DMV3D: Denoising Multi-View Diffusion using 3D Large Reconstruction Model
ICLR 2024(2023)
Abstract
We propose \textbf{DMV3D}, a novel 3D generation approach that uses a transformer-based 3D large reconstruction model to denoise multi-view diffusion. Our reconstruction model incorporates a triplane NeRF representation and can denoise noisy multi-view images via NeRF reconstruction and rendering, achieving single-stage 3D generation in $\sim$30s on single A100 GPU. We train \textbf{DMV3D} on large-scale multi-view image datasets of highly diverse objects using only image reconstruction losses, without accessing 3D assets. We demonstrate state-of-the-art results for the single-image reconstruction problem where probabilistic modeling of unseen object parts is required for generating diverse reconstructions with sharp textures. We also show high-quality text-to-3D generation results outperforming previous 3D diffusion models. Our project website is at: https://justimyhxu.github.io/projects/dmv3d/ .
MoreTranslated text
Key words
3D Generation,Single-view 3D Reconstruction,text-to-3D
AI Read Science
Must-Reading Tree
Example
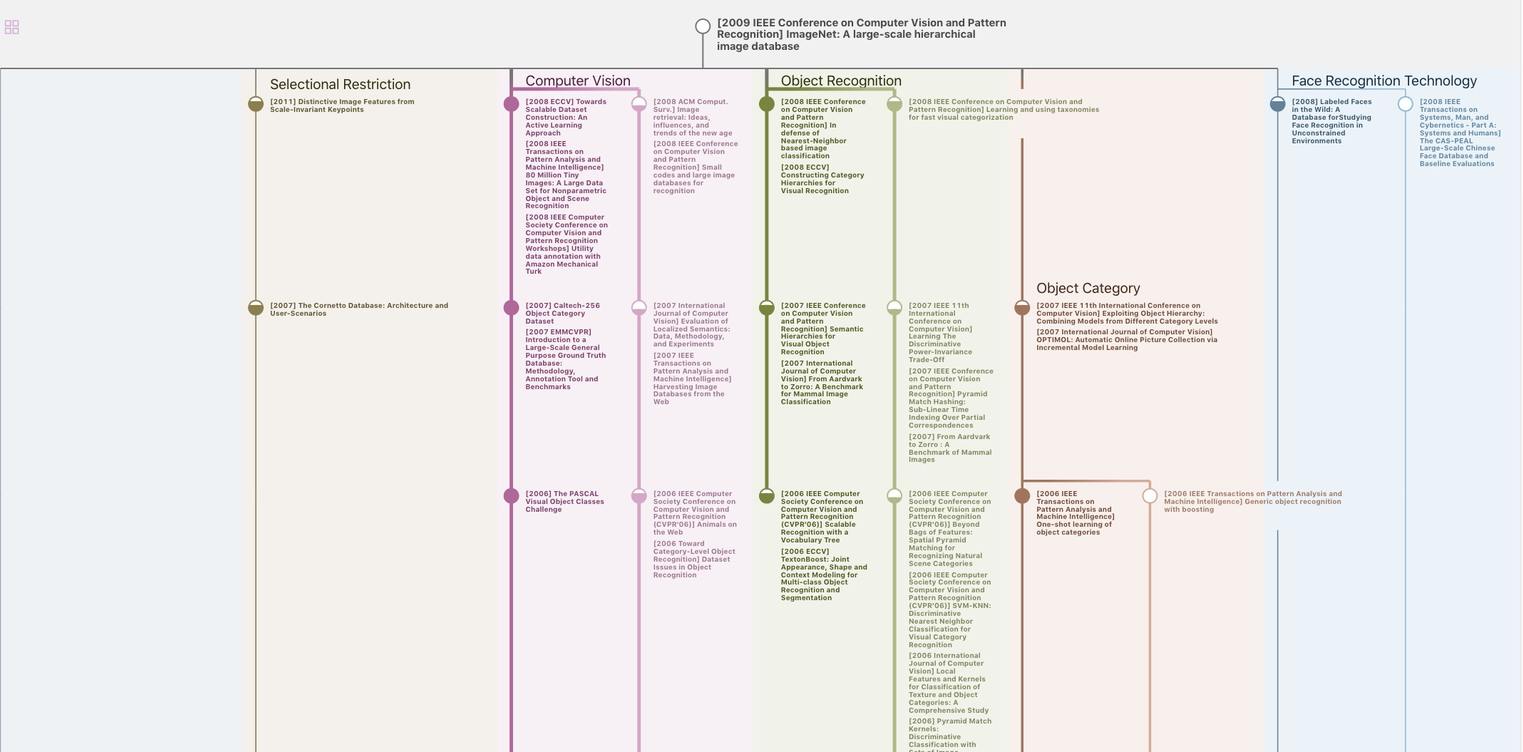
Generate MRT to find the research sequence of this paper
Chat Paper
Summary is being generated by the instructions you defined