AttentionMGT-DTA: A multi-modal drug-target affinity prediction using graph transformer and attention mechanism
Neural Networks(2024)
摘要
The accurate prediction of drug-target affinity (DTA) is a crucial step in drug discovery and design. Traditional experiments are very expensive and time-consuming. Recently, deep learning methods have achieved notable performance improvements in DTA prediction. However, one challenge for deep learning-based models is appropriate and accurate representations of drugs and targets, especially the lack of effective exploration of target representations. Another challenge is how to comprehensively capture the interaction information between different instances, which is also important for predicting DTA. In this study, we propose AttentionMGT-DTA, a multi-modal attention-based model for DTA prediction. AttentionMGT-DTA represents drugs and targets by a molecular graph and binding pocket graph, respectively. Two attention mechanisms are adopted to integrate and interact information between different protein modalities and drug-target pairs. The experimental results showed that our proposed model outperformed state-of-the-art baselines on two benchmark datasets. In addition, AttentionMGT-DTA also had high interpretability by modeling the interaction strength between drug atoms and protein residues. Our code is available at https://github.com/JK-Liu7/AttentionMGT-DTA.
更多查看译文
关键词
Drug–target affinity,Graph neural network,Graph transformer,Attention mechanism,Multi-modal learning
AI 理解论文
溯源树
样例
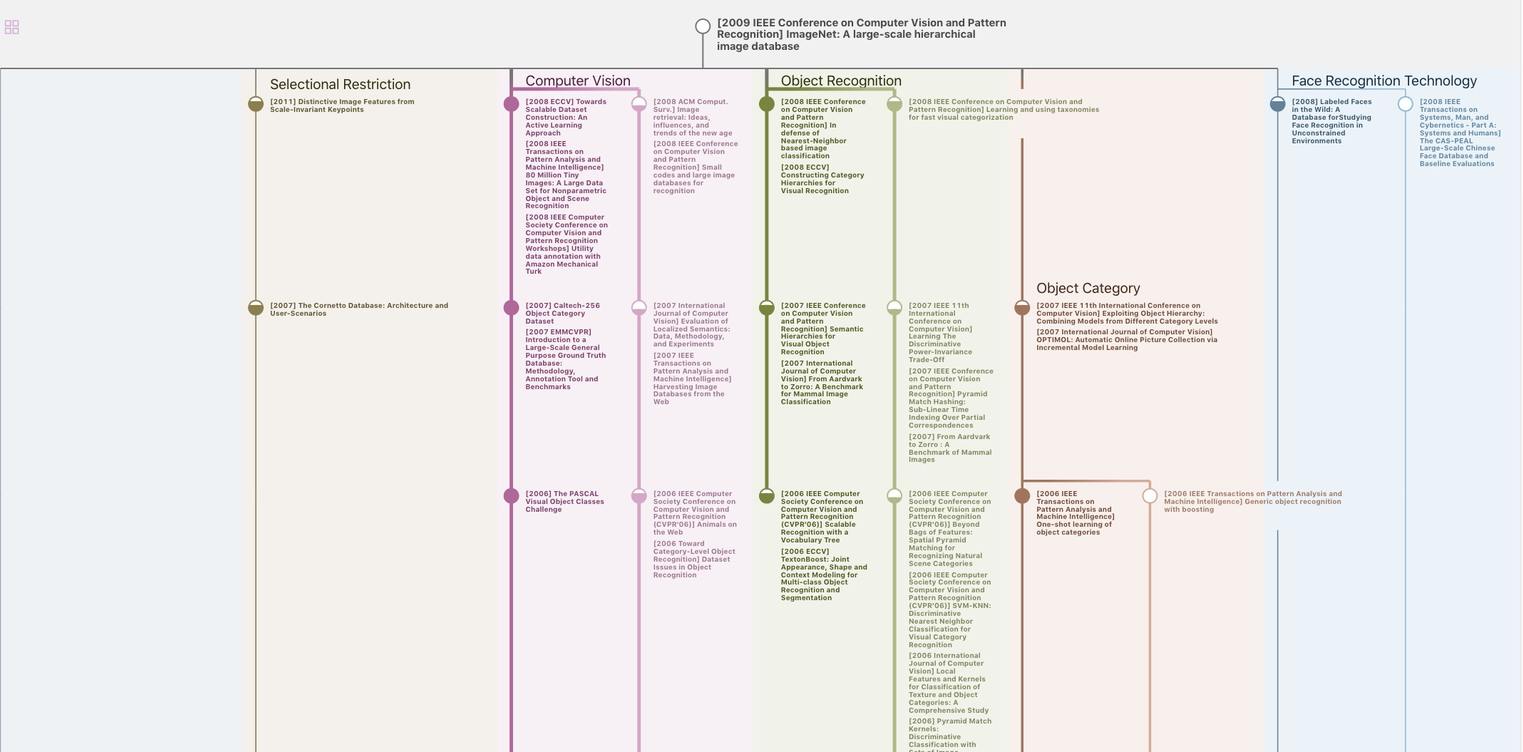
生成溯源树,研究论文发展脉络
Chat Paper
正在生成论文摘要