A Meta-learning based Method for Day Ahead Electricity Price Forecasting in Markets with Renewable Energy Resources
2023 IEEE 12th Global Conference on Consumer Electronics (GCCE)(2023)
摘要
Renewable energy is clean, sustainable, and affordable, but its growing integration increases the volatility in the price of electricity. However, many studies of electricity price forecasting are based on the market with large amounts of data and fail to consider the impact of renewable energy. We applied a meta-learning approach to predict 96-point day ahead electricity price in three spot electricity markets with renewable energy resources in China and compared the performances with Autoregressive Integrated Moving Average (ARIMA), Prophet, eXtreme Gradient Boosting (XGBoost), Light Gradient Boosting Machine (LightGBM) and Long Short-Term Memory (LSTM). The proposed model achieved the best performance with the mean absolute error (MAE) of 0.292 among the models applying different algorithms in the test dataset of three markets. The difference between proposed model and other base forecasters assessing by an independent Diebold-Mariano (DM) test was statistically significant. Exhibiting superior predictive performance, our novel model enables swift and accurate electricity market predictions, even in emerging markets with less than a year of historical data.
更多查看译文
关键词
renewable energy,electricity price forecasting,meta-learning,machine learning,deep learning
AI 理解论文
溯源树
样例
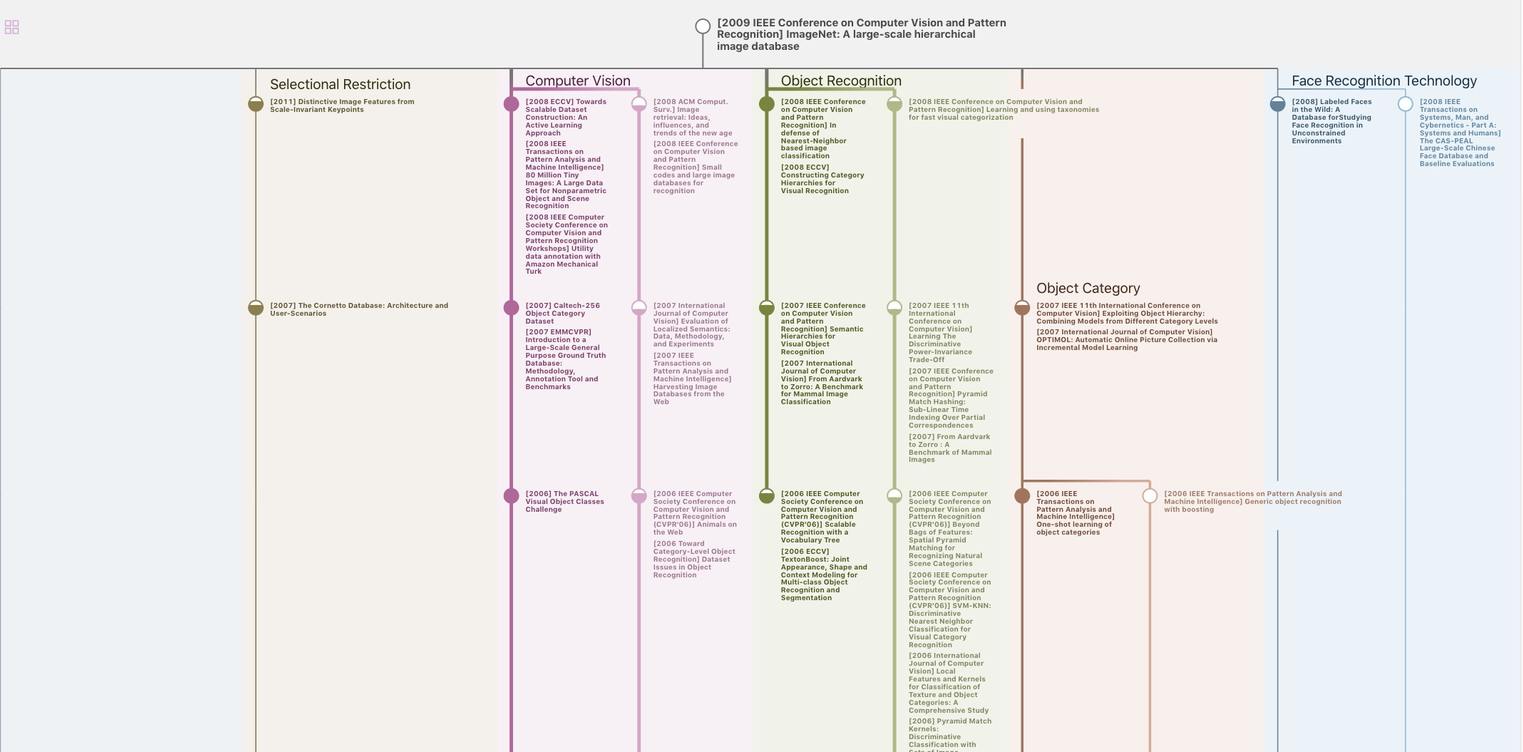
生成溯源树,研究论文发展脉络
Chat Paper
正在生成论文摘要