Low-dimensional controllability of brain networks
arxiv(2023)
摘要
Network controllability is a powerful tool to study causal relationships in complex systems and identify the driver nodes for steering the network dynamics into desired states. However, due to ill-posed conditions, results become unreliable when the number of drivers becomes too small compared to the network size. This is a very common situation, particularly in real-world applications, where the possibility to access multiple nodes at the same time is limited by technological constraints, such as in the human brain. Although targeting smaller network parts might improve accuracy, challenges may remain for extremely unbalanced situations, when for example there is one single driver. To address this problem, we developed a mathematical framework that combines concepts from spectral graph theory and modern network science. Instead of controlling the original network dynamics, we aimed to control its low-dimensional embedding into the topological space derived from the network Laplacian. By performing extensive simulations on synthetic networks, we showed that a relatively low number of projected components is enough to improve the overall control accuracy, notably when dealing with very few drivers. Based on these findings, we introduced alternative low-dimensional controllability metrics and used them to identify the main driver areas of the human connectome obtained from N=6134 healthy individuals in the UK-biobank cohort. Results revealed previously unappreciated influential regions compared to standard approaches, enabled to draw control maps between distinct specialized large-scale brain systems, and yielded an anatomically-based understanding of hemispheric functional lateralization. Taken together, our results offered a theoretically-grounded solution to deal with network controllability in real-life applications and provided insights into the causal interactions of the human brain.
更多查看译文
AI 理解论文
溯源树
样例
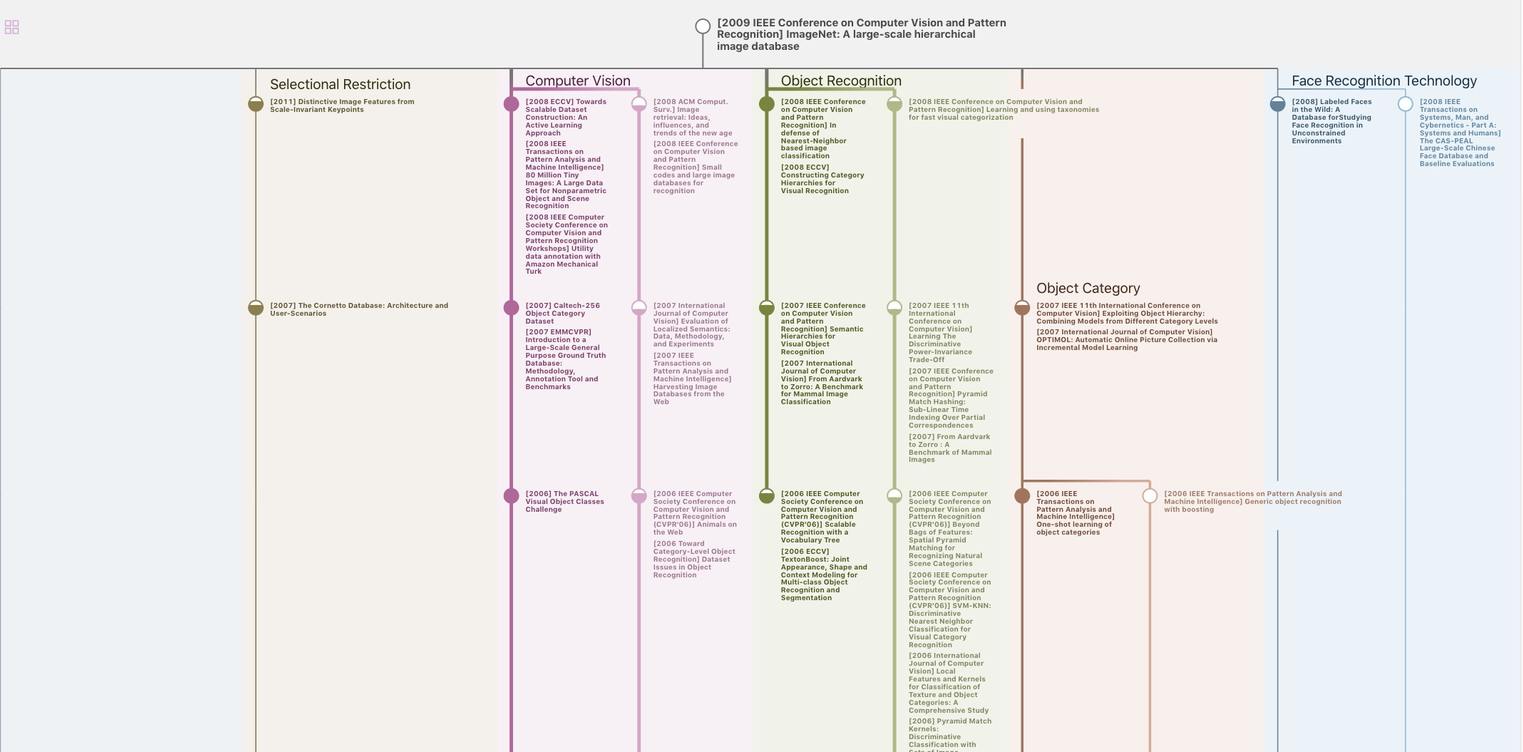
生成溯源树,研究论文发展脉络
Chat Paper
正在生成论文摘要