Unsupervised Dialogue State Tracking for End-to-End Task-Oriented Dialogue with a Multi-Span Prediction Network
Journal of Computer Science and Technology(2023)
摘要
This paper focuses on end-to-end task-oriented dialogue systems, which jointly handle dialogue state tracking (DST) and response generation. Traditional methods usually adopt a supervised paradigm to learn DST from a manually labeled corpus. However, the annotation of the corpus is costly, time-consuming, and cannot cover a wide range of domains in the real world. To solve this problem, we propose a multi-span prediction network (MSPN) that performs unsupervised DST for end-to-end task-oriented dialogue. Specifically, MSPN contains a novel split-merge copy mechanism that captures long-term dependencies in dialogues to automatically extract multiple text spans as keywords. Based on these keywords, MSPN uses a semantic distance based clustering approach to obtain the values of each slot. In addition, we propose an ontology-based reinforcement learning approach, which employs the values of each slot to train MSPN to generate relevant values. Experimental results on single-domain and multi-domain task-oriented dialogue datasets show that MSPN achieves state-of-the-art performance with significant improvements. Besides, we construct a new Chinese dialogue dataset MeDial in the low-resource medical domain, which further demonstrates the adaptability of MSPN.
更多查看译文
关键词
dialogue,tracking,end-to-end,task-oriented,multi-span
AI 理解论文
溯源树
样例
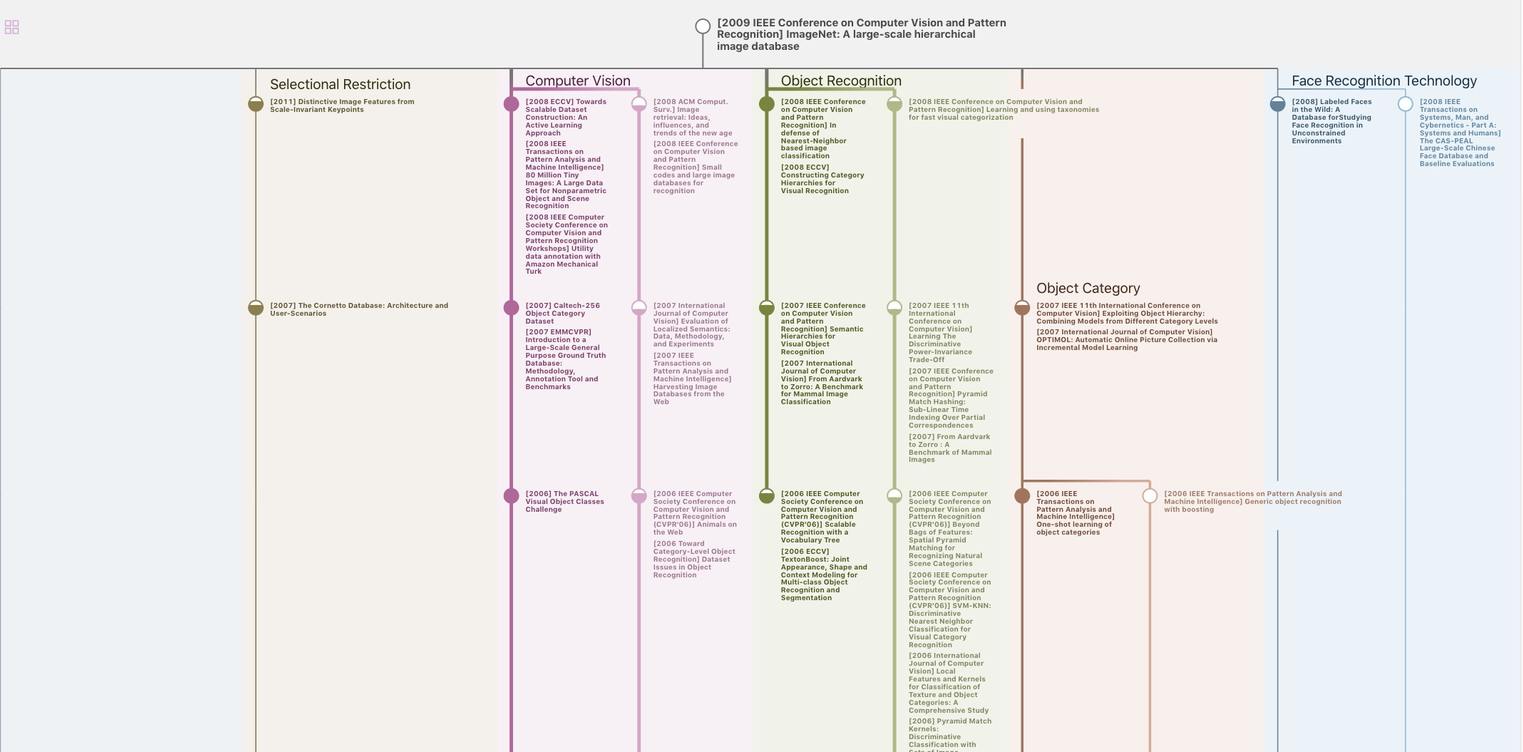
生成溯源树,研究论文发展脉络
Chat Paper
正在生成论文摘要