Color-UNet++: A resolution for colorization of grayscale images using improved UNet++
Multimedia Tools and Applications(2021)
摘要
Colorization is the computer-assisted application of color to a gray scale image, which presents two problems to modern deep learning-based approaches. One is to provide colorization models with both high expressibility and strong learning ability, as current models have difficulty both excelling at coloring and being easy to train. The other is to return a picture without uneven overlap. This paper proposes a deep convolutional network framework called Color-UNet++ for the end-to-end solution of these colorization problems. Color-UNet++ is adjusted to settle gradient dispersion and explosion by capturing more transfer and intermediate results during backpropagation. We adjust the de-convolution structure to solve the problem of uneven overlap. We design the model in YUV instead of RGB color space, with an objective function that is appropriate to the coloring problem and can capture a wide range of colors. A large number of experimental results on LFW and LSUN datasets confirm the method’s superiority.
更多查看译文
关键词
Colorization,Deep learning,Convolutional netural network,Image processing
AI 理解论文
溯源树
样例
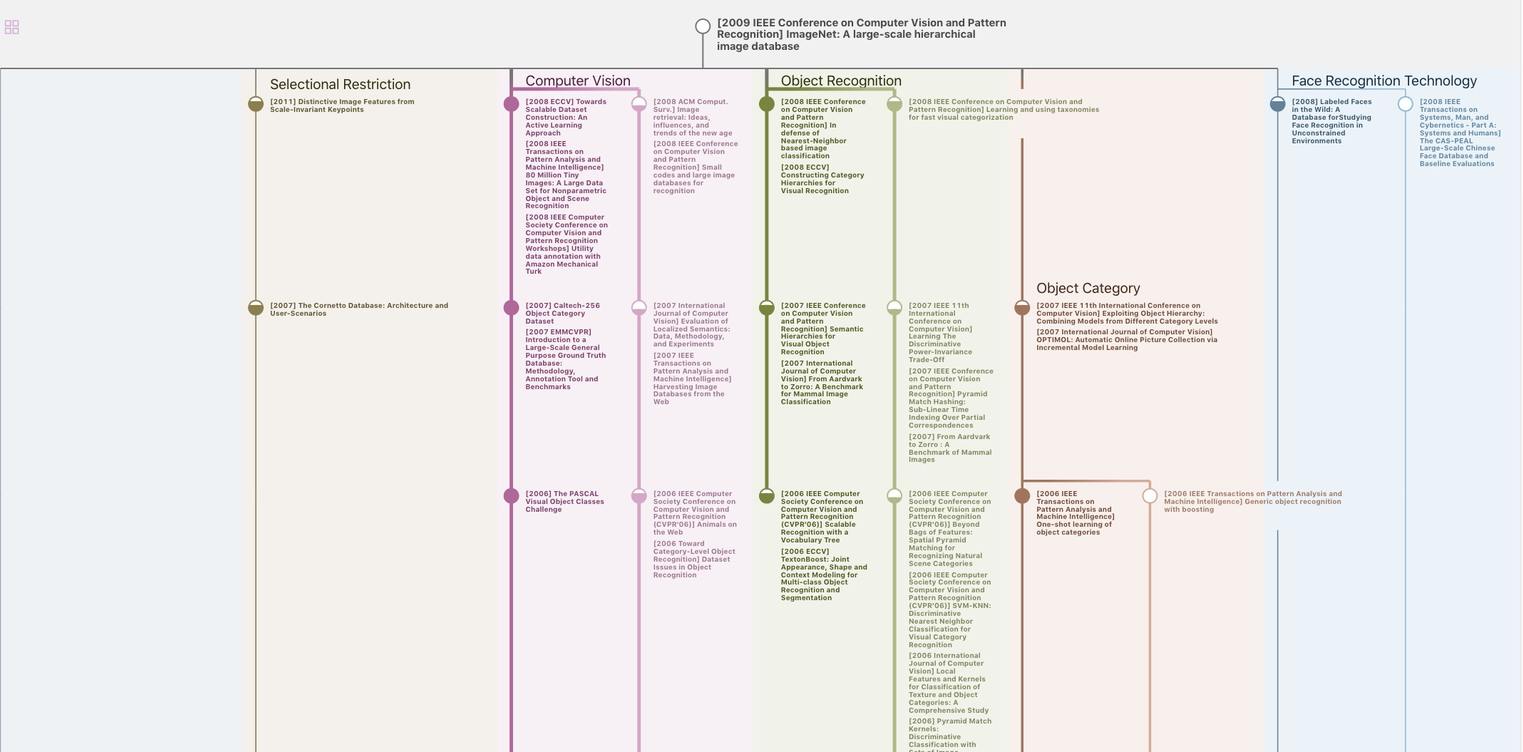
生成溯源树,研究论文发展脉络
Chat Paper
正在生成论文摘要