Ever: Mitigating Hallucination in Large Language Models through Real-Time Verification and Rectification
CoRR(2023)
摘要
Large Language Models (LLMs) have demonstrated remarkable proficiency in
generating fluent text. However, they often encounter the challenge of
generating inaccurate or hallucinated content. This issue is common in both
non-retrieval-based generation and retrieval-augmented generation approaches,
and existing post-hoc rectification methods may not address the accumulated
hallucination errors that may be caused by the "snowballing" issue, especially
in reasoning tasks. To tackle these challenges, we introduce a novel approach
called Real-time Verification and Rectification (Ever). Instead of waiting
until the end of the generation process to rectify hallucinations, Ever employs
a real-time, step-wise generation and hallucination rectification strategy. The
primary objective is to detect and rectify hallucinations as they occur during
the text generation process. When compared to both retrieval-based and
non-retrieval-based baselines, Ever demonstrates a significant improvement in
generating trustworthy and factually accurate text across a diverse range of
tasks, including short-form QA, biography generation, and multi-hop reasoning.
更多查看译文
AI 理解论文
溯源树
样例
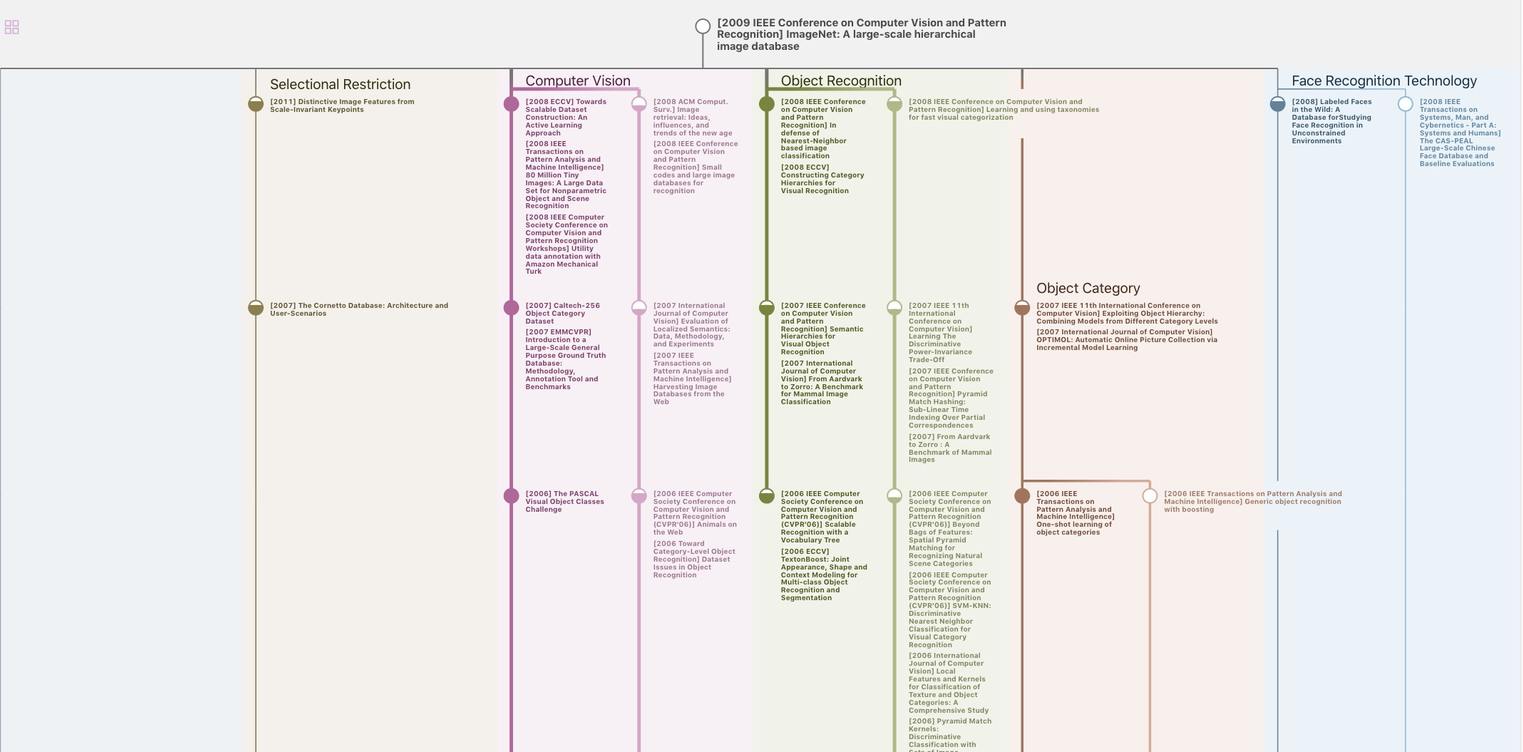
生成溯源树,研究论文发展脉络
Chat Paper
正在生成论文摘要