Deep Learning-Driven Library Design for the De Novo Discovery of Bioactive Thiopeptides
ACS CENTRAL SCIENCE(2023)
摘要
Broad substrate tolerance of ribosomally synthesized and post-translationally modified peptide (RiPP) biosynthetic enzymes has allowed numerous strategies for RiPP engineering. However, despite relaxed specificities, exact substrate preferences of RiPP enzymes are often difficult to pinpoint. Thus, when designing combinatorial libraries of RiPP precursors, balancing the compound diversity with the substrate fitness can be challenging. Here, we employed a deep learning model to streamline the design of mRNA display libraries. Using an in vitro reconstituted thiopeptide biosynthesis platform, we performed mRNA display-based profiling of substrate fitness for the biosynthetic pathway involving five enzymes to train an accurate deep learning model. We then utilized the model to design optimal mRNA libraries and demonstrated their utility in affinity selections against IRAK4 kinase and the TLR10 cell surface receptor. The selections led to the discovery of potent thiopeptide ligands against both target proteins (K-D up to 1.3 nM for the best compound against IRAK4 and 300 nM for TLR10). The IRAK4-targeting compounds also inhibited the kinase at single-digit mu M concentrations in vitro, exhibited efficient internalization into HEK293H cells, and suppressed NF-kB-mediated signaling in cells. Altogether, the developed approach streamlines the discovery of pseudonatural RiPPs with de novo designed biological activities and favorable pharmacological properties.
更多查看译文
关键词
bioactive thiopeptides,discovery,learning-driven
AI 理解论文
溯源树
样例
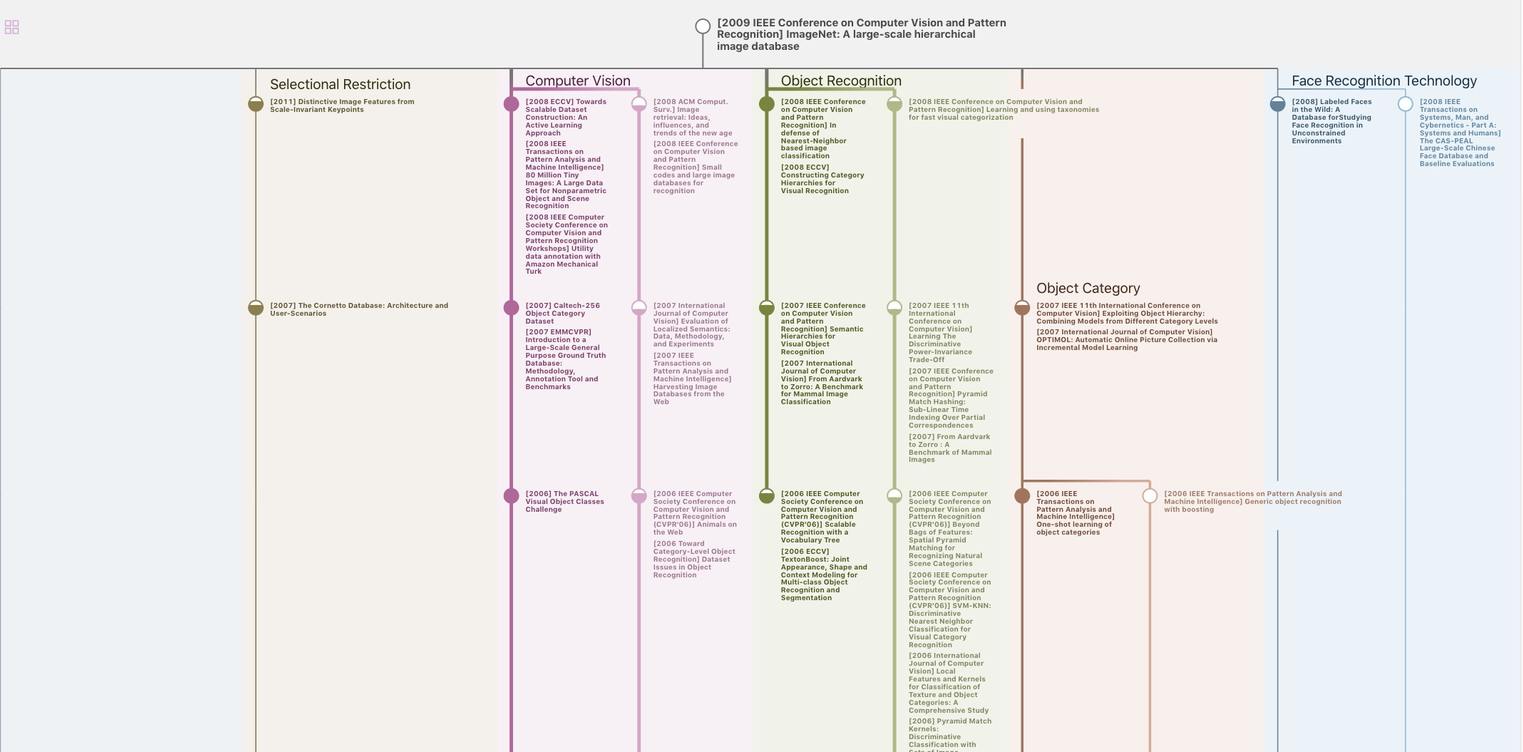
生成溯源树,研究论文发展脉络
Chat Paper
正在生成论文摘要