An Edge AI Accelerator Design Based on LRCN Model for Real-time EEG-based Emotion Detection System on the RISC-V FPGA Platform
2023 IEEE 12th Global Conference on Consumer Electronics (GCCE)(2023)
摘要
With the development of neural networks and big data, research on emotion recognition has gradually increased. In many ways of emotion recognition, we proposed using physiological signals such as Electroencephalography(EEG) to achieve high-precision emotion recognition. In this paper, the model we built was based on the concept of Long-term Recurrent Convolutional Networks(LRCN), which is used for emotion recognition of EEG signals. In addition, we achieved better performance in terms of area and speed by introducing and optimizing an AI architecture with high data reuse. Our structure adopt a strategy of high data reusability and can be reprocessed to various size of convolution layer and fully connected layer. The optimized architecture can achieve an peak performance of 128.2 GMACS/W, and uses only 196 KGates (NAND2) with average power consumption 0.039 mW in TSMC 90-nm.
更多查看译文
关键词
Affective Computing,Electroencephalogram,Accelerator,LRCN,Deep Learning
AI 理解论文
溯源树
样例
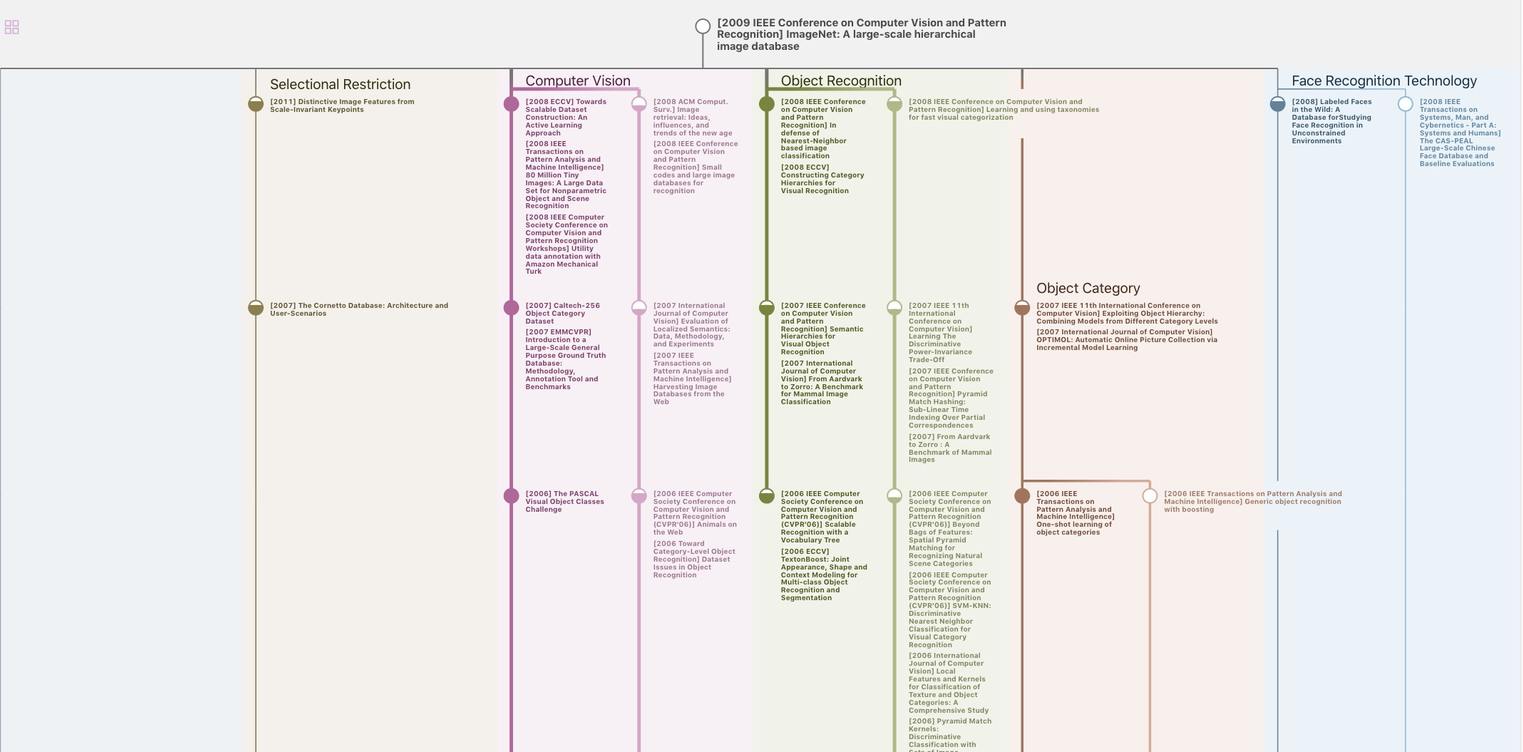
生成溯源树,研究论文发展脉络
Chat Paper
正在生成论文摘要