Eight pruning deep learning models for low storage and high-speed COVID-19 computed tomography lung segmentation and heatmap-based lesion localization
Computers in Biology and Medicine(2022)
摘要
COVLIAS 1.0: an automated lung segmentation was designed for COVID-19 diagnosis. It has issues related to storage space and speed. This study shows that COVLIAS 2.0 uses pruned AI (PAI) networks for improving both storage and speed, wiliest high performance on lung segmentation and lesion localization. ology : The proposed study uses multicenter ∼9,000 CT slices from two different nations, namely, CroMed from Croatia (80 patients, experimental data), and NovMed from Italy (72 patients, validation data). We hypothesize that by using pruning and evolutionary optimization algorithms, the size of the AI models can be reduced significantly, ensuring optimal performance. Eight different pruning techniques (i) differential evolution (DE), (ii) genetic algorithm (GA), (iii) particle swarm optimization algorithm (PSO), and (iv) whale optimization algorithm (WO) in two deep learning frameworks (i) Fully connected network (FCN) and (ii) SegNet were designed. COVLIAS 2.0 was validated using “Unseen NovMed” and benchmarked against MedSeg. Statistical tests for stability and reliability were also conducted. Pruning algorithms (i) FCN-DE, (ii) FCN-GA, (iii) FCN–PSO, and (iv) FCN-WO showed improvement in storage by 92.4 %, 95.3 %, 98.7 %, and 99.8 % respectively when compared against solo FCN, and (v) SegNet-DE, (vi) SegNet-GA, (vii) SegNet-PSO, and (viii) SegNet-WO showed improvement by 97.1 %, 97.9 %, 98.8 %, and 99.2 % respectively when compared against solo SegNet. AUC > 0.94 (p < 0.0001) on CroMed and > 0.86 (p < 0.0001) on NovMed data set for all eight EA model. PAI <0.25 s per image. DenseNet-121-based Grad-CAM heatmaps showed validation on glass ground opacity lesions. Eight PAI networks that were successfully validated are five times faster, storage efficient, and could be used in clinical settings. • Eight evolutional algorithms (EA) based on Deep Learning for reduced storage and high speed. • Multicenter study with 9,000 CT slices. • COVID-19 based CT lung segmentation and lesion localization. • Benchmarking against the EA against MedSeg (web-based tool). • Statistical analysis for reliability and stability, including comprehensive data analysis.
更多查看译文
关键词
COVID-19,Lung CT,Hounsfield units,Glass ground opacities,AI,Deep learning,Pruning,Lung segmentation
AI 理解论文
溯源树
样例
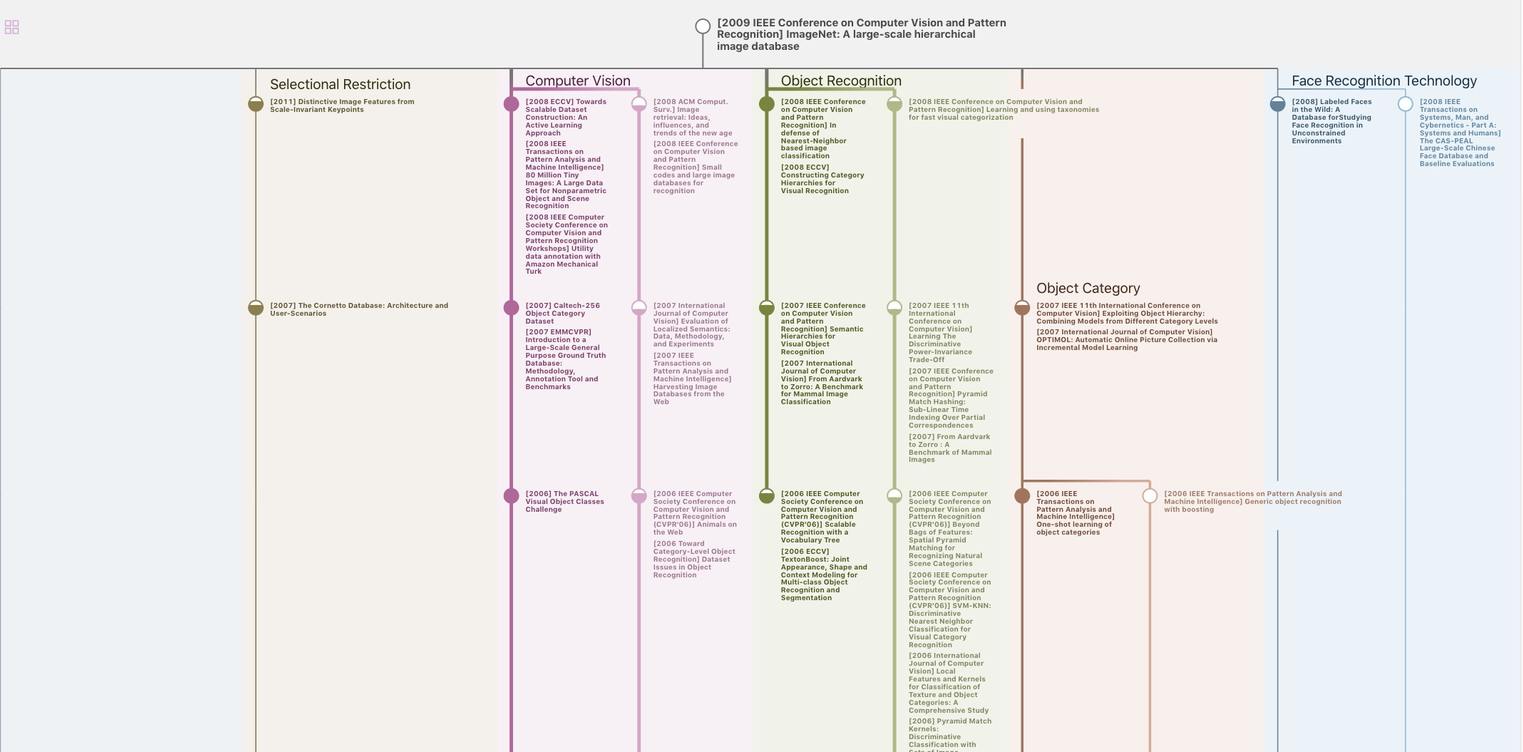
生成溯源树,研究论文发展脉络
Chat Paper
正在生成论文摘要