Verifying in the Dark: Verifiable Machine Unlearning by Using Invisible Backdoor Triggers
IEEE TRANSACTIONS ON INFORMATION FORENSICS AND SECURITY(2024)
摘要
Machine unlearning as a fundamental requirement in Machine-Learning-as-a-Service (MLaaS) has been extensively studied with increasing concerns about data privacy. It requires MLaaS providers should delete training data upon user requests. Unfortunately, none of the existing studies can efficiently achieve machine unlearning validation while preserving the retraining efficiency and the service quality after data deletion. Besides, how to craft the validation scheme to prevent providers from spoofing validation by forging proofs remains under-explored. In this paper, we introduce a backdoor-assisted validation scheme for machine unlearning. The proposed design is built from the ingenious combination of backdoor triggers and incremental learning to assist users in verifying proofs of machine unlearning without compromising performance and service quality. We propose to embed invisible markers based on backdoor triggers into privacy-sensitive data to prevent MLaaS providers from distinguishing poisoned data for validation spoofing. Users can use prediction results to determine whether providers comply with data deletion requests. Besides, we incorporate our validation scheme into an efficient incremental learning approach via our index structure to further facilitate the performance of retraining after data deletion. Evaluation results on real-world datasets confirm the efficiency and effectiveness of our proposed verifiable machine unlearning scheme.
更多查看译文
关键词
Machine unlearning,ML-as-a-service,backdoor attacks,incremental learning
AI 理解论文
溯源树
样例
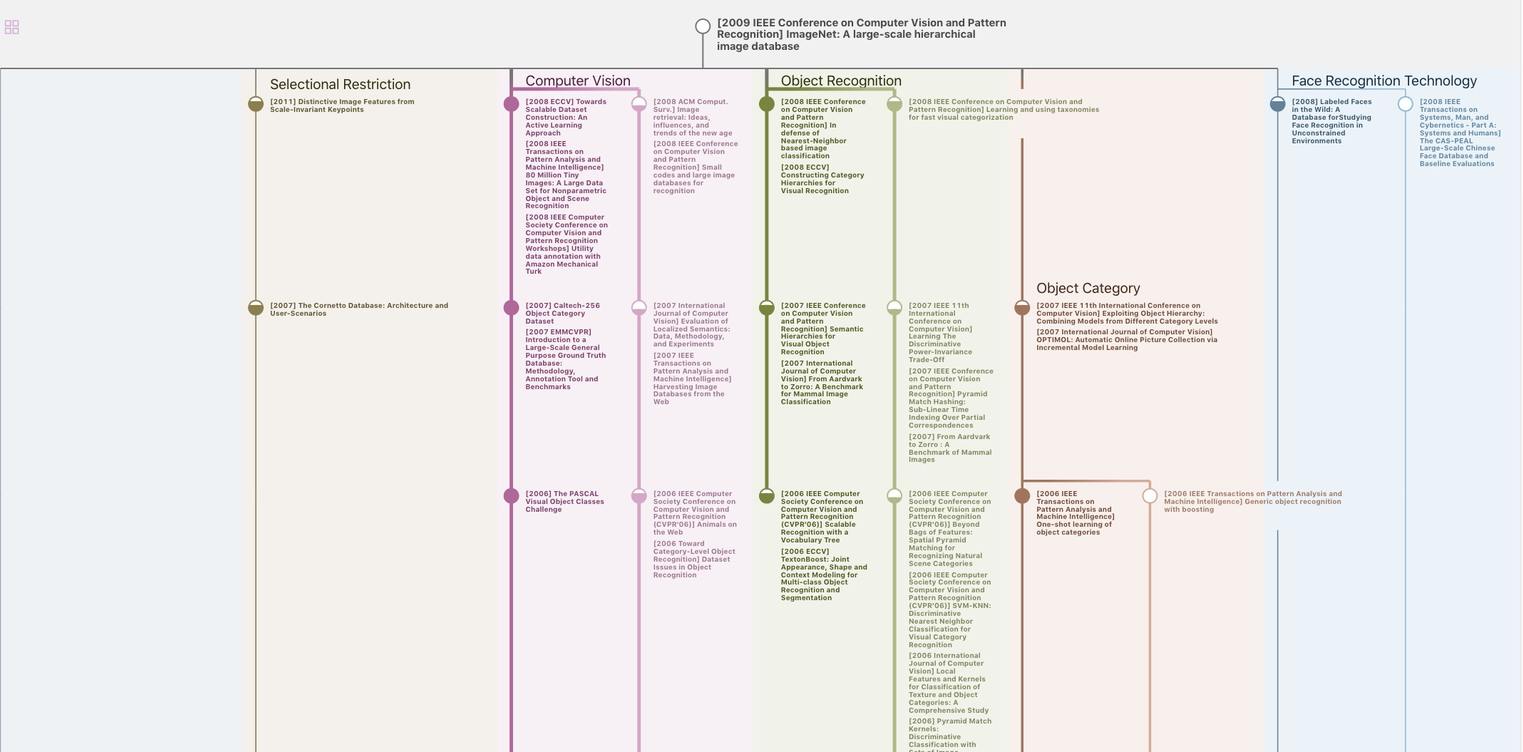
生成溯源树,研究论文发展脉络
Chat Paper
正在生成论文摘要