Learning to optimise wind farms with graph transformers
APPLIED ENERGY(2024)
摘要
This work proposes a novel data -driven model capable of providing accurate predictions for the power generation of all wind turbines in wind farms of arbitrary layout, yaw angle configurations and wind conditions. The proposed model functions by encoding a wind farm into a fully connected graph and processing the graph representation through a graph transformer. The resultant graph transformer surrogate demonstrates robust generalisation capabilities and effectively uncovers latent structural patterns embedded within the graph representation of wind farms. The versatility of the proposed approach extends to the optimisation of yaw angle configurations through the application of genetic algorithms. This evolutionary optimisation strategy facilitated by the graph transformer surrogate achieves prediction accuracy levels comparable to industrially standard wind farm simulation tools, with a relative accuracy of more than 99% in identifying optimal yaw angle configurations of previously unseen wind farm layouts. An additional advantage lies in the significant reduction in computational costs, positioning the proposed methodology as a compelling tool for efficient and accurate wind farm optimisation.
更多查看译文
关键词
Deep learning,Transformers,Graph neural networks,Genetic algorithms,Wind farm power,Wake steering optimisation
AI 理解论文
溯源树
样例
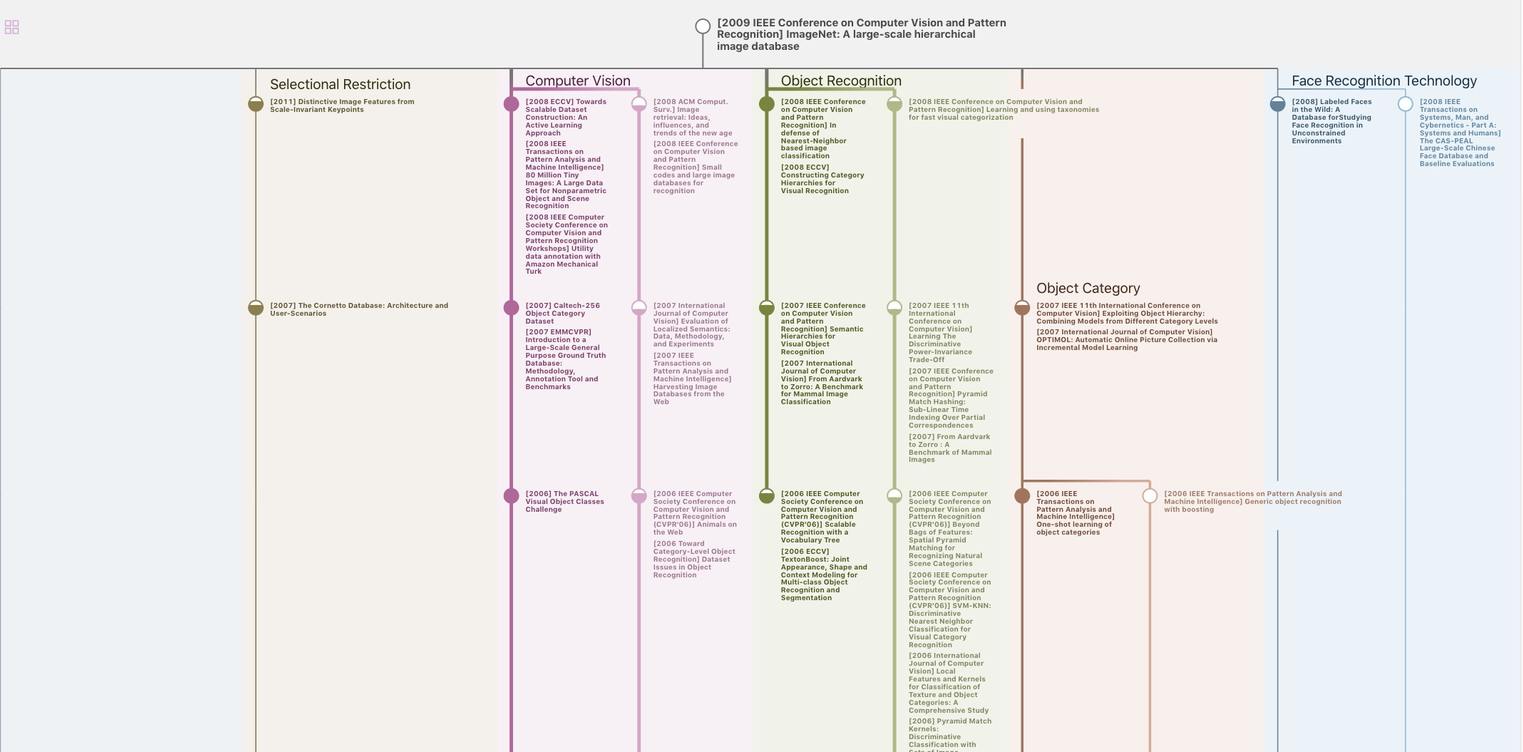
生成溯源树,研究论文发展脉络
Chat Paper
正在生成论文摘要