Text-to-Sticker: Style Tailoring Latent Diffusion Models for Human Expression.
CoRR(2023)
摘要
We introduce Style Tailoring, a recipe to finetune Latent Diffusion Models
(LDMs) in a distinct domain with high visual quality, prompt alignment and
scene diversity. We choose sticker image generation as the target domain, as
the images significantly differ from photorealistic samples typically generated
by large-scale LDMs. We start with a competent text-to-image model, like Emu,
and show that relying on prompt engineering with a photorealistic model to
generate stickers leads to poor prompt alignment and scene diversity. To
overcome these drawbacks, we first finetune Emu on millions of sticker-like
images collected using weak supervision to elicit diversity. Next, we curate
human-in-the-loop (HITL) Alignment and Style datasets from model generations,
and finetune to improve prompt alignment and style alignment respectively.
Sequential finetuning on these datasets poses a tradeoff between better style
alignment and prompt alignment gains. To address this tradeoff, we propose a
novel fine-tuning method called Style Tailoring, which jointly fits the content
and style distribution and achieves best tradeoff. Evaluation results show our
method improves visual quality by 14%, prompt alignment by 16.2% and scene
diversity by 15.3%, compared to prompt engineering the base Emu model for
stickers generation.
更多查看译文
AI 理解论文
溯源树
样例
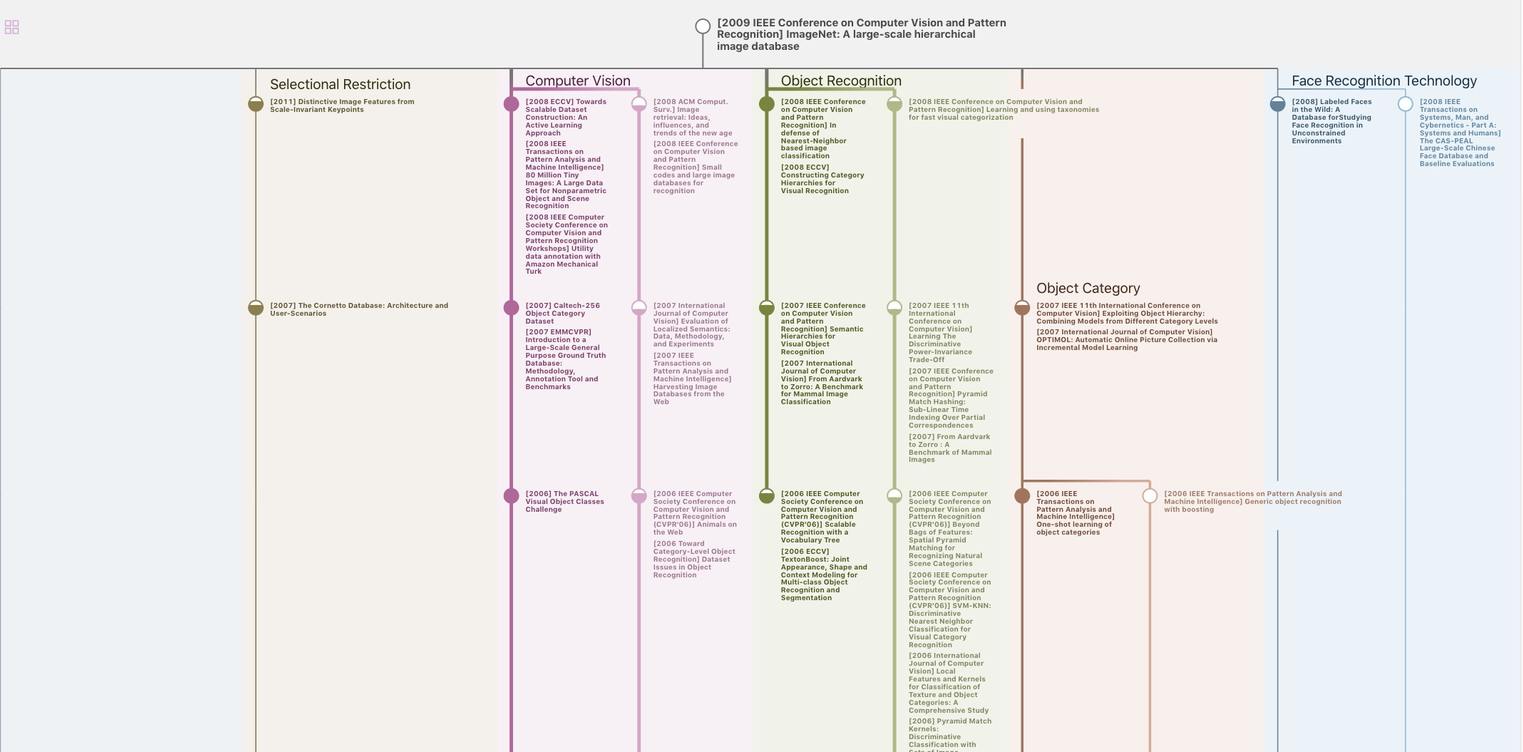
生成溯源树,研究论文发展脉络
Chat Paper
正在生成论文摘要