Federated Knowledge Graph Completion via Latent Embedding Sharing and Tensor Factorization
23RD IEEE INTERNATIONAL CONFERENCE ON DATA MINING, ICDM 2023(2023)
摘要
Knowledge graphs (KGs), which consist of triples, are inherently incomplete and always require completion procedure to predict missing triples. In real-world scenarios, KGs are distributed across clients, complicating completion tasks due to privacy restrictions. Many frameworks have been proposed to address the issue of federated knowledge graph completion. However, the existing frameworks, including FedE, FedR, and FEKG, have certain limitations. = FedE poses a risk of information leakage, FedR's optimization efficacy diminishes when there is minimal overlap among relations, and FKGE suffers from computational costs and mode collapse issues. To address these issues, we propose a novel method, i.e., Federated Latent Embedding Sharing Tensor factorization (FLEST), which is a novel approach using federated tensor factorization for KG completion. FLEST decompose the embedding matrix and enables sharing of latent dictionary embeddings to lower privacy risks. Empirical results demonstrate FLEST's effectiveness and efficiency, offering a balanced solution between performance and privacy. FLEST expands the application of federated tensor factorization in KG completion tasks.
更多查看译文
关键词
Knowledge Graph Completion,Federated Graph Learning,Federated Tensor Decomposition
AI 理解论文
溯源树
样例
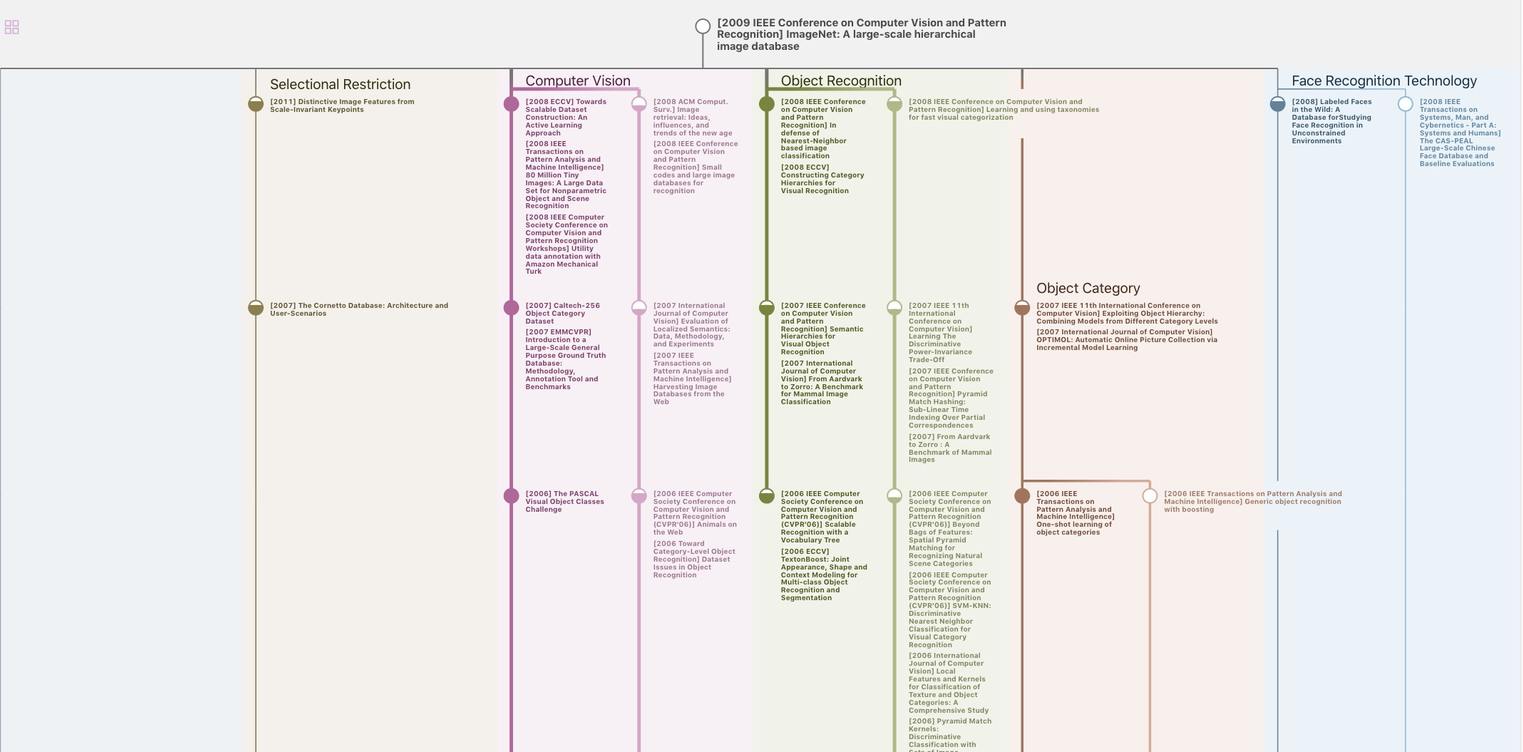
生成溯源树,研究论文发展脉络
Chat Paper
正在生成论文摘要