Efficient Scanning Activity Detection in IoT Networks Using Ensemble Learning.
AIMLR '23: Proceedings of the 2023 Asia Conference on Artificial Intelligence, Machine Learning and Robotics(2023)
摘要
The rapidly expanding universe of Internet of Things (IoT) networks has led to greater security risks due to the proliferation of sophisticated threats such as scanning activities, where adversaries use scanning to identify and exploit vulnerabilities in target IoT devices, which often have limited computing power and storage, making them attractive targets; to address this escalating challenge, this research proposes a comprehensive ensemble learning methodology specifically tailored for robust and timely detection of scanning activities in IoT networks, integrating a diverse set of base classifiers including Support Vector Machine (SVM), Neural Network (NN), Decision Trees (DT), and Linear Regression (LR), with predictions from the base models combined using a Linear Regression meta-classifier into a stacked ensemble model; extensive comparative evaluation on an IoT network dataset demonstrates the proposed ensemble technique achieves significantly higher accuracy, detection rate, F-measure, precision, and Area Under ROC Curve (AUC) versus individual traditional machine learning models, and remarkably, exhibits consistent performance across different cross-validation folds, highlighting its inherent stability and validity, suggesting it generalizes well without overfitting; in summary, this research puts forward an efficient and highly effective ensemble learning solution for the timely and precise detection of scanning attacks against IoT networks, serving as a vital step towards strengthening security against escalating threats..
更多查看译文
AI 理解论文
溯源树
样例
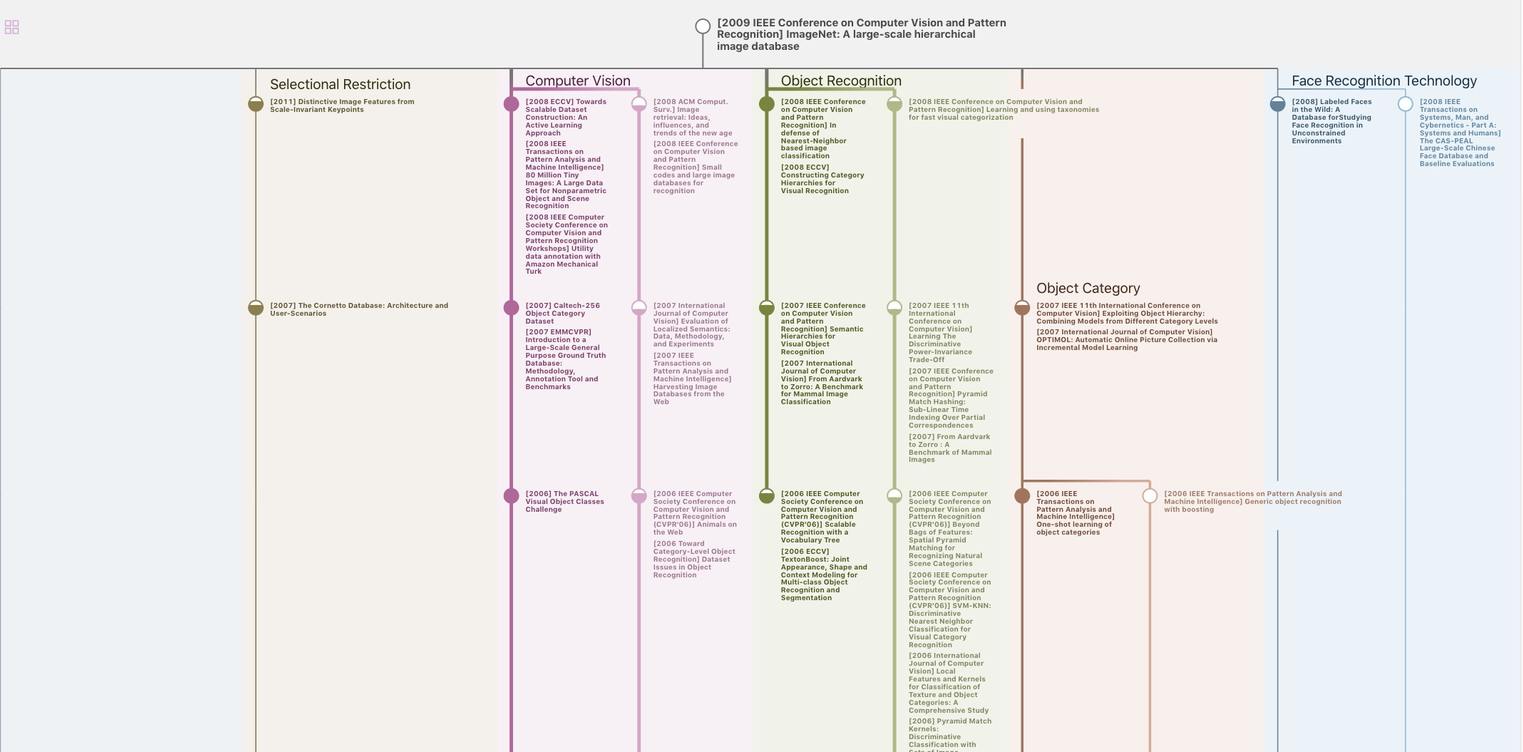
生成溯源树,研究论文发展脉络
Chat Paper
正在生成论文摘要