Predicting the transmission trend of respiratory viruses in new regions via geospatial similarity learning
INTERNATIONAL JOURNAL OF APPLIED EARTH OBSERVATION AND GEOINFORMATION(2023)
摘要
The outbreak and spread of COVID-19 remind us again of the devastating attack that human-to-human transmitted respiratory infectious diseases (H-HRIDs) bring to global economics and public health. Predicting the trend of H-HRIDs yields important suggestions for making response strategies. Existing methods predict the future trend of H-HRIDs based on the prophase epidemiological data. However, it is also crucial to predict the potential outbreak trend of H-HRIDs in regions without prophase epidemiological data based on those with epidemiological data available. This prediction can provide constructive pharmaceutical strategies and non-pharmaceutical interventions such as pre-allocating the limited preventions to regions with different potential trends, especially at the early stage of an H-HRID. In this paper, transform the problem of predicting the early-stage trend of an H-HRID in new regions without prophase epidemiological data into the problem of learning regions' geospatial features via a Contrastive learning-based Hierarchical Graph Convolutional Neural Network (CHGCN). Specifically, CHGCN first generates training data from regions with epidemiological data available via a Relaxed Graph Edit Distance (RGED) algorithm. CHGCN then uses a constructive learning schema to learn region embeddings, where regions with similar geospatial features and H-HRID trends exhibit similar embeddings. Once trained, CHGCN searches for the region with epidemiological data available that has the highest embedding similarity to the given query region without prophase epidemiological data, so as to achieve early-stage H-HRID trend prediction for the query region. Experimental results on COVID-19 demonstrate that the proposed CHGCN achieves state-of-the-art performance in predicting the early-stage trend of H-HRIDs, compared with the baselines.
更多查看译文
关键词
Human-to-human respiratory infectious disease,Spatial data analysis,Graph neural networks,Region similarity learning,COVID-19
AI 理解论文
溯源树
样例
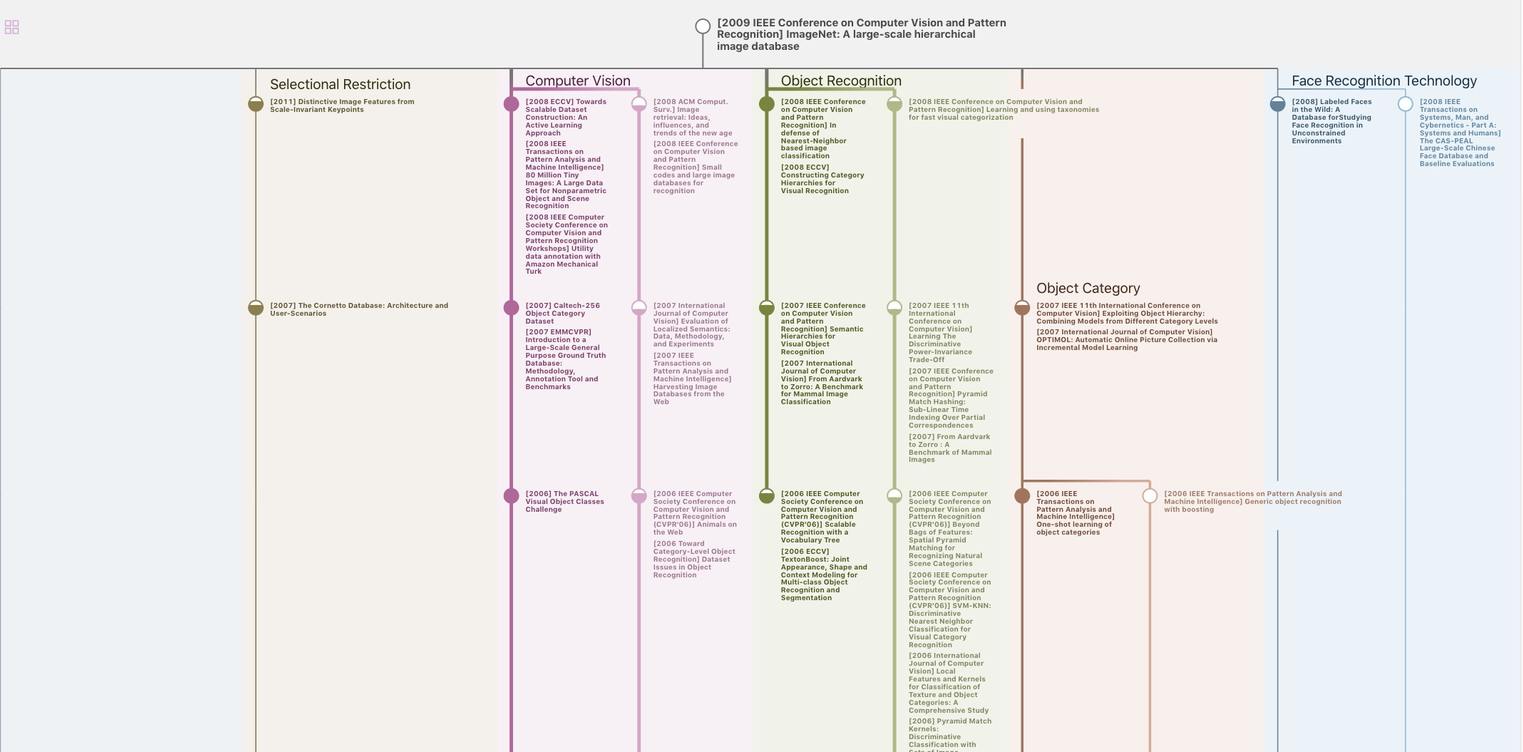
生成溯源树,研究论文发展脉络
Chat Paper
正在生成论文摘要