Towards Complex-Valued Deep Architectures with Data Model Preservation for Sea Surface Current Estimation
20TH INTERNATIONAL CONFERENCE ON CONTENT-BASED MULTIMEDIA INDEXING, CBMI 2023(2023)
摘要
The application of deep learning methods in various fields is rapidly increasing. The development of complex-valued (CV) networks that can process CV data has provided many opportunities for utilizing the immense capabilities of deep networks for CV data, including Synthetic Aperture Radar (SAR). However, the physical model and basic properties of the original SAR data must be preserved in the CV architecture. Without these properties, the physical parameters cannot be accurately retrieved from the SAR data. This study evaluates the competency of CV deep architectures to preserve the properties of the original SAR data and how it affects the retrieval of physical parameters. Ocean Surface Current (OSC) is an important parameter for ocean circulation and plays a vital role globally. In this work, the correlation Doppler estimation (CDE) method is used to estimate the OSC from SAR data before and after reconstruction with the CV autoencoder. The obtained OSCs are compared, and we demonstrate the ability of the CV deep architectures to learn the data model and preserve the original Doppler centroid (f(DC)) information in the SAR data. This research paves the way for the development of CV deep architectures for physical parameter retrieval and prediction from CV SAR data in future studies.
更多查看译文
关键词
Complex-Valued Deep Networks,Doppler Centroid,Ocean Surface Current (OSC),Synthetic Aperture Radar (SAR)
AI 理解论文
溯源树
样例
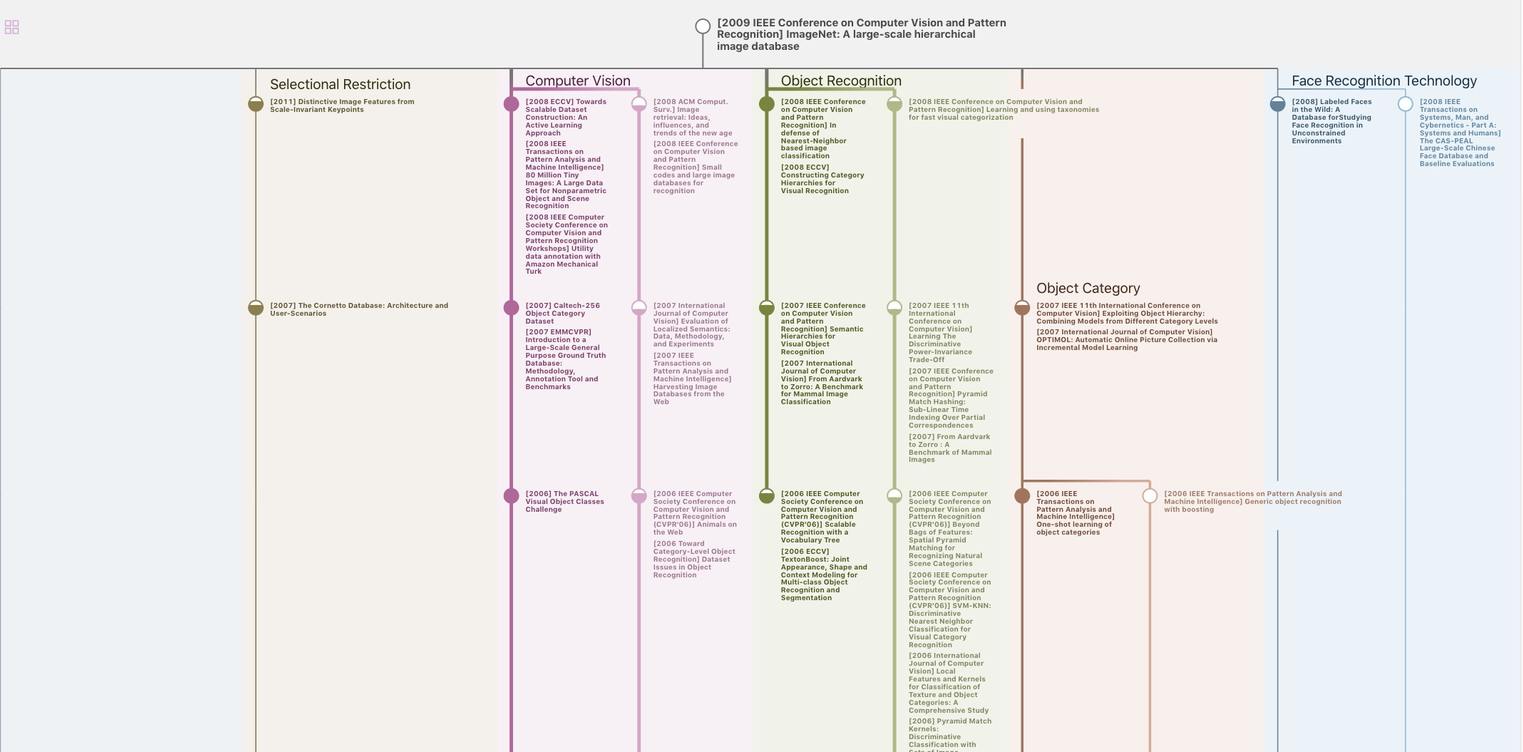
生成溯源树,研究论文发展脉络
Chat Paper
正在生成论文摘要