Unknown fault detection method for rolling bearings based on image and signal series feature fusion enhancement
Multimedia Tools and Applications(2023)
摘要
This paper mainly studies the problem of finding new fault classes under different modes in the field of intelligent fault diagnosis, that is, in the case of some labeled faults, new classes are revealed in unlabeled fault samples. In this paper, we introduce a comprehensive multi-modal framework for novel fault discovery and explore the impact of different modalities on the task of identifying new fault classes. To enhance the robustness of feature representation in complex environments, We adopted the approach inspired by ChatGPT, wherein we conducted pre-training on a substantial amount of labeled data to learn general features, patterns, and representations of various fault types. During the pre-training process, we integrated multiple modalities of data to prevent the loss of information due to the absence of single-modal data, thereby enhancing the accuracy of clustering. Furthermore, we introduced a multi-modal fusion method based on saliency correlation to complementarily fuse the information from different modalities. This approach effectively eliminated data redundancy arising from diverse modalities. Adhering to the principle that improving the quality of pseudo-label generation during the new class discovery phase enhances the accuracy of clustering for new classes, we extend the multi-modal concept. We introduce a Discriminative Relationship Enhancement method that capitalizes on cross-validation of pseudo-label predictions from different modalities during the pseudo-label prediction phase. This augmentation enhances the precision of pseudo-labels during the new class discovery phase. We evaluated the effectiveness of our proposed framework on fault datasets CWRU and PU, achieving promising results.
更多查看译文
关键词
Novel class discovery,Intelligent fault diagnosis,Multimodal,Convolutional neural network
AI 理解论文
溯源树
样例
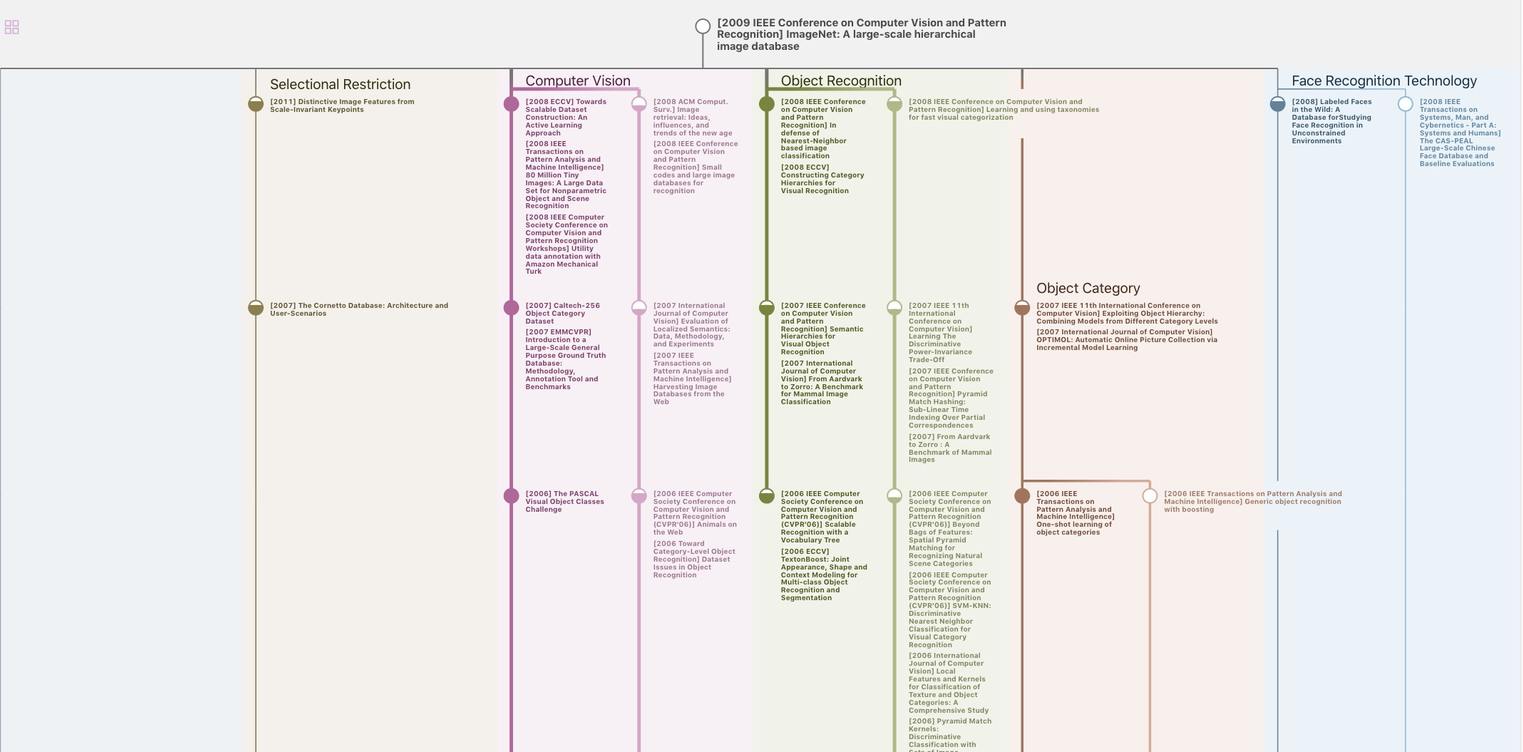
生成溯源树,研究论文发展脉络
Chat Paper
正在生成论文摘要