Extreme Learning Machine (ELM) for Detection of Hazardous Near Earth Objects
SCCC(2023)
摘要
The protection of planet Earth, its inhabitants, and all living beings requires the identification of potentially dangerous objects, the simulation of impacts with Earth, and the mitigation of such threats. This research proposes the use of ELMs to distinguish between potentially dangerous objects and those that are not. The ELMs applied in this study include the standard ELM, the Regularized ELM, and the Weighted ELM (in versions W 1 and W 2 ). For the training and validation of the hazard object classification models, the “Nearest Earth Objects” database from NASA, available on Kaggle, was used. From this database, five features of the objects and a binary output indicating the danger or not towards Earth were used. The models were evaluated based on accuracy, geometric mean, and training time. According to the results, the Weighted ELM in its W 1 version offers the best performance, as it is capable of more effectively classifying dangerous and non-dangerous objects for Earth, with an accuracy of 0.8, a geometric mean of 0.7, and a training time of 1.8 seconds. Based on the results obtained, the viability of classifying whether objects are potentially dangerous for Earth is confirmed. However, to increase the performance of the models, it is recommended to continue exploring other types of ELMs.
更多查看译文
关键词
extreme learning machine,near Earth object,NASA,binary classification
AI 理解论文
溯源树
样例
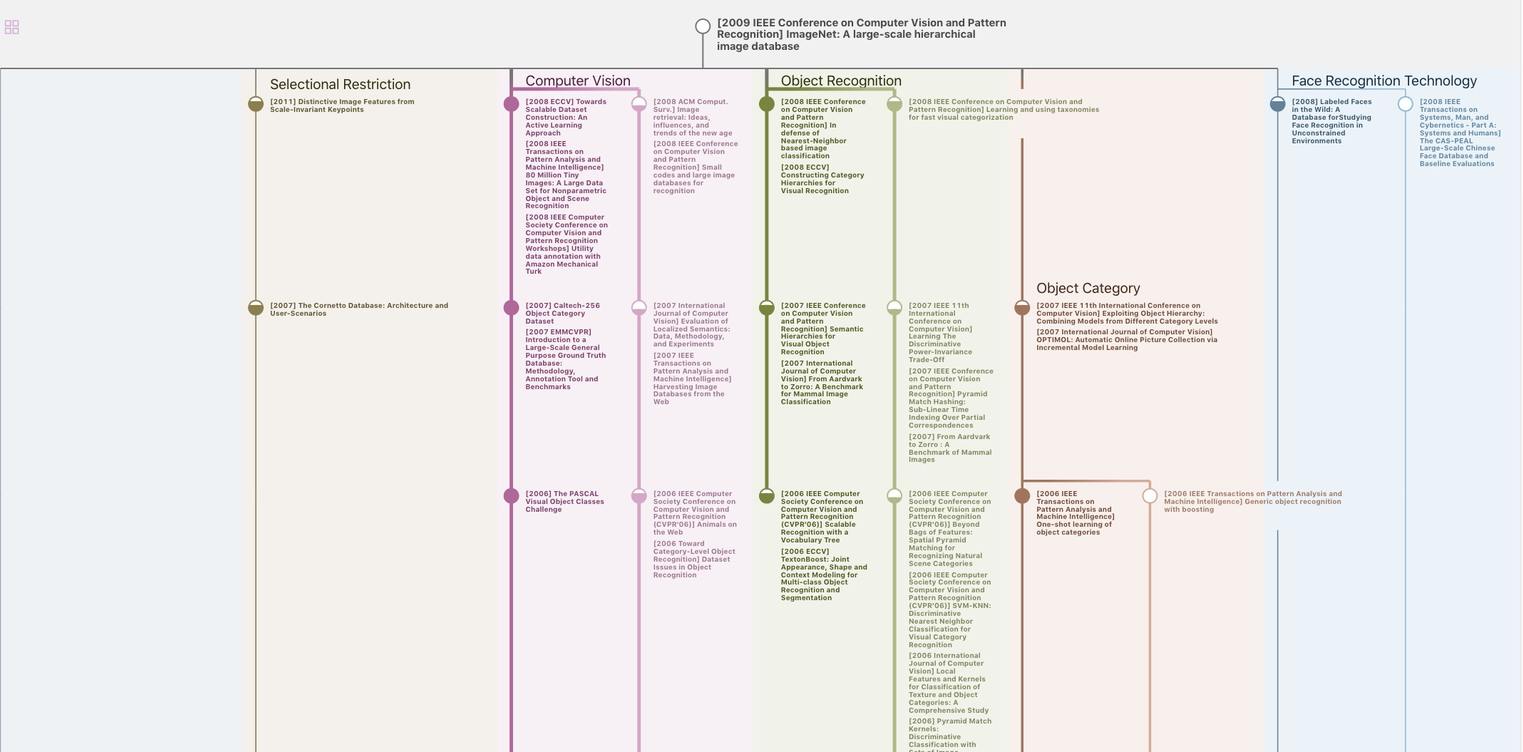
生成溯源树,研究论文发展脉络
Chat Paper
正在生成论文摘要