Prediction of Head-Neck Cancer Recurrence from Pet/CT Images with Havrda-Charvat Entropy
2023 Twelfth International Conference on Image Processing Theory, Tools and Applications (IPTA)(2023)
摘要
This paper proposes a loss function based on Havrda–Charvat entropy in deep neural networks for outcome prediction in head–neck cancers. Havrda-Charvat is a parameterized cross-entropy which generalizes the classical Shannon-based cross-entropy. Its parameter denoted α takes its values in ]0, ∞[ and one can recover some usual entropies, for instance Shannon for α = 1 or Gini coefficient for α = 2. In this paper, we propose to use this entropy to predict cancer recurrence by incorporating it in a neural network instead of Shannon’s entropy for better adaptability. Our deep network is composed of a double auto-encoder to extract features and a classifier to predire cancer outcome. The experiments are conducted on MICCAI challenge dataset of Head-Neck cancer. The influence of the parameter on the results is studied and an optimal interval of its values is found. A result shows that Havrda–Charvat entropy can achieve better prediction performance than Shannon entropy, which is the most widely used in prediction task nowadays.
更多查看译文
关键词
Deep neural networks,Shannon entropy,Havrda–Charvat entropy,generalized entropies,recurrence prediction,head–neck cancer,parameter estimation,CT images,PET images
AI 理解论文
溯源树
样例
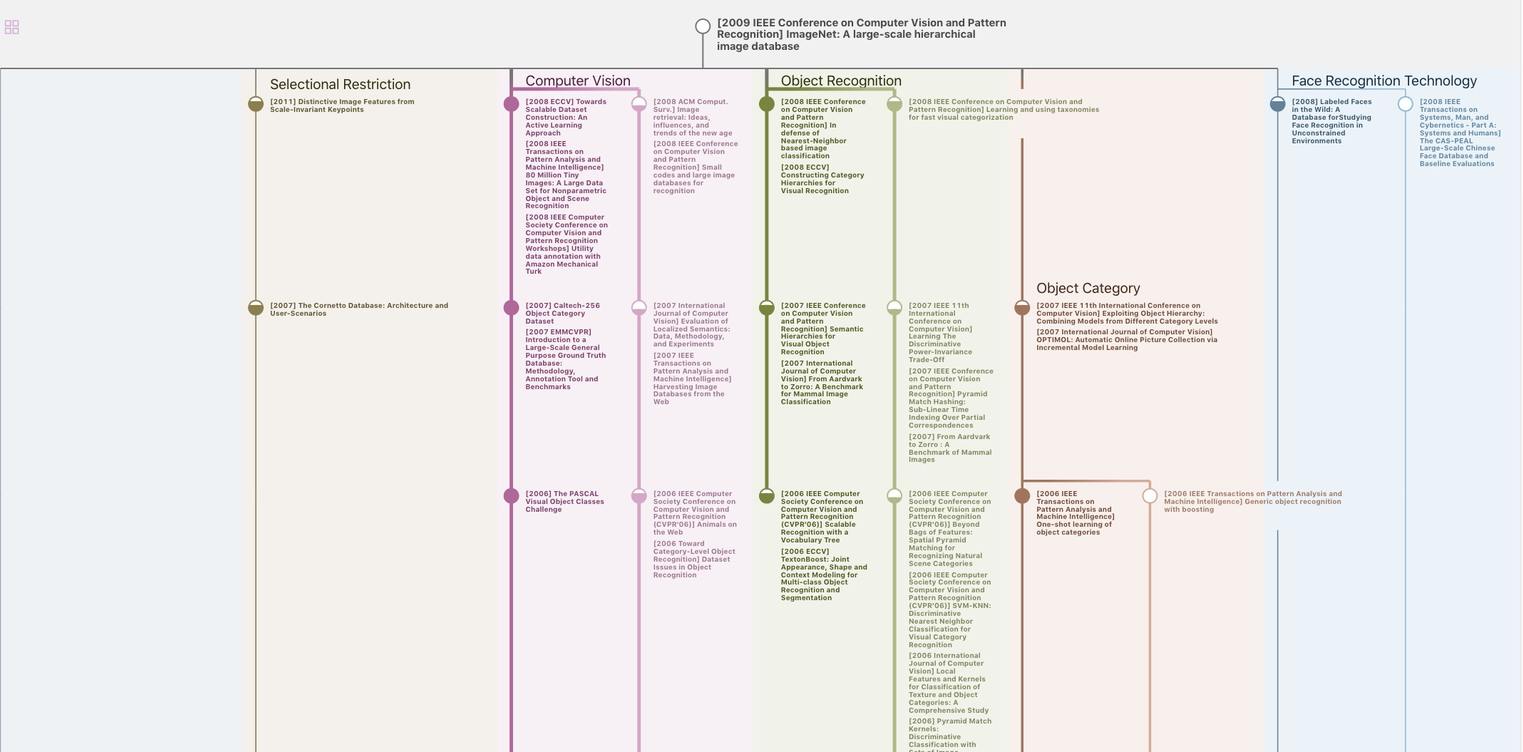
生成溯源树,研究论文发展脉络
Chat Paper
正在生成论文摘要