Deep Learning Aided Minimum Mean Square Error Estimation of Gaussian Source in Industrial Internet-of-Things Networks
IEEE Transactions on Industrial Cyber-Physical Systems(2024)
摘要
This paper investigates the problem of estimating complex-valued Gaussian signals in an industrial Internet of Things (IIoT) environment, where the channel fading is temporally correlated and modeled by a finite state Markov process. To address the non-trivial problem of estimating channel fading states and signals simultaneously, we propose two deep learning (DL)-aided minimum mean square error (MMSE) estimation schemes. More specifically, our proposed framework consists of two steps, (i) a DL-aided channel fading state estimation and prediction step, followed by (ii) a linear MMSE estimation step to estimate the source signals for the learned channel fading states. Our proposed framework employs three DL models, namely the fully connected deep neural network (DNN), long short-term memory (LSTM) integrated DNN, and temporal convolution network (TCN). Extensive simulations show that these three DL models achieve similar accuracy in predicting the states of wireless fading channels. Our proposed data-driven approaches exhibit a reasonable performance gap in normalized mean square error (NMSE) compared to the genie-aided scheme, which considers perfect knowledge of instantaneous channel fading states.
更多查看译文
关键词
Channel fading,deep learning,IIoT,industry 5.0,MMSE,markov process
AI 理解论文
溯源树
样例
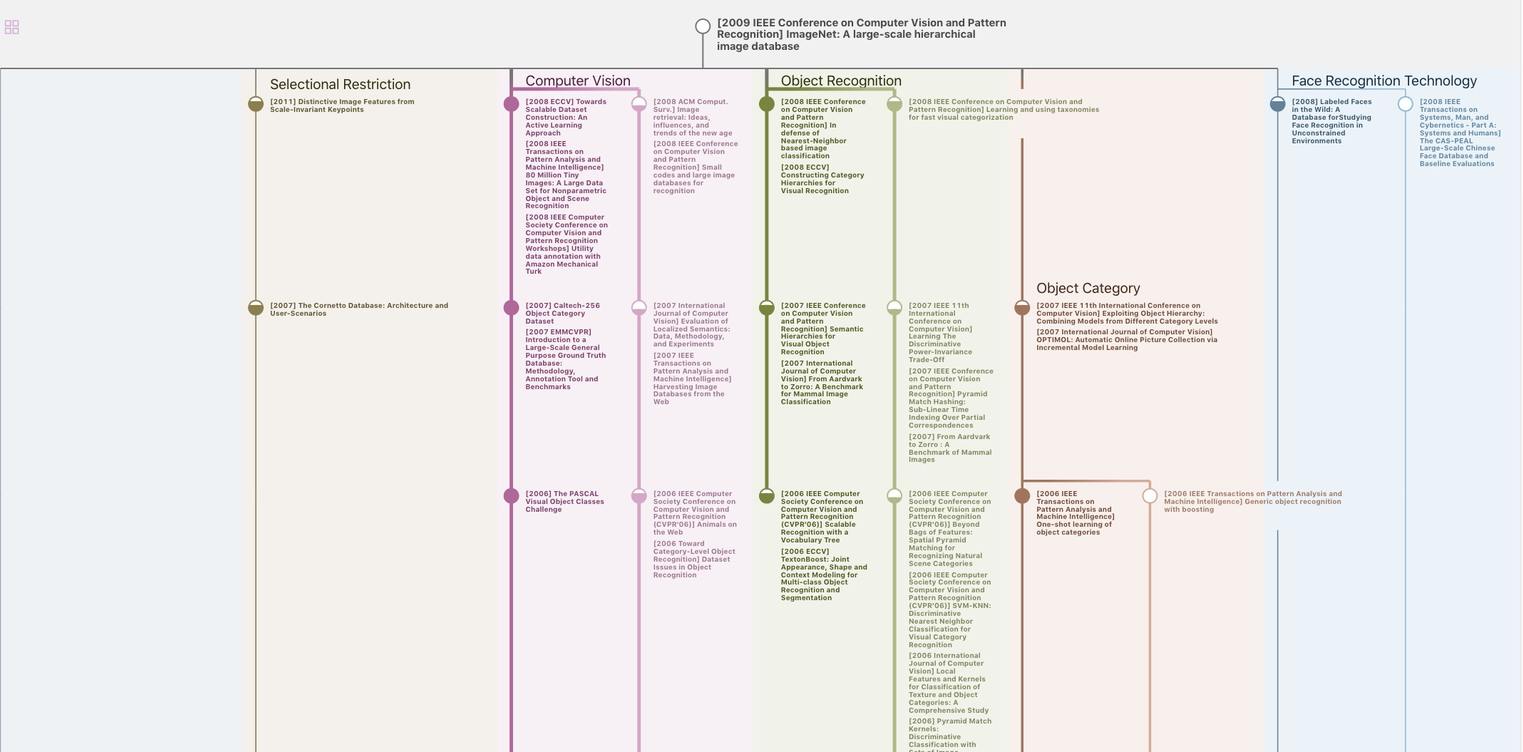
生成溯源树,研究论文发展脉络
Chat Paper
正在生成论文摘要