LWOSNet: A Lightweight One-Shot Network Framework for Object Pose Estimation
IEEE Sensors Journal(2023)
摘要
The 6-D pose estimation of objects is a crucial task for robotic manipulation. The currently popular methods, that is, deep learning-based methods, usually have high requirements on the training dataset and the network architecture, which is likely to increase the cost of data annotation and training time. In this article, we propose a lightweight one-shot network (LWOSNet) to estimate the 6-D poses of multiple objects in real time and provide two feasible routes to generate synthetic training data with the objects at hand. The input of LWOSNet is a red-green-blue (RGB) image, and the output is the objects' semantic labels and 6-D poses. The whole process is divided into three stages: the image pre-processing stage, the keypoints extraction stage, and the 6-D pose inference stage. Firstly, we leverage the first eight layers of visual geometry group 19 (VGG-19) and two convolutional layers to downscale the dimensionality of the image feature, which effectively reduces the parameters of the network. Then, the processed features are input into two different network branches to identify the categories of the objects and generate the 3-D bounding boxes. Finally, the LWOSNet outputs the semantic labels and the 6-D poses calculated by the perspective-n-point (PnP) algorithm. Additionally, we conducted a series of detection experiments and robot grasping experiments. The experimental results indicate that the LWOSNet accurately detects the categories and 6-D poses of multiple objects, and the robot successfully grasps the target objects based on this information.
更多查看译文
关键词
6-D pose estimation,lightweight one-shot network (LWOSNeT),robotic grasp,semantic label,synthetic data
AI 理解论文
溯源树
样例
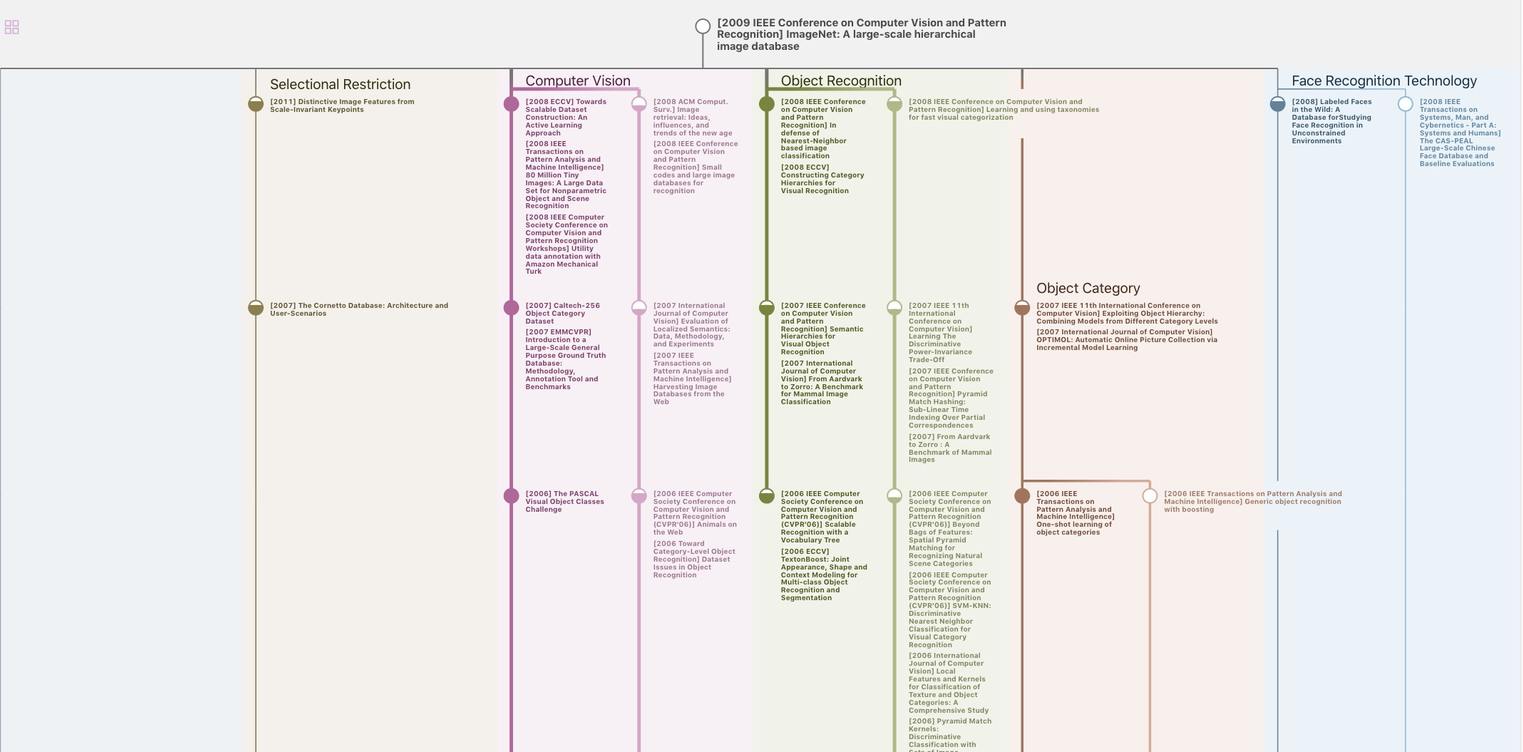
生成溯源树,研究论文发展脉络
Chat Paper
正在生成论文摘要