on the number of layers. However, when restricted to polynomial-time algorithms, the density threshold scales with the square root of the number of layers, assuming correctness of a low-degree polynomial hardness conjecture. Our results provide a nearly complete picture of the optimal inference in multiple-layer stochastic block models and partially settle the open question in Lei and Lin (2022) regarding the optimality of the bias-adjusted spectral method.
更多查看译文
AI 理解论文
溯源树
样例
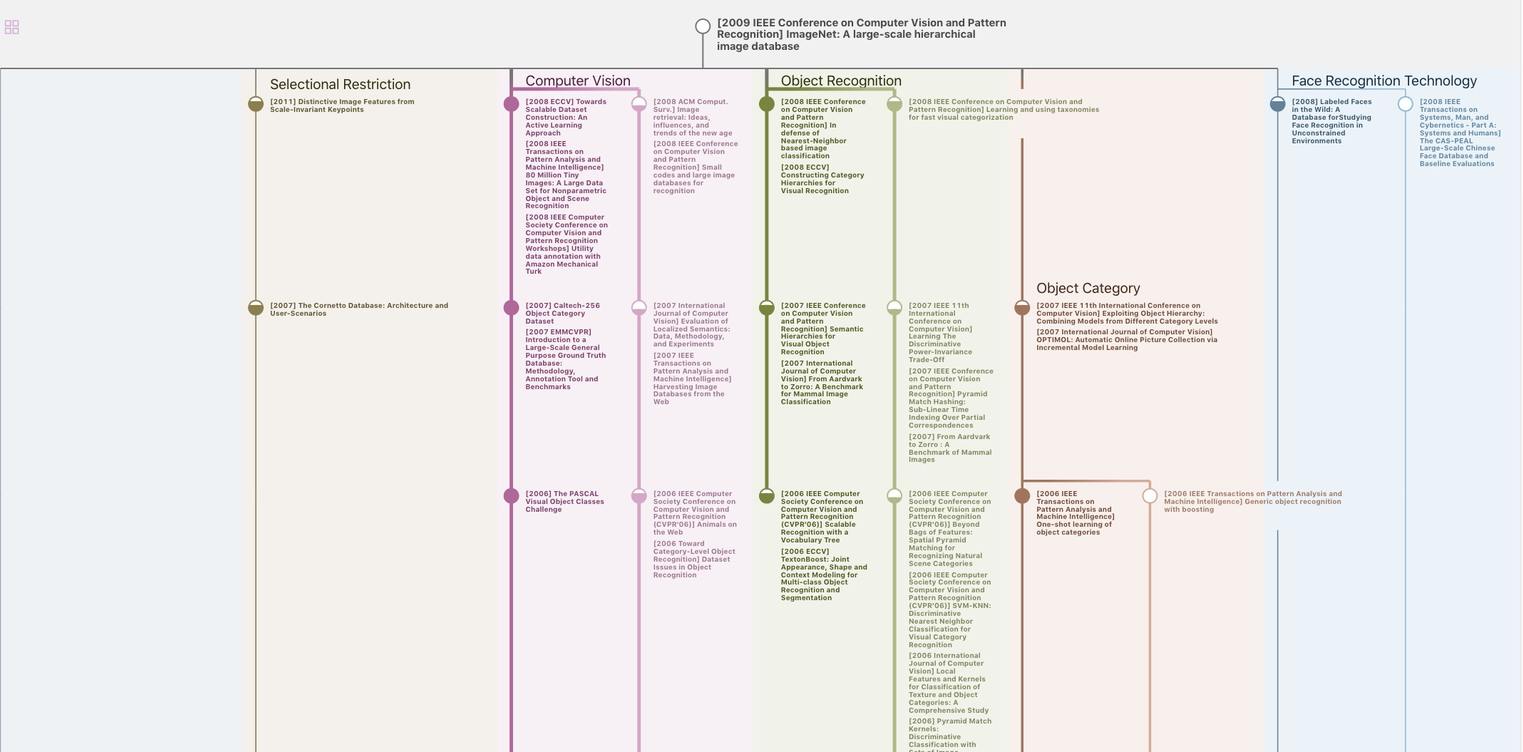
生成溯源树,研究论文发展脉络
Chat Paper
正在生成论文摘要