Hourglass Tokenizer for Efficient Transformer-Based 3D Human Pose Estimation
arXiv (Cornell University)(2023)
摘要
Transformers have been successfully applied in the field of video-based 3Dhuman pose estimation. However, the high computational costs of these videopose transformers (VPTs) make them impractical on resource-constrained devices.In this paper, we present a plug-and-play pruning-and-recovering framework,called Hourglass Tokenizer (HoT), for efficient transformer-based 3D human poseestimation from videos. Our HoT begins with pruning pose tokens of redundantframes and ends with recovering full-length tokens, resulting in a few posetokens in the intermediate transformer blocks and thus improving the modelefficiency. To effectively achieve this, we propose a token pruning cluster(TPC) that dynamically selects a few representative tokens with high semanticdiversity while eliminating the redundancy of video frames. In addition, wedevelop a token recovering attention (TRA) to restore the detailedspatio-temporal information based on the selected tokens, thereby expanding thenetwork output to the original full-length temporal resolution for fastinference. Extensive experiments on two benchmark datasets (i.e., Human3.6M andMPI-INF-3DHP) demonstrate that our method can achieve both high efficiency andestimation accuracy compared to the original VPT models. For instance, applyingto MotionBERT and MixSTE on Human3.6M, our HoT can save nearly 50without sacrificing accuracy and nearly 40respectively. Code and models are available athttps://github.com/NationalGAILab/HoT.
更多查看译文
关键词
3D Human Pose,Pose Estimation,Gesture Recognition,Multiple Object Tracking,Action Recognition
AI 理解论文
溯源树
样例
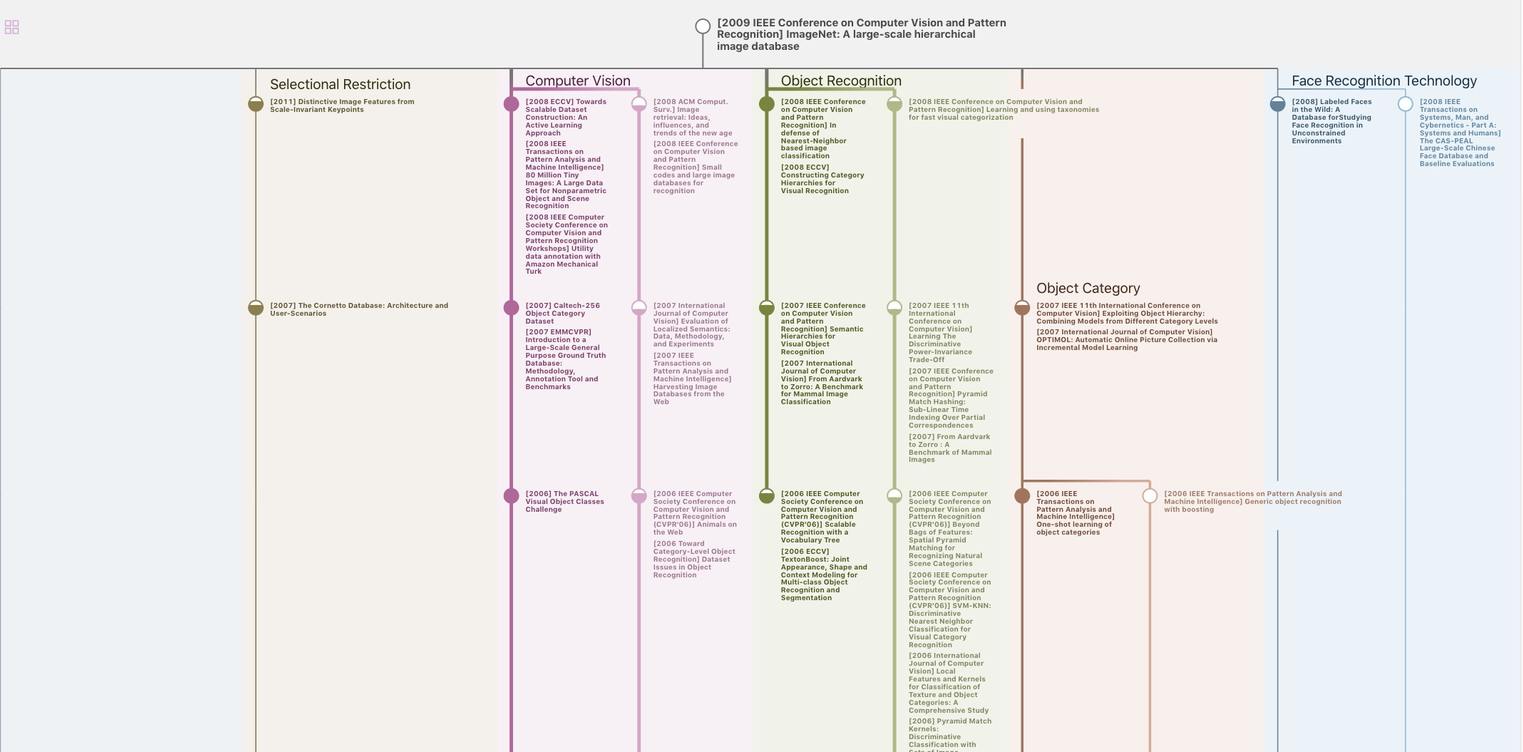
生成溯源树,研究论文发展脉络
Chat Paper
正在生成论文摘要