Integration of text-mining and telemedicine appointment optimization
Annals of Operations Research(2023)
摘要
Nowadays, many countries view profitable telemedicine as a viable strategy for meeting healthcare needs, especially during the pandemic. Existing appointment models are based on patients’ structured data. We study the value of incorporating textual patient data into telemedicine appointment optimization. Our research contributes to the healthcare operations management literature by developing a new framework showing (1) the value of the text in the telemedicine appointment problem, (2) the value of incorporating the textual and structured data in the problem. In particular, in the first phase of the framework, a text-driven classification model is developed to classify patients into normal and prolonged service time classes. In the second phase, we integrate the classification model into two existing decision-making policies. We analyze the performance of our proposed policy in the presence of existing methods on a data set from the National Telemedicine Center of China (NTCC). We first show that our classifier can achieve 90.4% AUC in a binary task based on textual data. We next show that our method outperforms the stochastic model available in the literature. In particular, with a slight change of actual distribution from historical data to a normal distribution, we observe that our policy improves the average profit of the policy obtained from the stochastic model by 42% and obtains lower relative regret (18%) from full information than the stochastic model (148%). Furthermore, our policy provides a promising trade-off between the cancellation and postponement rates of patients, resulting in a higher profit and a better schedule strategy for the telemedicine center.
更多查看译文
关键词
Telemedicine appointment scheduling,Machine learning,Text mining,Stochastic programming
AI 理解论文
溯源树
样例
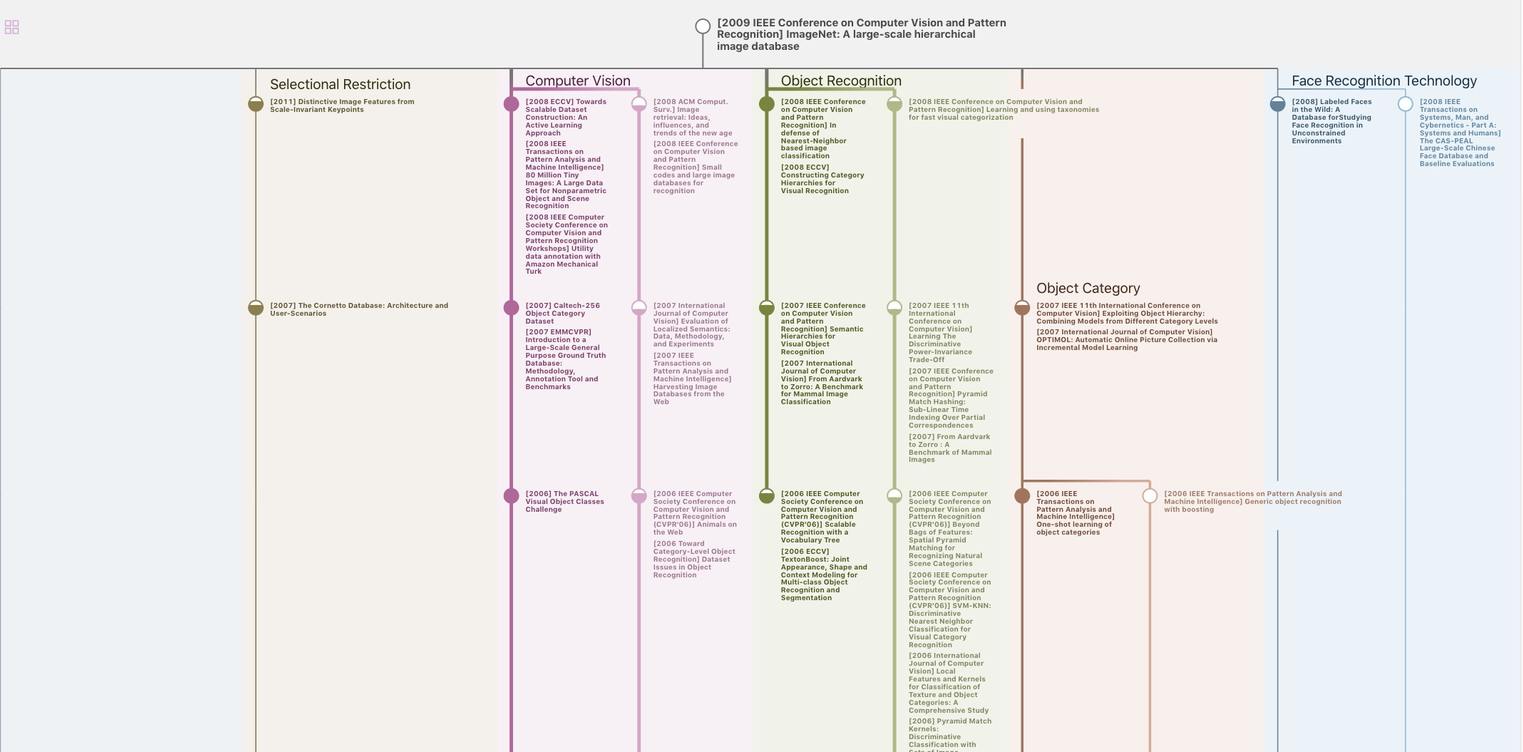
生成溯源树,研究论文发展脉络
Chat Paper
正在生成论文摘要