DyPipe: A Holistic Approach to Accelerating Dynamic Neural Networks with Dynamic Pipelining
Journal of Computer Science and Technology(2023)
摘要
Dynamic neural network (NN) techniques are increasingly important because they facilitate deep learning techniques with more complex network architectures. However, existing studies, which predominantly optimize the static computational graphs by static scheduling methods, usually focus on optimizing static neural networks in deep neural network (DNN) accelerators. We analyze the execution process of dynamic neural networks and observe that dynamic features introduce challenges for efficient scheduling and pipelining in existing DNN accelerators. We propose DyPipe, a holistic approach to optimizing dynamic neural network inferences in enhanced DNN accelerators. DyPipe achieves significant performance improvements for dynamic neural networks while it introduces negligible overhead for static neural networks. Our evaluation demonstrates that DyPipe achieves 1.7x speedup on dynamic neural networks and maintains more than 96% performance for static neural networks.
更多查看译文
关键词
dynamic neural network (NN),deep neural network (DNN) accelerator,dynamic pipelining
AI 理解论文
溯源树
样例
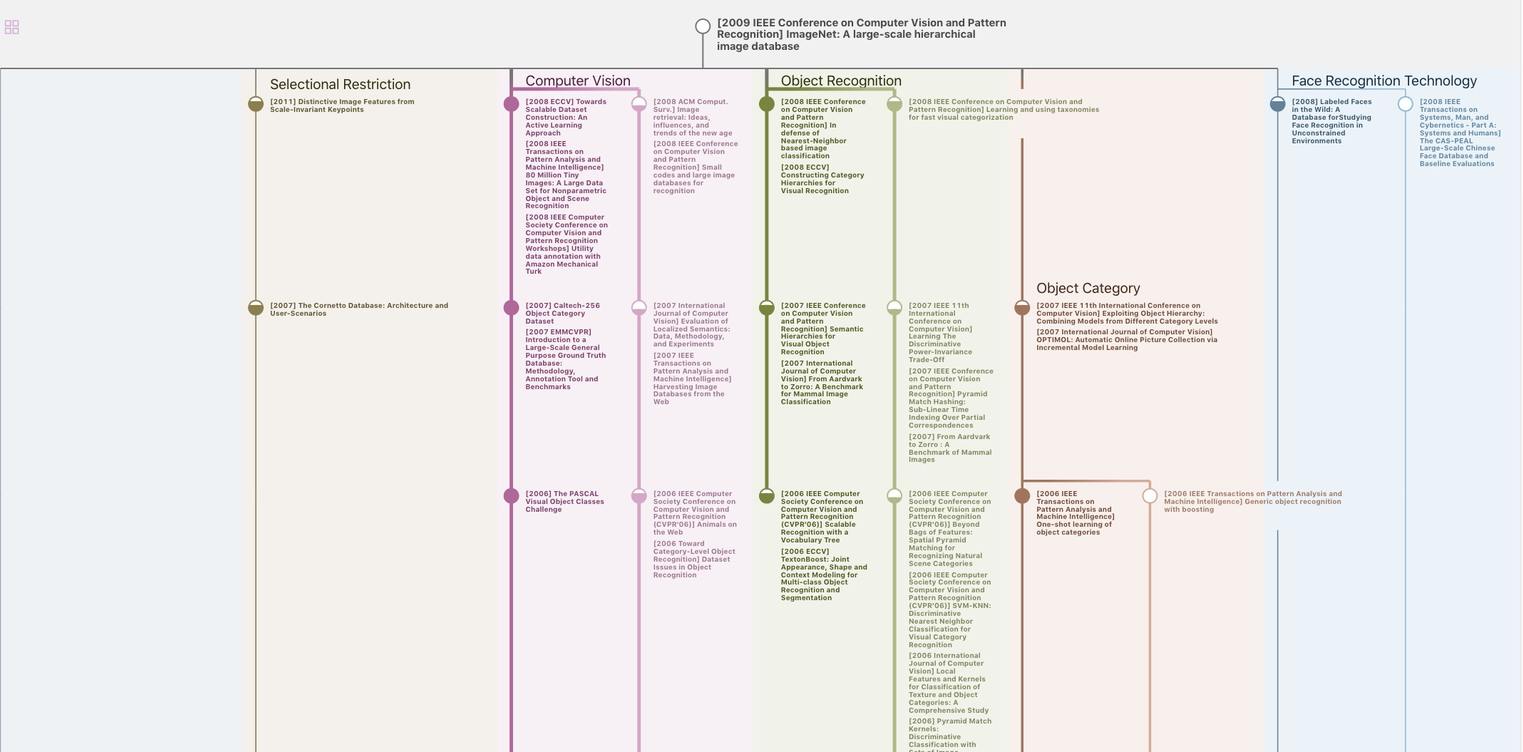
生成溯源树,研究论文发展脉络
Chat Paper
正在生成论文摘要