Dcam-net
Computers in Biology and Medicine(2023)
摘要
Fundus images are an essential basis for diagnosing ocular diseases, and using convolutional neural networks has shown promising results in achieving accurate fundus image segmentation. However, the difference between the training data (source domain) and the testing data (target domain) will significantly affect the final segmentation performance. This paper proposes a novel framework named DCAM-NET for fundus domain generalization segmentation, which substantially improves the generalization ability of the segmentation model to the target domain data and enhances the extraction of detailed information on the source domain data. This model can effectively overcome the problem of poor model performance due to cross-domain segmentation. To enhance the adaptability of the segmentation model to target domain data, this paper proposes a multi-scale attention mechanism module (MSA) that functions at the feature extraction level. Extracting different attribute features to enter the corresponding scale attention module further captures the critical features in channel, position, and spatial regions. The MSA attention mechanism module also integrates the characteristics of the self-attention mechanism, it can capture dense context information, and the aggregation of multi-feature information effectively enhances the generalization of the model when dealing with unknown domain data. In addition, this paper proposes the multi-region weight fusion convolution module (MWFC), which is essential for the segmentation model to extract feature information from the source domain data accurately. Fusing multiple region weights and convolutional kernel weights on the image to enhance the model adaptability to information at different locations on the image, the fusion of weights deepens the capacity and depth of the model. It enhances the learning ability of the model for multiple regions on the source domain. Our experiments on fundus data for cup/disc segmentation show that the introduction of MSA and MWFC modules in this paper effectively improves the segmentation ability of the segmentation model on the unknown domain. And the performance of the proposed method is significantly better than other methods in the current domain generalization segmentation of the optic cup/disc. • A novel domain generalization segmentation framework is proposed. • A new multi-scale attention mechanism module is proposed. • A novel convolution module is proposed. • Experiments on fundus images show the excellent performance of our method.
更多查看译文
关键词
Multi-scale attention mechanism module (MSA),Multi-region weight fusion convolution module (MWFC),Fundus domain generalization segmentation
AI 理解论文
溯源树
样例
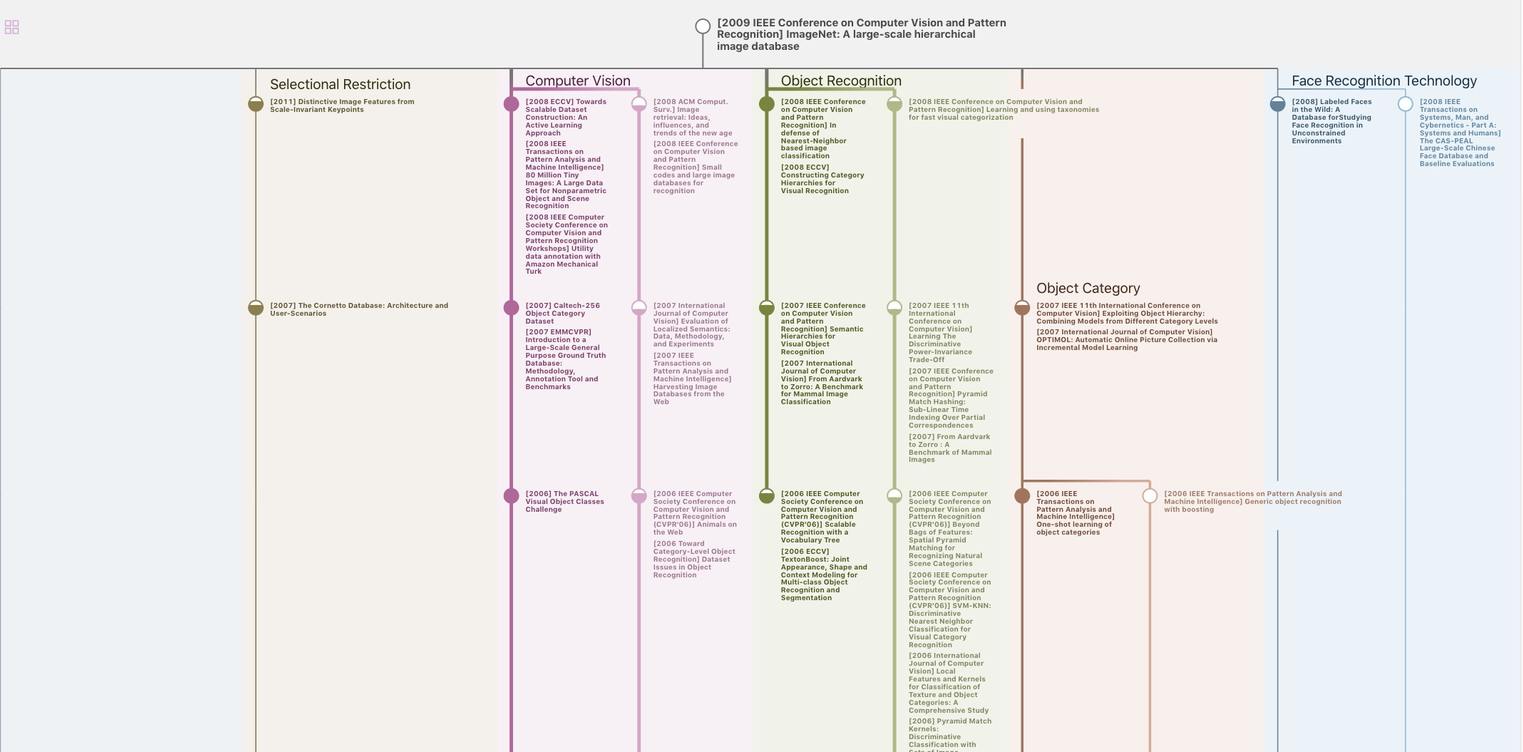
生成溯源树,研究论文发展脉络
Chat Paper
正在生成论文摘要