Improving Diagnostic Sensitivity for Imbalanced Musculoskeletal Disorder Data: A Sensitivity-Based Multi-Sampling Technique for Osteoarthritis Prediction
medrxiv(2023)
摘要
Background: Medical datasets containing musculoskeletal disorders may have data imbalances due to the incidence of the disease, which may limit the predictive ability, such as the sensitivity, of musculoskeletal diagnostic prediction models built from these data. This study aimed to increase the sensitivity performance of osteoarthritis (OA) prediction when building a model by adjusting an OA imbalanced dataset using a sensitivity-based multi-sampling (SMS) technique. Methods: OA Data were obtained from the Korea National Health and Nutrition Examination Survey (KNHANES). SMS technique combining oversampling and undersampling was applied to the imbalanced OA data, and the RandomForest algorithm was used for machine learning modeling. Model performance was evaluated based on accuracy, sensitivity, and specificity and compared with other hybrid sampling techniques. Result: In the SMS technique, ADASYN, Borderline-SMOTE, SMOTE oversampling and ENN undersampling techniques were combined and applied. The OA prediction model using the SMS technique showed the highest sensitivity (82.20) but the lowest specificity (82.26) and accuracy (82.26) compared to other hybrid models. Conclusion: SMS technology offers a potential solution for improving sensitivity performance for prediction models built on medical data imbalances due to low-incidence diseases. Nonetheless, caution is warranted due to the concern that while improving sensitivity, it may decrease specificity with a trade-off.
### Competing Interest Statement
The authors have declared no competing interest.
### Funding Statement
This study did not receive any funding
### Author Declarations
I confirm all relevant ethical guidelines have been followed, and any necessary IRB and/or ethics committee approvals have been obtained.
Yes
The details of the IRB/oversight body that provided approval or exemption for the research described are given below:
This study was approved by the Public Institutional Review Board Designated by Ministry of Health and Welfare (approval number: P01-202311-01-031)
I confirm that all necessary patient/participant consent has been obtained and the appropriate institutional forms have been archived, and that any patient/participant/sample identifiers included were not known to anyone (e.g., hospital staff, patients or participants themselves) outside the research group so cannot be used to identify individuals.
Yes
I understand that all clinical trials and any other prospective interventional studies must be registered with an ICMJE-approved registry, such as ClinicalTrials.gov. I confirm that any such study reported in the manuscript has been registered and the trial registration ID is provided (note: if posting a prospective study registered retrospectively, please provide a statement in the trial ID field explaining why the study was not registered in advance).
Yes
I have followed all appropriate research reporting guidelines, such as any relevant EQUATOR Network research reporting checklist(s) and other pertinent material, if applicable.
Yes
This study used data samples obtained from the 8th Korean National Health and Nutrition Survey (KNHANES) of the Korea Centers for Disease Control and Prevention from 2019 to 2020.
更多查看译文
AI 理解论文
溯源树
样例
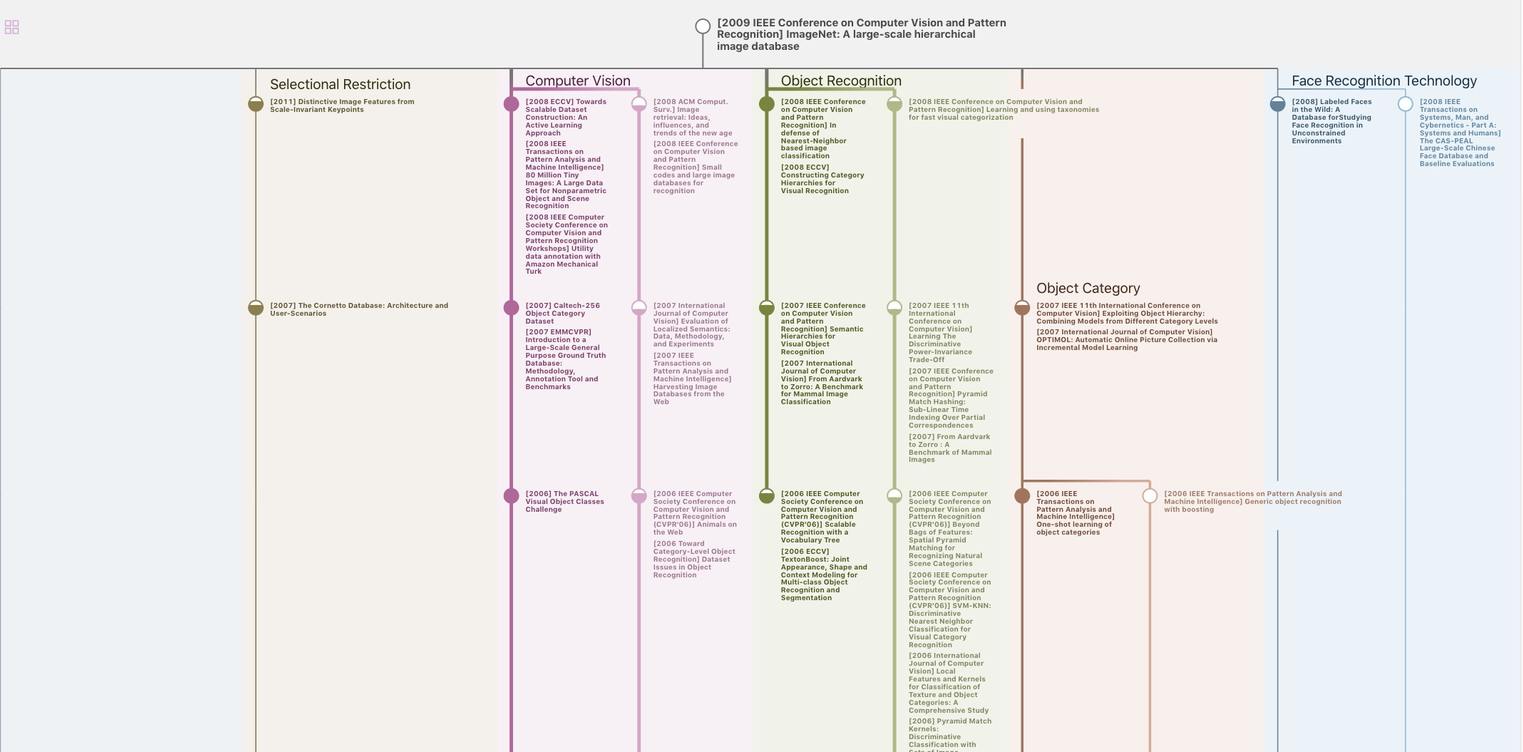
生成溯源树,研究论文发展脉络
Chat Paper
正在生成论文摘要