Quick and effective approximation of in silico saturation mutagenesis experiments with first-order Taylor expansion
bioRxiv (Cold Spring Harbor Laboratory)(2024)
摘要
To understand the decision process of genomic sequence-to-function models, various explainable AI algorithms have been proposed. These methods determine the importance of each nucleotide in a given input sequence to the model's predictions, and enable discovery of cis regulatory motif grammar for gene regulation. The most commonly applied method is in silico saturation mutagenesis (ISM) because its per-nucleotide importance scores can be intuitively understood as the computational counterpart to in vivo saturation mutagenesis experiments. While ISM is highly interpretable, it is computationally challenging to perform, because it requires computing three forward passes for every nucleotide in the given input sequence; these computations add up when analyzing a large number of sequences, and become prohibitive as the length of the input sequences and size of the model grows. Here, we show how to use the first-order Taylor approximation to compute ISM, which reduces its computation cost to a single forward pass for an input sequence. We use our theoretical derivation to connect ISM with the gradient of the model and show how this approximation is related to a recently suggested correction of the model's gradients for genomic sequence analysis. We show that the Taylor ISM (TISM) approximation is robust across different model ablations, random initializations, training parameters, and data set sizes.
### Competing Interest Statement
The authors have declared no competing interest.
更多查看译文
关键词
silico saturation mutagenesis experiments,effective approximation,expansion,first-order
AI 理解论文
溯源树
样例
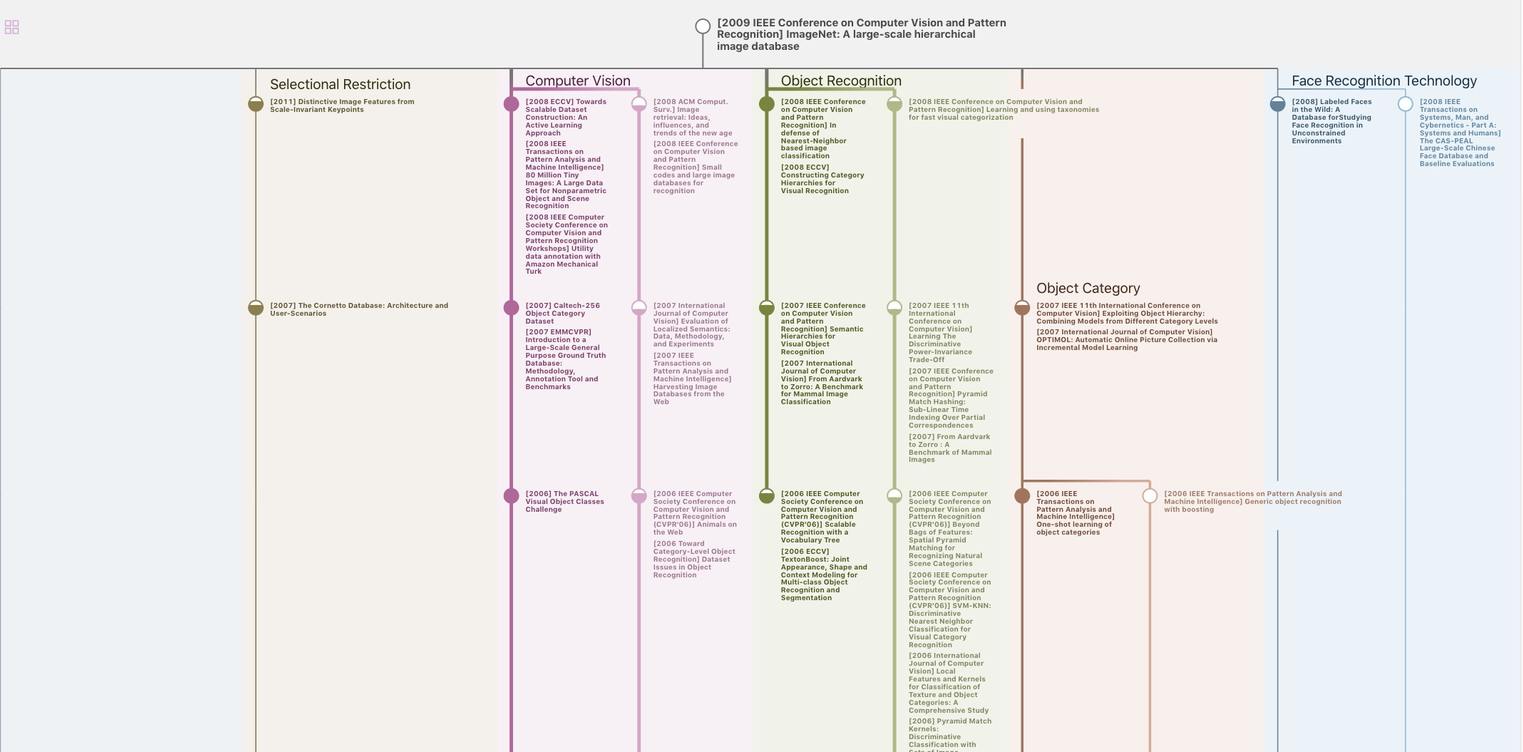
生成溯源树,研究论文发展脉络
Chat Paper
正在生成论文摘要