Comparing neural models using their perceptual discriminability predictions
bioRxiv (Cold Spring Harbor Laboratory)(2023)
摘要
Internal representations are not uniquely identifiable from perceptual measurements: different representations can generate identical perceptual predictions, and similar representations may predict dissimilar percepts. Here, we generalize a previous method ("Eigendistortions" - Berardino et al., 2017) to enable comparison of models based on their metric tensors, which can be verified perceptually. Metric tensors characterize sensitivity to stimulus perturbations, reflecting both the geometric and stochastic properties of the representation, and providing an explicit prediction of perceptual discriminability. Brute force comparison of model predicted metric tensors would require estimation of human perceptual thresholds along an infeasibly large set of stimulus directions. To circumvent this "perceptual curse of dimensionality", we compute and measure discrimination capabilities for a small set of most-informative perturbations, reducing the measurement cost from thousands of hours (a conservative estimate) to a single trial. We show that this single measurement, made for a variety of different test stimuli, is sufficient to differentiate models, select models that better match human perception, or generate new models that combine the advantages of existing models. We demonstrate the power of this method in comparison of (1) two models for trichromatic color representation, with differing internal noise; and (2) two autoencoders trained with different regularizers.
### Competing Interest Statement
The authors have declared no competing interest.
更多查看译文
AI 理解论文
溯源树
样例
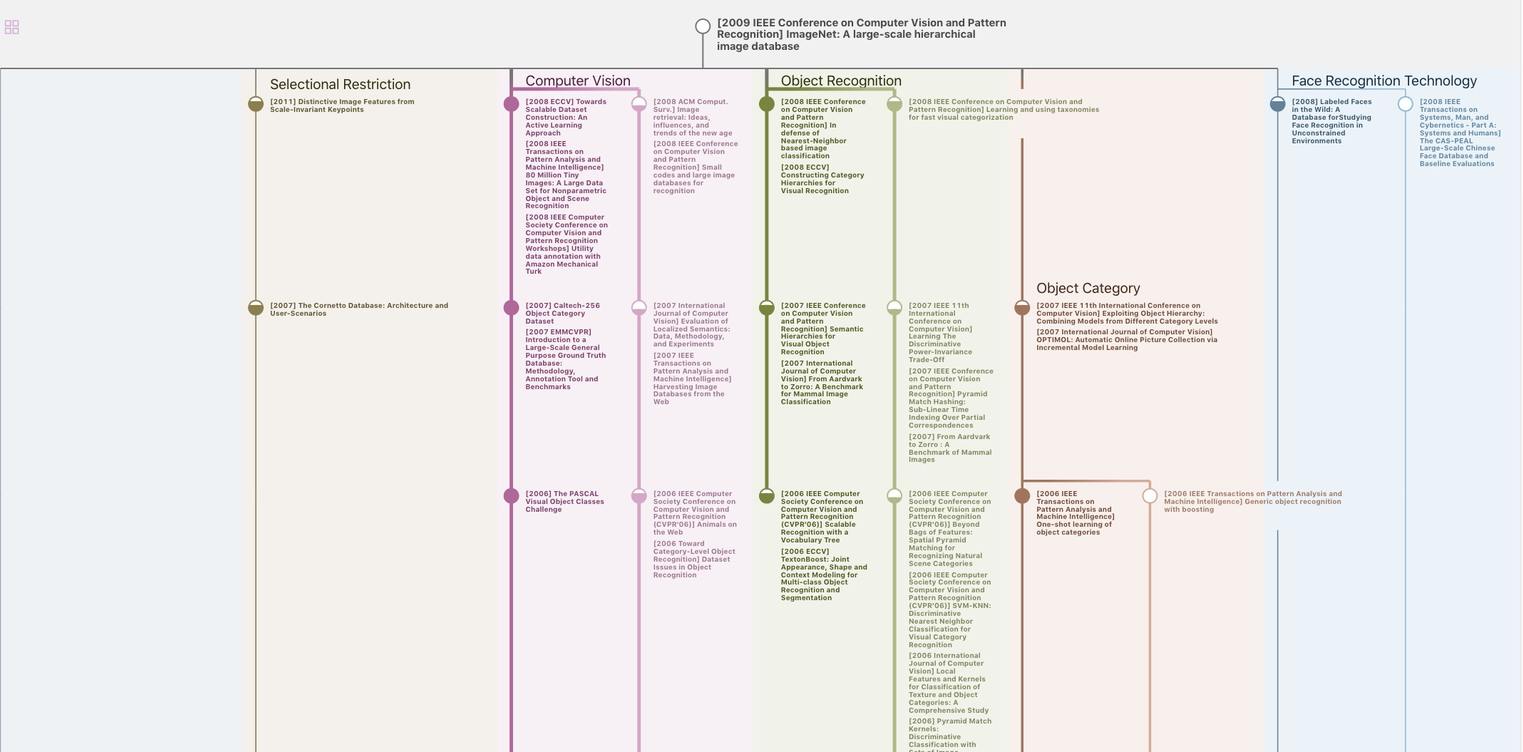
生成溯源树,研究论文发展脉络
Chat Paper
正在生成论文摘要