Incorporating Data Preparation and Clustering Techniques for Workload Segmentation in Large-Scale Cloud Data Centers
2023 Fourth International Conference on Intelligent Data Science Technologies and Applications (IDSTA)(2023)
摘要
The interconnected dependencies of various cloud-hosted services and applications make managing cloud resources more difficult. Observing fully operational virtual computing instances based on task profiles can aid in identifying workload characteristics. Scheduling and managing workloads may be optimized by tailoring selection and decision-making processes in response to workload segmentation. Cloud data center managers use models that group operations and tasks with similar structures, allowing for a more straightforward comparison of outcomes and improved cloud service performance and availability. However, conventional clustering algorithms can cluster the cloud workload into a single data grouping pattern. Since cloud workload data is open to diverse interpretations, several legitimate categories are hiding in the various data outlooks. Considering high-dimensional data, where several attribute profiles define each job, we provide a strategy for grouping cloud workloads at the task scheduling level. The proposed model is applied to a real-world data center workload, which includes a trace of virtual instances derived from the Microsoft Azure public dataset. Several data clustering methods and pipelines are examined and contrasted. The results show that different feature engineering methods used in data pipelines lead to various valid clustering schemes that can be used to improve the performance of workload segmentation in big cloud data centers.
更多查看译文
关键词
Cloud Workload Segmentation,Features Engineering,Clustering Techniques,Cloud Computing
AI 理解论文
溯源树
样例
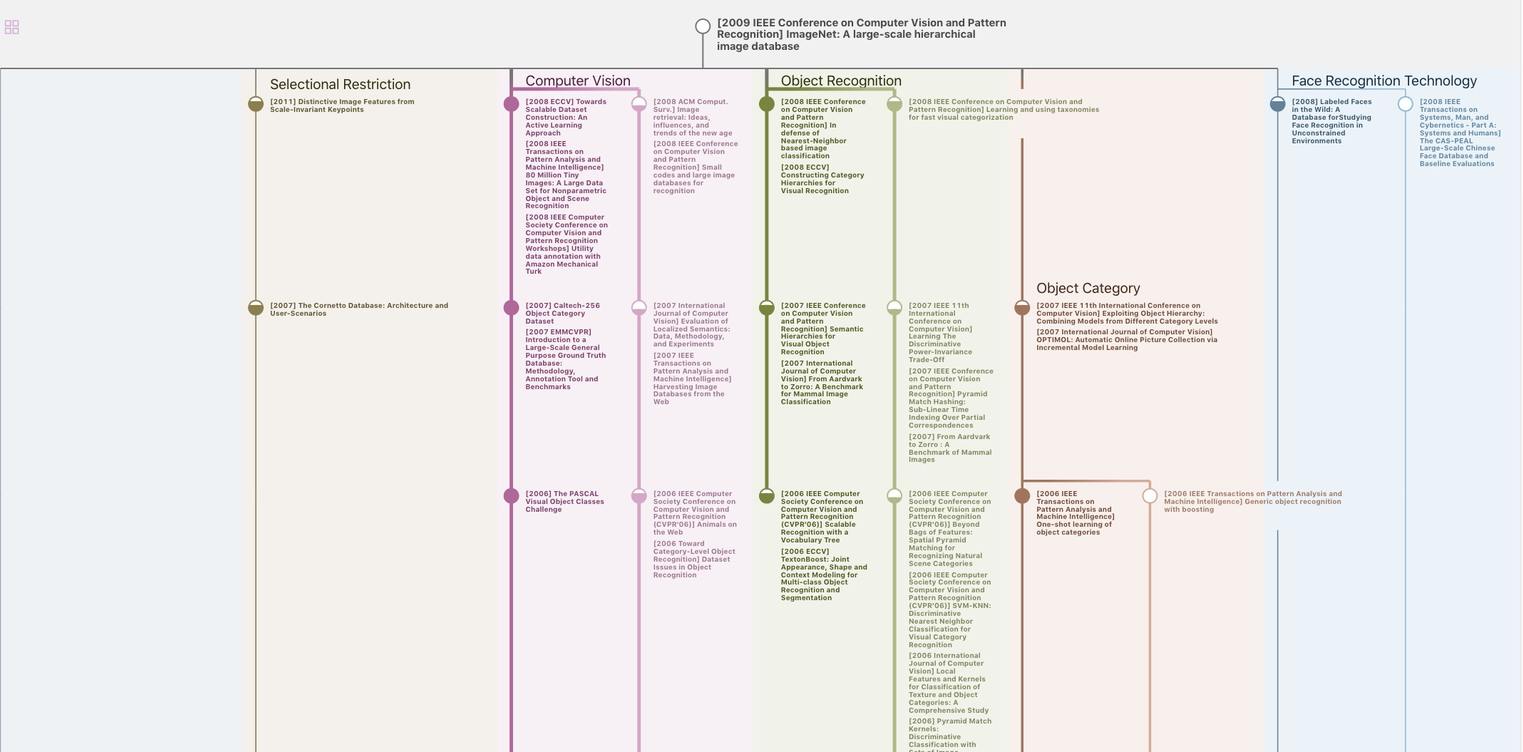
生成溯源树,研究论文发展脉络
Chat Paper
正在生成论文摘要