An Unsupervised Online Streaming Feature Selection Algorithm with Density Peak Clustering
2023 IEEE International Conference on Networking, Sensing and Control (ICNSC)(2023)
摘要
Streaming feature selection (SFS), which enters the feature space in the form of flow and plays an important role in processing high dimensional data. Online streaming feature selection (OSFS) is a real-time streaming feature selection method. Most of the existing online streaming feature selection methods are based on the relevance between feature and label, ignoring the lack of label information, which frequently results in the failure of feature selection. In addition, in numerous practical application scenarios like intelligent medical treatment, label information with the high cost of acquiring label information is missing, which poses a great challenge to feature selection. To solve these problems, a novel unsupervised online streaming feature selection algorithm with density peak clustering (UOSFS-DPC) is proposed to reduce feature redundancy by clustering online streaming features. An online unsupervised relevance analysis and online unsupervised redundancy analysis are also adopted to select important feature subsets from the streaming features. Experimental results on six benchmark datasets and compared studies with eight representative SFS algorithms demonstrate that UOSFS-DPC outperforms its peers when label information is unknown.
更多查看译文
关键词
Online Feature Selection,Feature Stream clustering,Unsupervised Online Learning,Density Clustering,Streaming Feature Selection
AI 理解论文
溯源树
样例
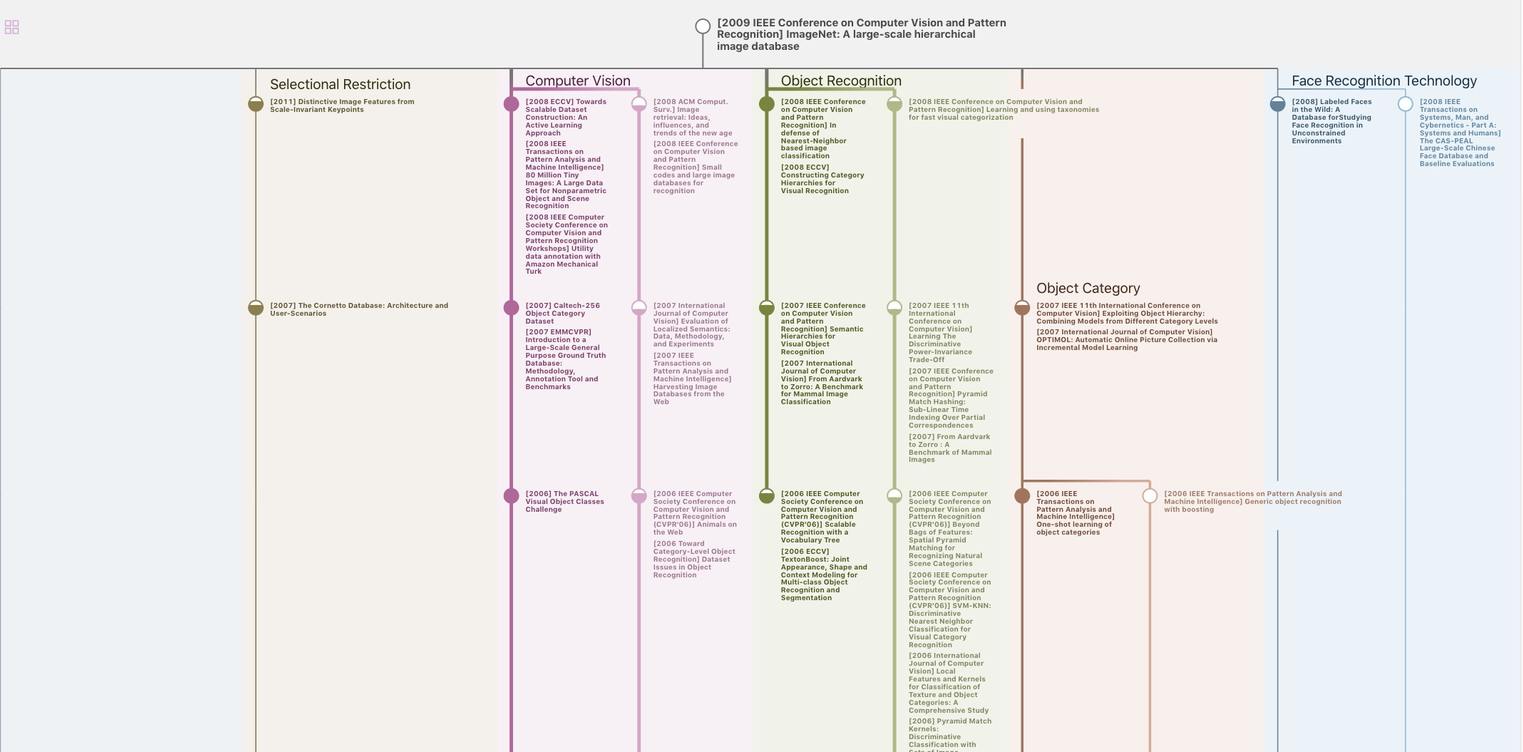
生成溯源树,研究论文发展脉络
Chat Paper
正在生成论文摘要