Distance-Aware Learning for Inductive Link Prediction on Temporal Networks
IEEE TRANSACTIONS ON NEURAL NETWORKS AND LEARNING SYSTEMS(2023)
摘要
Inductive link prediction on temporal networks aims to predict the future links associated with node(s) unseen in the historical timestamps. Existing methods generate the predictions mainly by learning node representation from the node/edge attributes as well as the network dynamics or by measuring the distance between nodes on the temporal network structure. However, the attribute information is unavailable in many realistic applications and the structure-aware methods highly rely on nodes' common neighbors, which are difficult to accurately detect, especially in sparse temporal networks. Thus, we propose a distance-aware learning (DEAL) approach for inductive link prediction on temporal networks. Specifically, we first design an adaptive sampling method to extract temporal adaptive walks for nodes, increasing the probability of including the common neighbors between nodes. Then, we design a dual-channel distance measuring component, which simultaneously measures the distance between nodes in the embedding space and on the dynamic graph structure for predicting future inductive edges. Extensive experiments are conducted on three public temporal network datasets, i.e., MathOverflow, AskUbuntu, and StackOverflow. The experimental results validate the superiority of DEAL over the state-of-the-art baselines in terms of accuracy, area under the ROC curve (AUC), and average precision (AP), where the improvements are especially obvious in scenarios with only limited data.
更多查看译文
关键词
Adaptive sampling,distance-aware learning (DEAL),inductive link prediction,temporal network
AI 理解论文
溯源树
样例
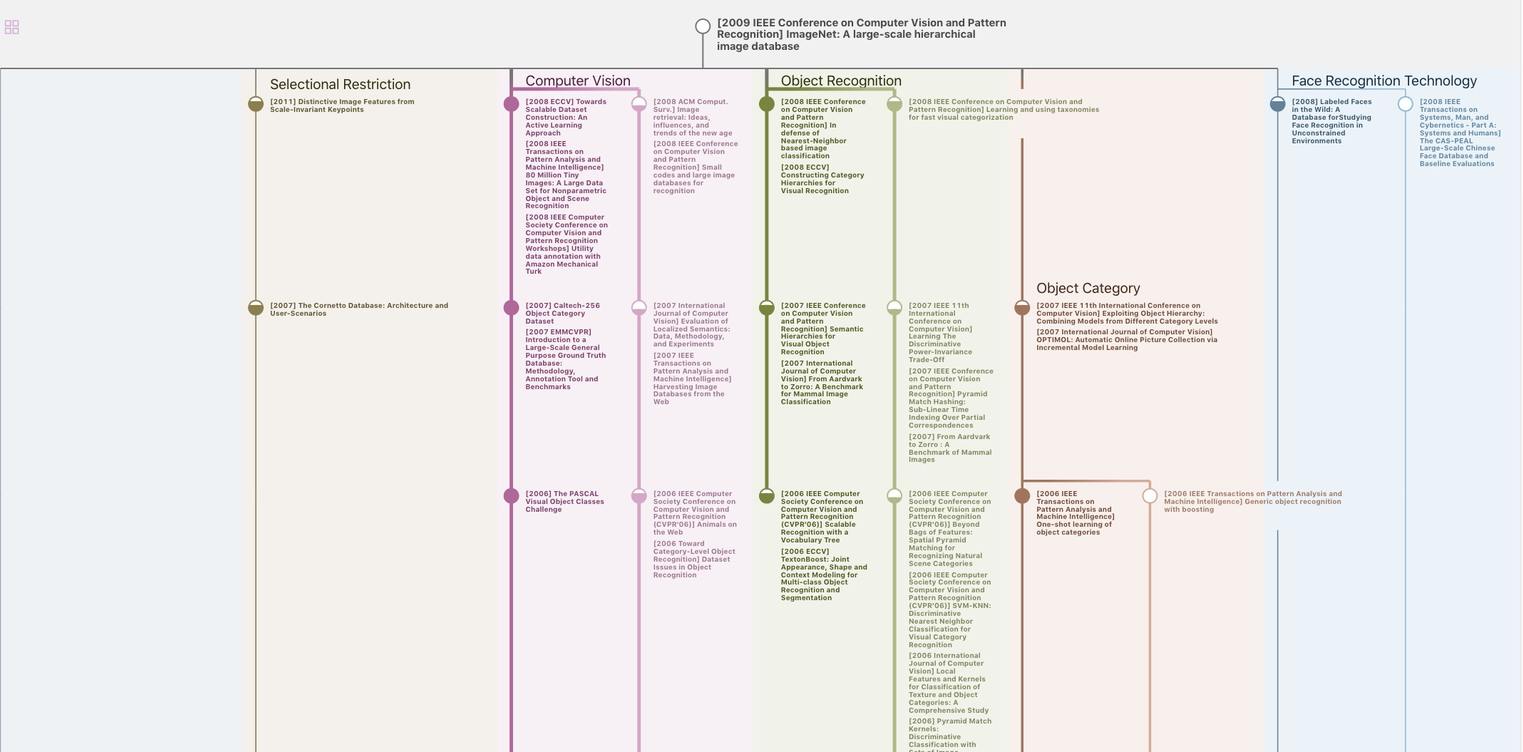
生成溯源树,研究论文发展脉络
Chat Paper
正在生成论文摘要