Cost Explosion for Efficient Reinforcement Learning Optimisation of Quantum Circuits
2023 IEEE International Conference on Rebooting Computing (ICRC)(2023)
摘要
Large scale optimisation of quantum circuits is a computationally challenging problem. Reinforcement Learning (RL) is a recent approach for learning strategies to optimise quantum circuits by increasing the reward of an optimisation agent. The reward is a function of the quantum circuit costs, such as gate and qubit counts, or circuit depth. Our goal is to improve the agent's optimization strategy, by including hints about how quantum circuits are optimized manually: there are situations when the cost of a circuit should be allowed to temporary explode, before applying optimisations which significantly reduce the circuit's cost. We bring numerical evidence, using Bernstein-Vazirani circuits, to support the advantage of this strategy. Our results are preliminary, and show that allowing cost explosions offers significant advantages for RL training, such as reaching optimum circuits. Cost explosion strategies have the potential to be an essential tool for RL of large-scale quantum circuit optimisation.
更多查看译文
AI 理解论文
溯源树
样例
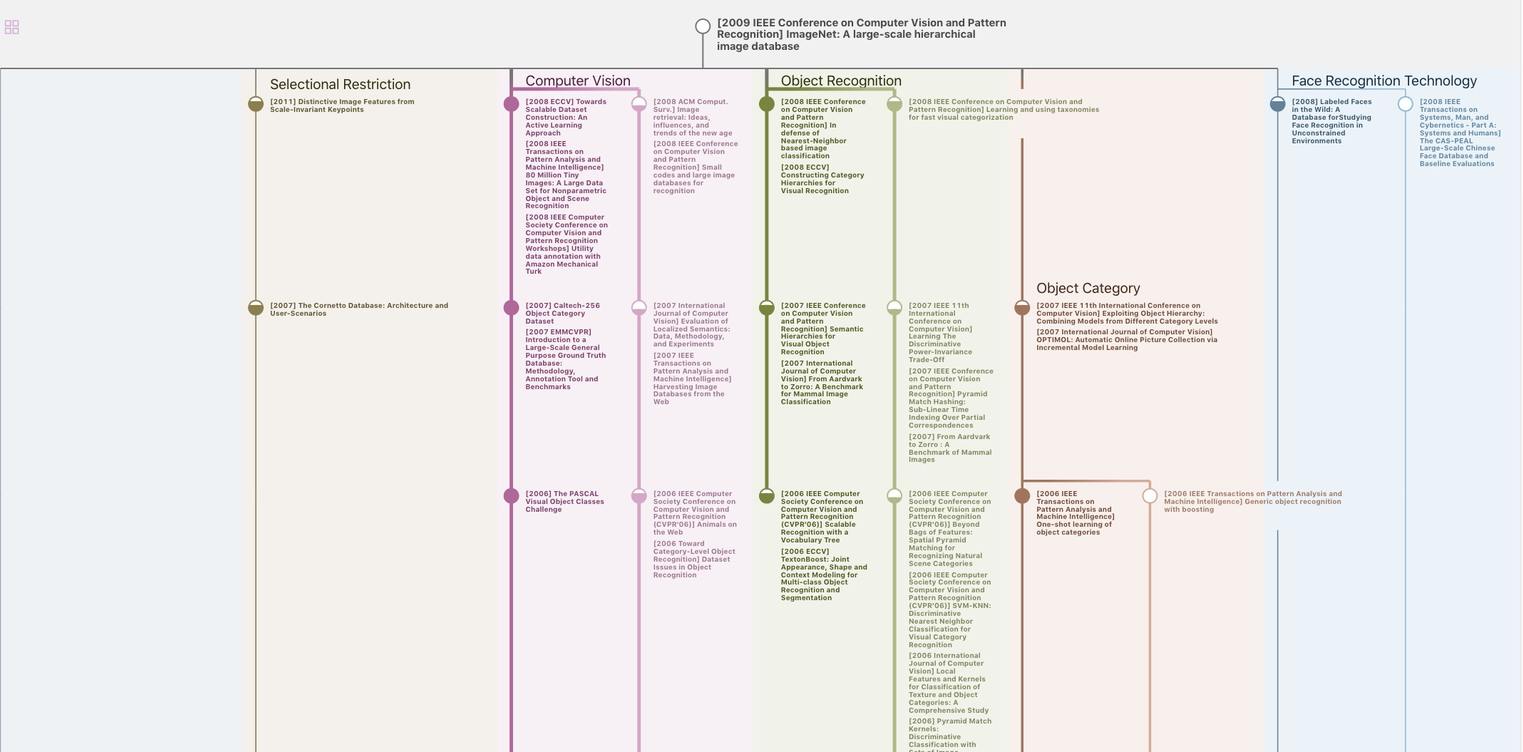
生成溯源树,研究论文发展脉络
Chat Paper
正在生成论文摘要