PillarNet++: Pillar-Based 3-D Object Detection With Multiattention
IEEE Sensors Journal(2023)
摘要
Light detection and ranging (LiDAR)-based 3-D object detection constitutes a fundamental component of autonomous driving technology. In this research, we propose a novel approach called PillarNet++ to tackle the challenges associated with fine-grained information loss during point cloud encoding and the inadequate interaction or incomplete fusion of feature maps across different scales in subsequent feature extraction stages, resulting in a decrease in partial occlusion and long-distance 3-D object detection accuracy, leading to false and missed detections. The PillarNet++ method primarily comprises two modules: the multiattention-pillar-encoding (MAPE) module and the pseudo-image-split-multibranch-feature-pyramid-network (PSMB-FPN) module. The MAPE module enhances the information extraction capability in nonempty pillars by integrating max pooling and average pooling, by fusion of the pointwise, channelwise, and pillarwise attention; the MAPE module can adaptively focus on the important information and suppress the secondary point clouds. In addition, the stacked MAPE modules can refine pillars and extract finer features. On the other hand, the PSMB-FPN module splits the pseudo-image along the channel dimension and subsequently performs MB-FPN feature extraction and fusion on each channel, facilitating the interaction of multiscale and multilevel feature maps and improving prediction accuracy. Experimental results on the KITTI 3-D object detection benchmark show that the PillarNet++ method has the best performance among single-stage object detection algorithms and even exceeds most two-stage methods.
更多查看译文
关键词
3-D object detection,autonomous driving,light detection and ranging (LiDAR),multiattention
AI 理解论文
溯源树
样例
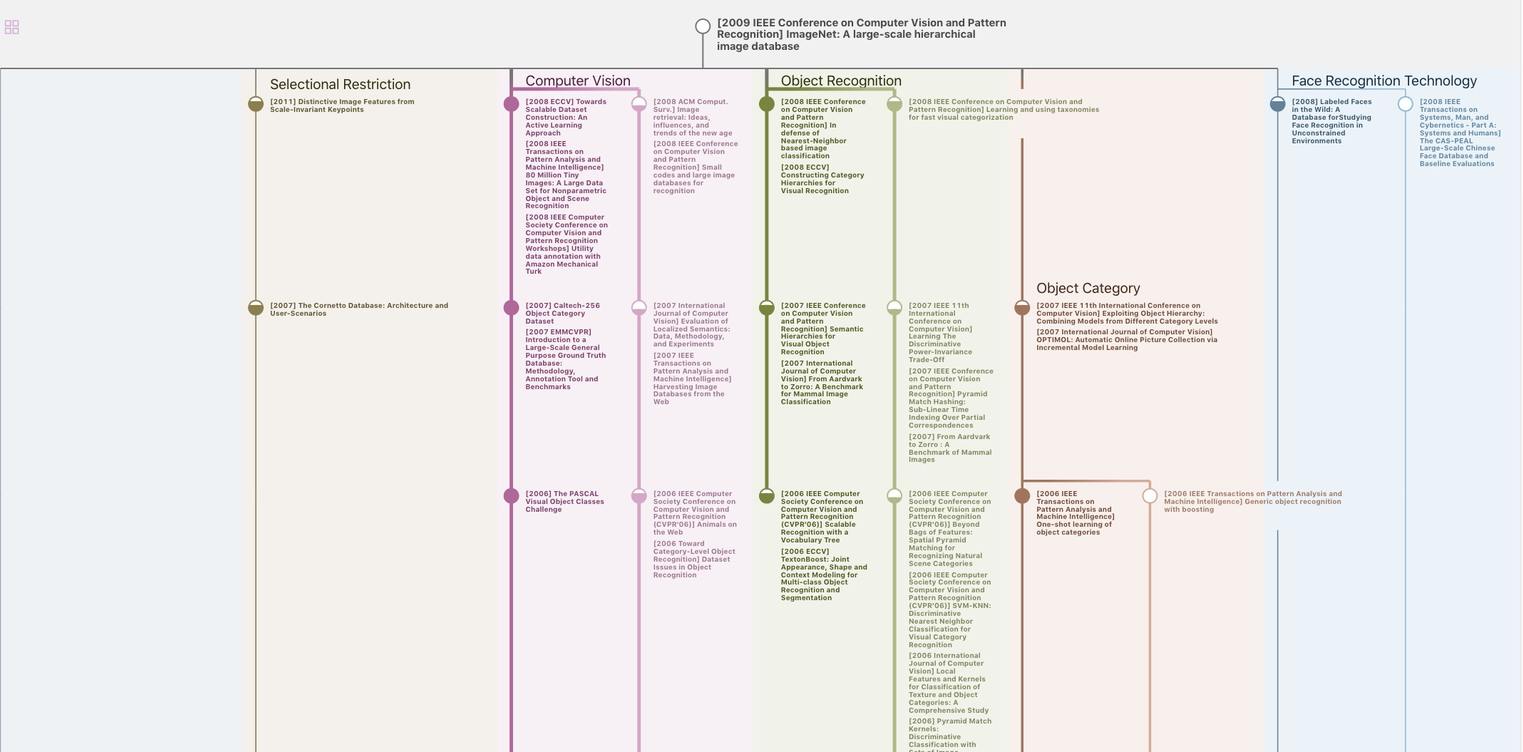
生成溯源树,研究论文发展脉络
Chat Paper
正在生成论文摘要