Performance Analysis of SVM-Based DOA Estimation for Uniform Linear Arrays
2023 IEEE 14th Annual Ubiquitous Computing, Electronics & Mobile Communication Conference (UEMCON)(2023)
摘要
Support vector machine (SVM) is a powerful machine learning algorithm which has been used for classification and regression in array signal processing. This paper investigates the relationship between the SVM classification accuracy and different signal and sensor parameters. For a uniform linear array (ULA), we consider the classification of two plane waves with different directions of arrival using the SVM algorithm. We vary the number of sensors, signal-to-noise ratio (SNR), and number of snapshots to examine their impacts on the classification accuracy. The simulation results show that increasing the number of sensors or SNR increases the accuracy, as expected. In low SNR regions, the accuracy values fluctuate substantially and are highly nonlinear functions of the number of snapshots. As the SNR level increases, the accuracy increases while the fluctuation in their values decreases. We express the accuracy as a polynomial function of the number of snapshots for fixed SNR values, using simulation data points. The simulation results show that increasing the SNR level improves the polynomial fitting. We also employed and compared three different SVM kernel functions: linear, polynomial, and radial basis function (RBF). The linear kernel provides the worst performance. Although the polynomial kernel shows superior performance compared to the RBF kernel, it takes significantly longer simulation time compared to the RBF kernel.
更多查看译文
关键词
Classification,curve fitting,direction of arrival,kernel,polynomial,radial basis function,support vector machine
AI 理解论文
溯源树
样例
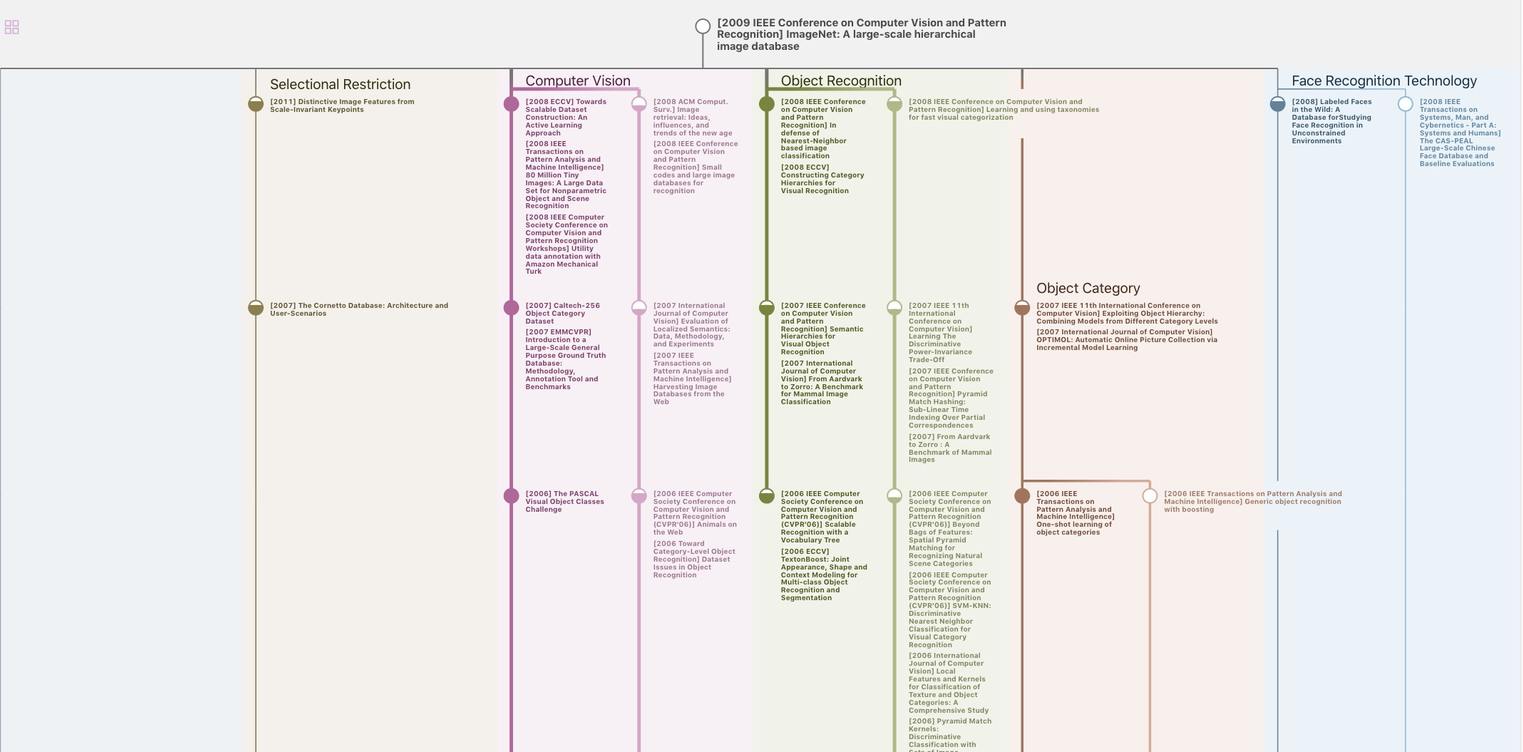
生成溯源树,研究论文发展脉络
Chat Paper
正在生成论文摘要