Learning-Based Control
Foundations and Trends in Systems and Control(2020)
摘要
This monograph presents a new framework for learning-based control synthesis of continuous-time dynamical systems with unknown dynamics. The new design paradigm proposed here is fundamentally different from traditional control theory. In the classical paradigm, controllers are often designed for a given class of dynamical control systems; it is a model-based design. Under the learning-based control framework, controllers are learned online from real-time input–output data collected along the trajectories of the control system in question. An entanglement of techniques from reinforcement learning and model-based control theory is advocated to find a sequence of suboptimal controllers that converge to the optimal solution as learning steps increase. On the one hand, this learning-based design approach attempts to overcome the well-known “curse of dimensionality” and the “curse of modeling” associated with Bellman’s Dynamic Programming. On the other hand, rigorous stability and robustness analysis can be derived for the closed-loop system with real-time learning-based controllers. The effectiveness of the proposed learning-based control framework is demonstrated via its applications to theoretical optimal control problems tied to various important classes of continuous-time dynamical systems and practical problems arising from biological motor control, connected and autonomous vehicles.
更多查看译文
AI 理解论文
溯源树
样例
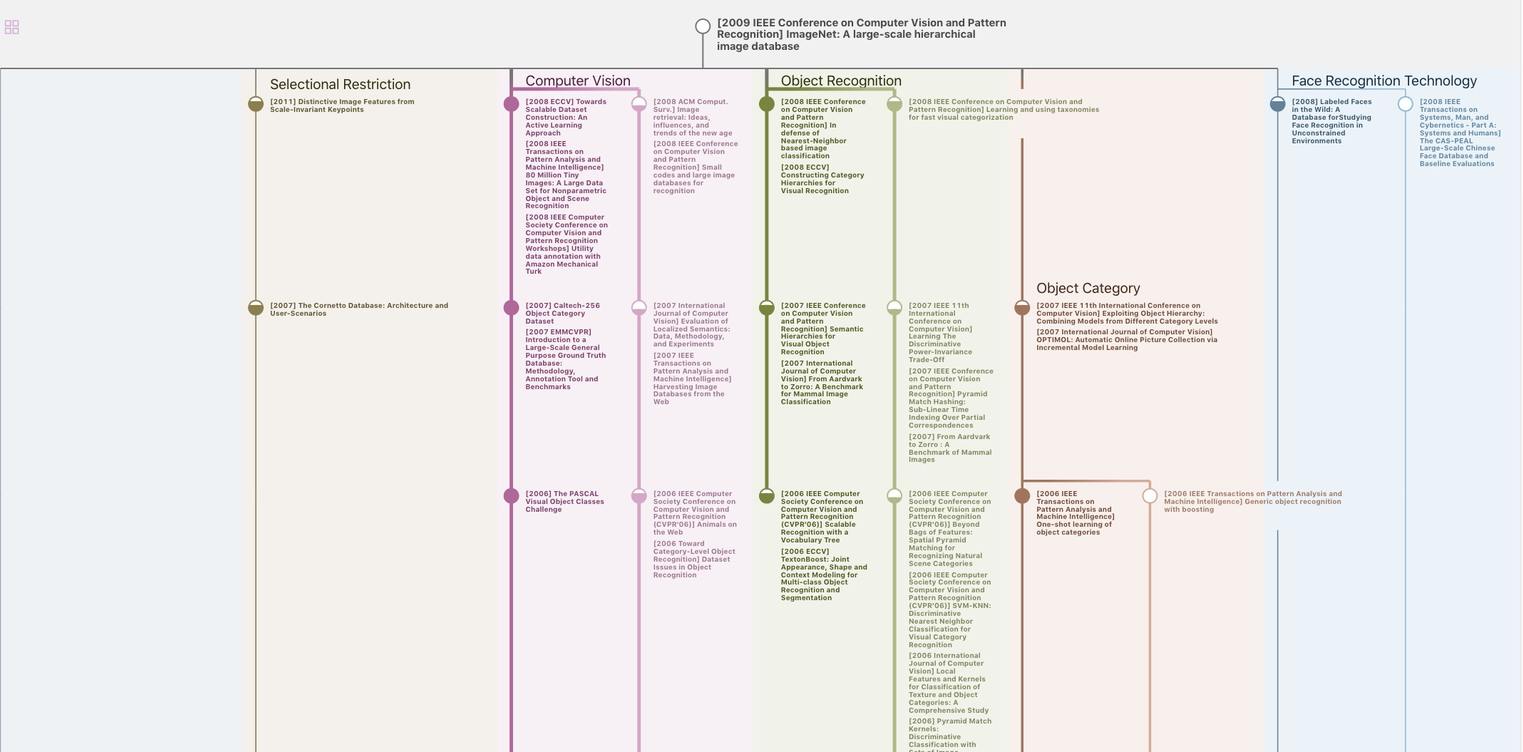
生成溯源树,研究论文发展脉络
Chat Paper
正在生成论文摘要