Generalizing
Machine Language(2022)
摘要
For hypergraph clustering, various methods have been proposed to define hypergraph p -Laplacians in the literature. This work proposes a general framework for an abstract class of hypergraph p -Laplacians from a differential-geometric view. This class includes previously proposed hypergraph p -Laplacians and also includes previously unstudied novel generalizations. For this abstract class, we extend current spectral theory by providing an extension of nodal domain theory for the eigenvectors of our hypergraph p -Laplacian. We use this nodal domain theory to provide bounds on the eigenvalues via a higher-order Cheeger inequality. Following our extension of spectral theory, we propose a novel hypergraph partitioning algorithm for our generalized p -Laplacian. Our empirical study shows that our algorithm outperforms spectral methods based on existing p -Laplacians.
更多查看译文
关键词
Spectral clustering,Hypergraph learning,Hypergraph p-Laplacian,Cheeger inequality
AI 理解论文
溯源树
样例
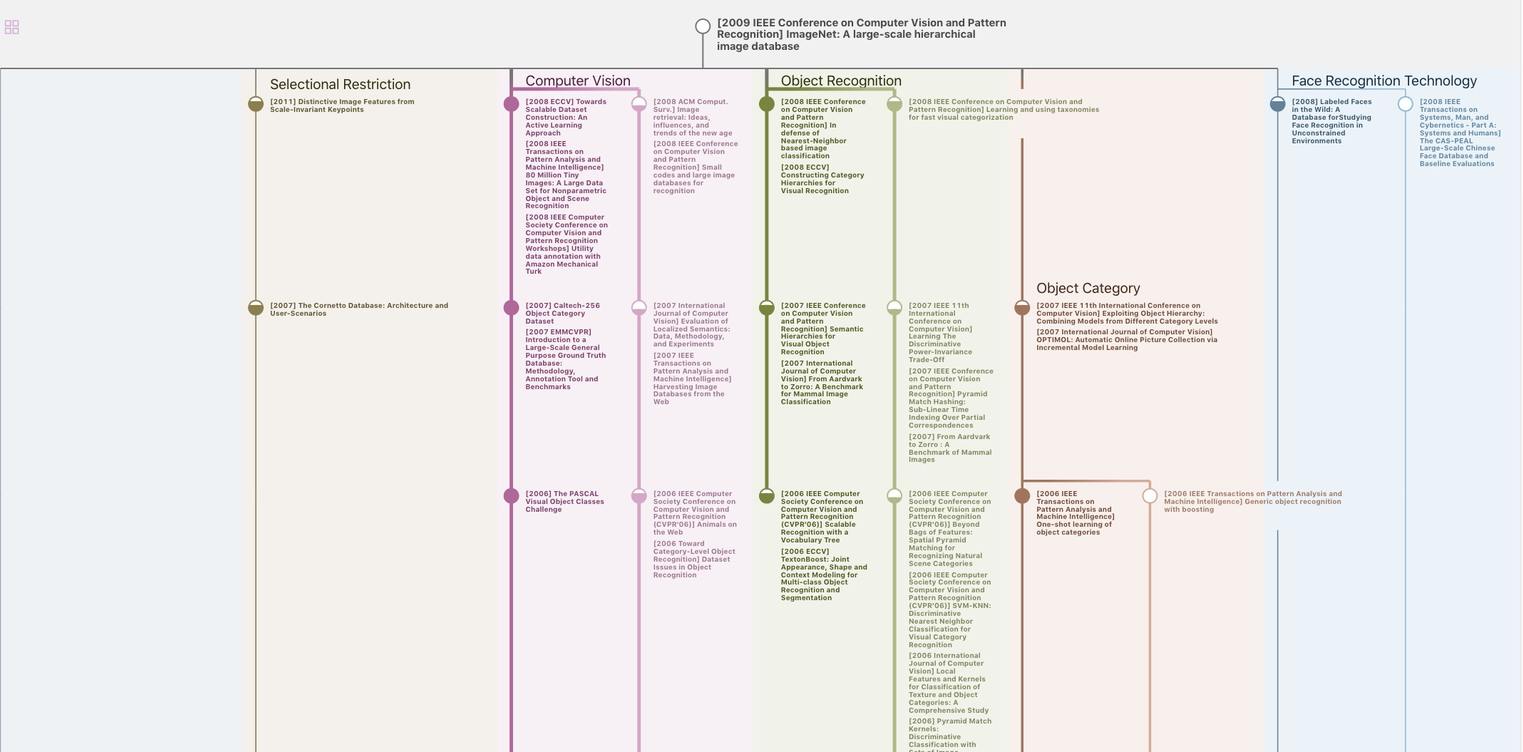
生成溯源树,研究论文发展脉络
Chat Paper
正在生成论文摘要