Design-time Reference Current Generation for Robust Spintronic-based Neuromorphic Architecture
ACM JOURNAL ON EMERGING TECHNOLOGIES IN COMPUTING SYSTEMS(2024)
摘要
Neural Networks (NN) can be efficiently accelerated in a neuromorphic fabric based on emerging resistive non-volatile memories (NVM), such as Spin Transfer Torque MagneticRAM(STT-MRAM). Compared to other NVM technologies, STT-MRAM offers many benefits, such as fast switching, high endurance, and CMOS process compatibility. However, due to its low ON/OFF-ratio, process variations and runtime temperature fluctuations can lead to miss-quantizing the sensed current and, in turn, degradation of inference accuracy. In this article, we analyze the impact of the sensed accumulated current variation on the inference accuracy in Binary NNs and propose a design-time reference current generation method to improve the robustness of the implemented NN under different temperature and process variation scenarios (up to 125. C). Our proposed method is robust to both process and temperature variations. The proposed method improves the accuracy of NN inference by up to 20.51% on the MNIST, Fashion-MNIST, and CIFAR-10 benchmark datasets in the presence of process and temperature variations without additional runtime hardware overhead compared to existing solutions.
更多查看译文
关键词
Computation-in-memory,STT-MRAM,neuromorphic computing,variations,robust neural network acceleration
AI 理解论文
溯源树
样例
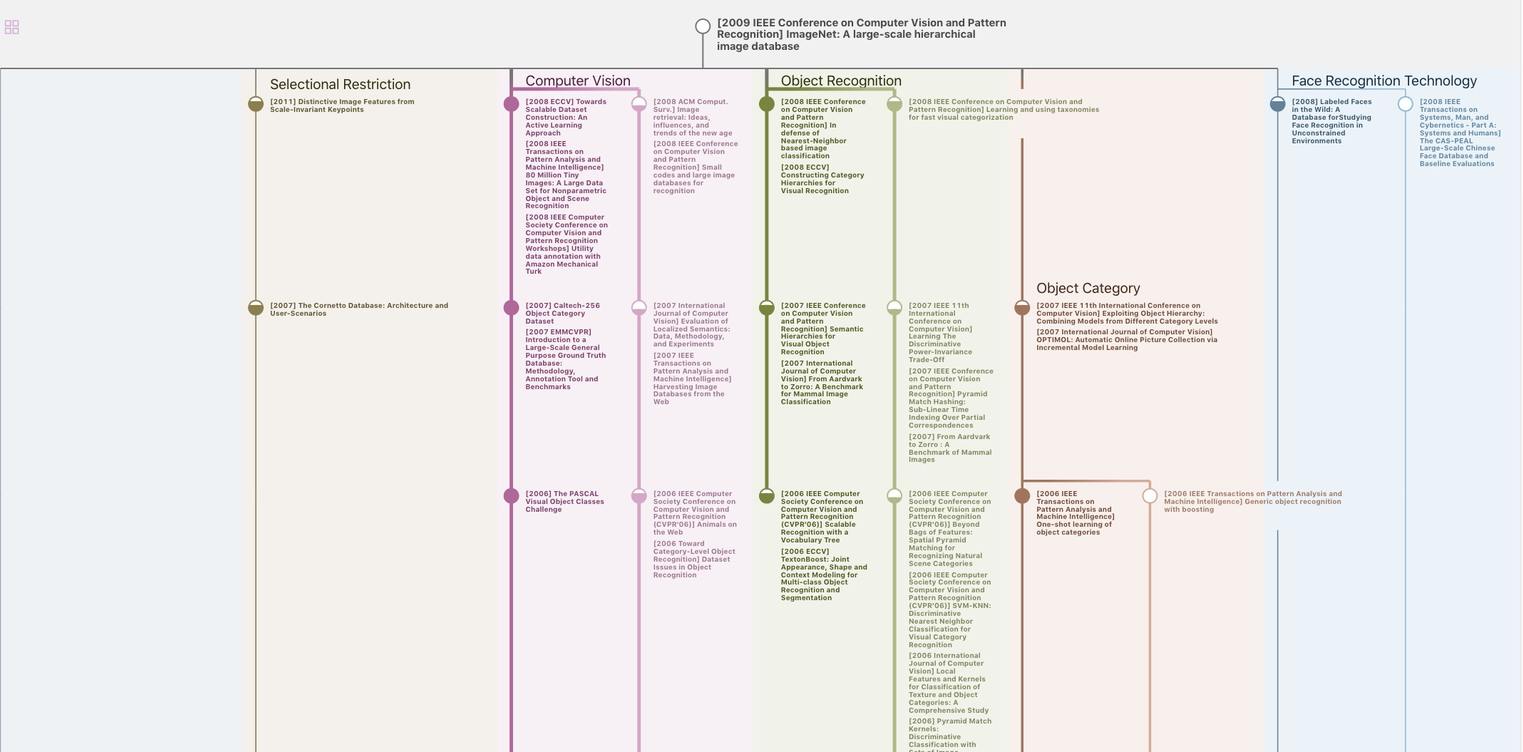
生成溯源树,研究论文发展脉络
Chat Paper
正在生成论文摘要