Reinforcement Learning Environment for Cyber-Resilient Power Distribution System
IEEE access(2023)
摘要
Recently, numerous data-driven approaches to control an electric grid using machine learning techniques have been investigated. Reinforcement learning (RL)-based techniques provide a credible alternative to conventional, optimization-based solvers especially when there is uncertainty in the environment, such as renewable generation or cyber system performance. Efficiently training an agent, however, requires numerous interactions with an environment to learn the best policies. There are numerous RL environments for power systems, and, similarly, there are environments for communication systems. Most cyber system simulators are based in a UNIX environment, while the power system simulators are based in the Windows operating system. Hence the generation of a cyber-physical, mixed-domain RL environment has been challenging. Existing co-simulation methods are efficient, but are resource and time intensive to generate large-scale data sets for training RL agents. Hence, this work focuses on the development and validation of a mixed-domain RL environment using OpenDSS for the power system and leveraging a discrete event simulator Python package, SimPy for the cyber system, which is operating system agnostic. Further, we present the results of co-simulation and training RL agents for a cyber-physical network reconfiguration and Volt-Var control problem in a power distribution feeder.
更多查看译文
关键词
Reinforcement learning,OpenDSS,SimPy,OpenAI gym,network reconfiguration,re-routing
AI 理解论文
溯源树
样例
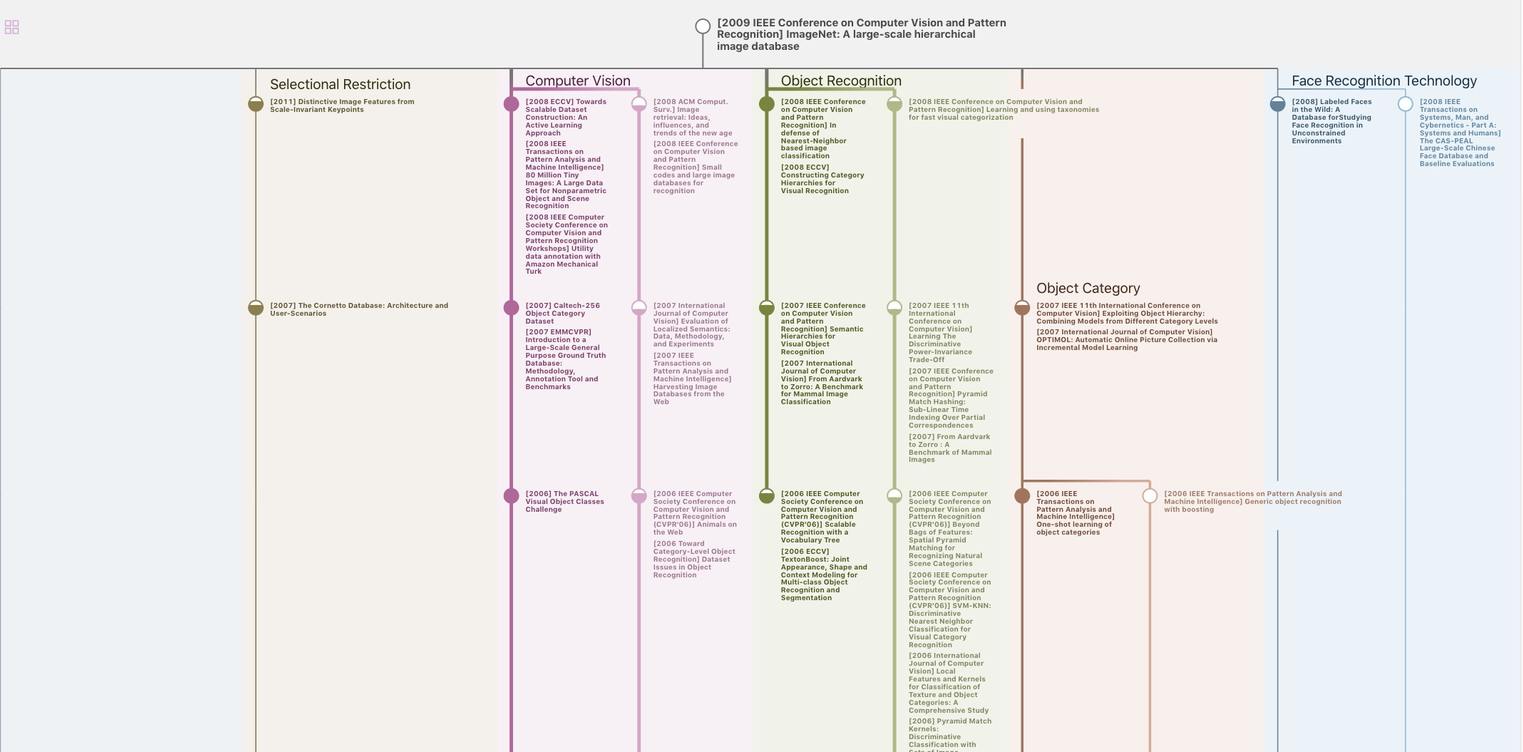
生成溯源树,研究论文发展脉络
Chat Paper
正在生成论文摘要