EAPR: explainable and augmented patient representation learning for disease prediction
Health Information Science and Systems(2023)
摘要
Patient representation learning aims to encode meaningful information about the patient’s Electronic Health Records (EHR) in the form of a mathematical representation. Recent advances in deep learning have empowered Patient representation learning methods with greater representational power, allowing the learned representations to significantly improve the performance of disease prediction models. However, the inherent shortcomings of deep learning models, such as the need for massive amounts of labeled data and inexplicability, limit the performance of deep learning-based Patient representation learning methods to further improvements. In particular, learning robust patient representations is challenging when patient data is missing or insufficient. Although data augmentation techniques can tackle this deficiency, the complex data processing further weakens the inexplicability of patient representation learning models. To address the above challenges, this paper proposes an Explainable and Augmented Patient Representation Learning for disease prediction (EAPR). EAPR utilizes data augmentation controlled by confidence interval to enhance patient representation in the presence of limited patient data. Moreover, EAPR proposes to use two-stage gradient backpropagation to address the problem of unexplainable patient representation learning models due to the complex data enhancement process. The experimental results on real clinical data validate the effectiveness and explainability of the proposed approach.
更多查看译文
关键词
Patient representation,Data augmentation,Disease prediction,Explanation method
AI 理解论文
溯源树
样例
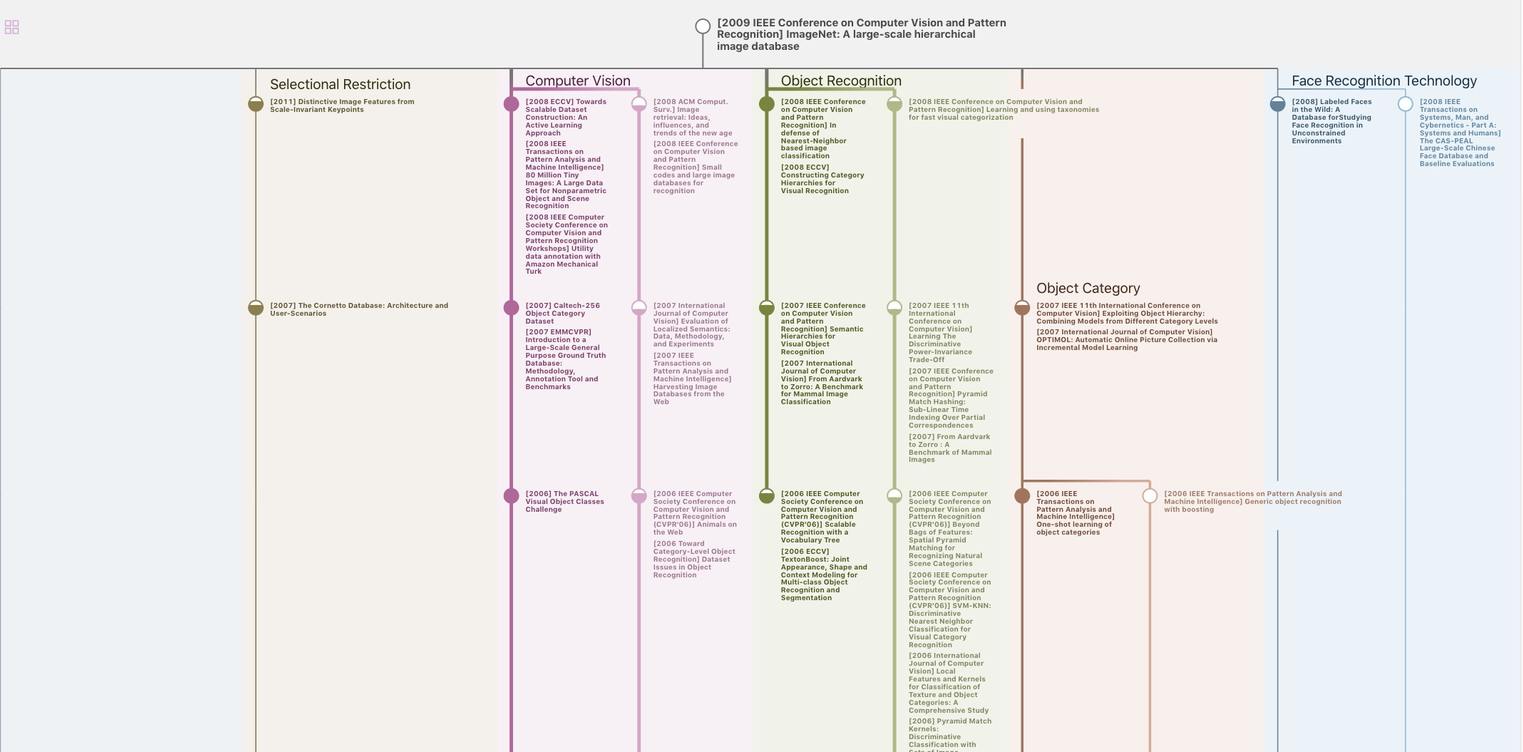
生成溯源树,研究论文发展脉络
Chat Paper
正在生成论文摘要