Sensitivity analysis with multiple treatments and multiple outcomes with applications to air pollution mixtures
arxiv(2023)
摘要
Understanding the health impacts of air pollution is vital in public health
research. Numerous studies have estimated negative health effects of a variety
of pollutants, but accurately gauging these impacts remains challenging due to
the potential for unmeasured confounding bias that is ubiquitous in
observational studies. In this study, we develop a framework for sensitivity
analysis in settings with both multiple treatments and multiple outcomes
simultaneously. This setting is of particular interest because one can identify
the strength of association between the unmeasured confounders and both the
treatment and outcome, under a factor confounding assumption. This provides
informative bounds on the causal effect leading to partial identification
regions for the effects of multivariate treatments that account for the maximum
possible bias from unmeasured confounding. We also show that when negative
controls are available, we are able to refine the partial identification
regions substantially, and in certain cases, even identify the causal effect in
the presence of unmeasured confounding. We derive partial identification
regions for general estimands in this setting, and develop a novel
computational approach to finding these regions.
更多查看译文
AI 理解论文
溯源树
样例
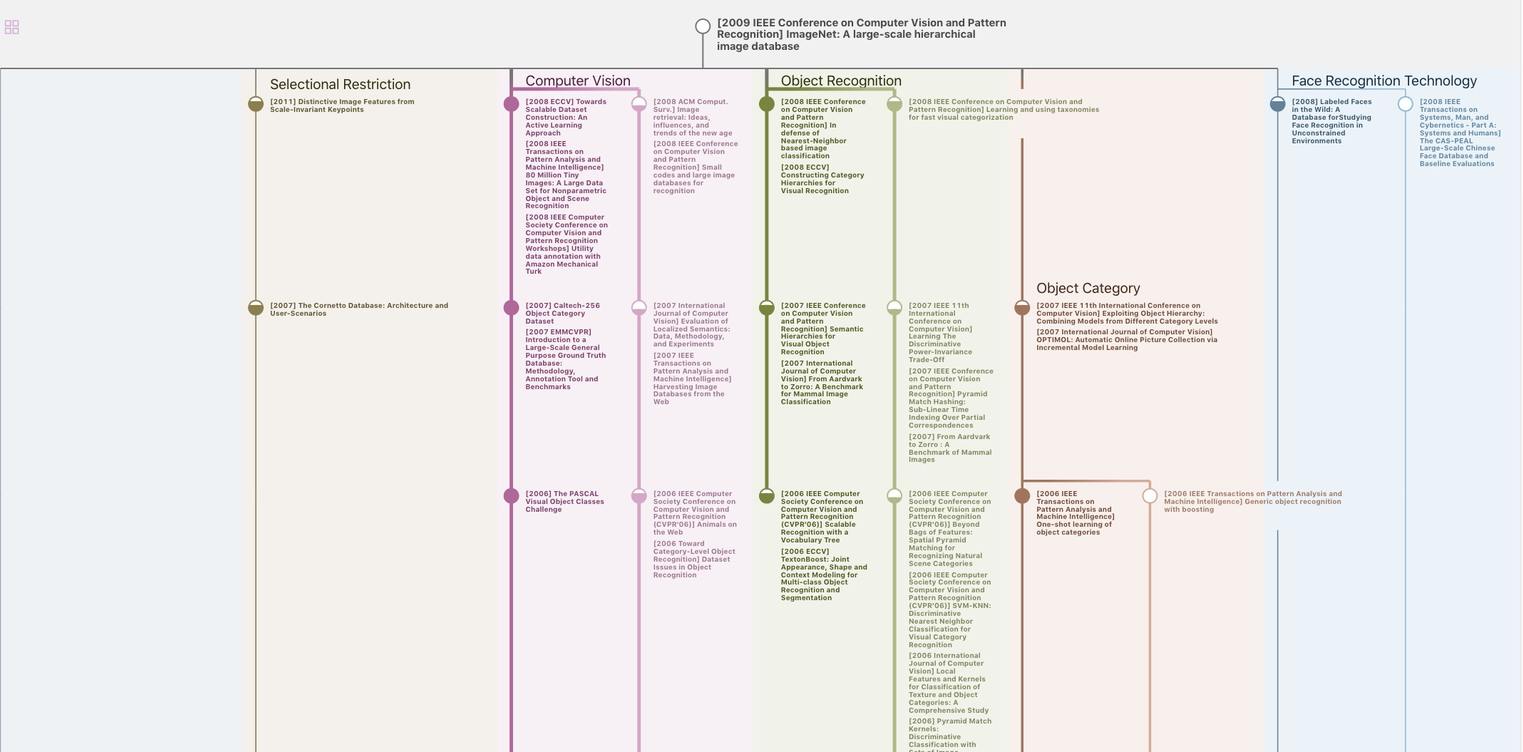
生成溯源树,研究论文发展脉络
Chat Paper
正在生成论文摘要