TES-Net: Temporal Excitation Shift for Action Recognition
2023 IEEE International Conference on Unmanned Systems (ICUS)(2023)
摘要
In order to model fine-grained temporal information in human action videos, this paper proposes a new video structure - temporal excitation shift network TES. This model incorporates TES temporal excitation shift modules into each residual block of the ResNet50 network, enabling the network to better learn short-term motion features in videos. The TES module acts on the input feature map and consists of two parts: the temporal excitation module TEM allocates weights along the temporal dimension of the input feature map, highlighting video frames that have a significant impact on classification results through attention; The temporal shift module TSM partially shifts and exchanges the input feature map along the channel dimension, so that the features of each video frame obtain feature information from adjacent frames, thereby implicitly extracting motion features. The TES module can be well integrated into the ResNet50 network and time segmented network to fully capture short-term motion features and long-term temporal information in videos. The experimental results show that the TES model proposed in this article effectively extracts the spatiotemporal features of human actions in videos. It achieved recognition results of 85.63%, 49.91%, 73.5%, 95.53%, and 76.1% on the Diving48, Something-V1, Kinetic400, UCF101, and Hmdb51 datasets, respectively. The inference speed of a single video reached 0.035 seconds. Therefore, the TES model achieved a good balance between recognition accuracy and speed.
更多查看译文
关键词
human action recognition,temporal excitation,temporal shift
AI 理解论文
溯源树
样例
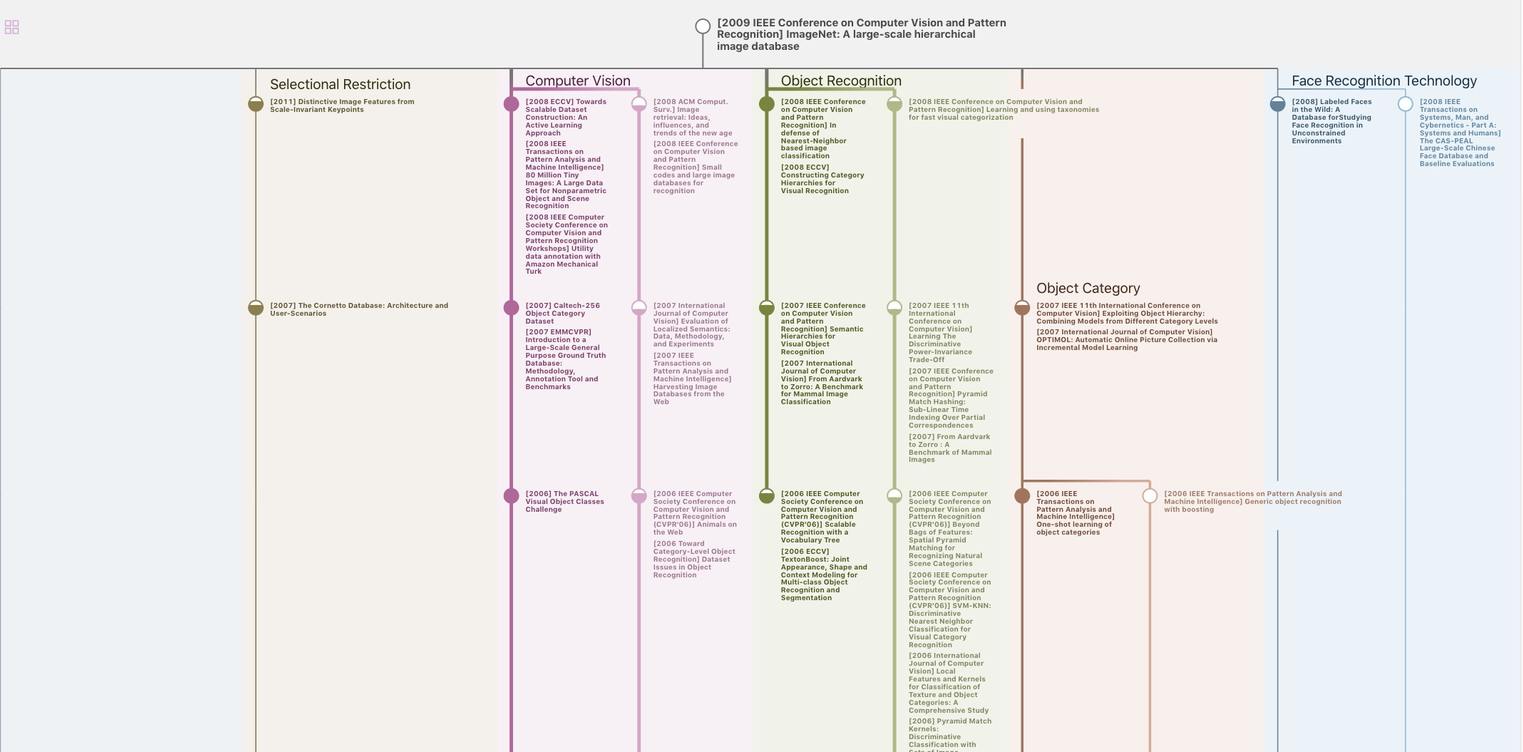
生成溯源树,研究论文发展脉络
Chat Paper
正在生成论文摘要