Practical and Efficient Secure Aggregation for Privacy-Preserving Machine Learning.
AIMLR '23: Proceedings of the 2023 Asia Conference on Artificial Intelligence, Machine Learning and Robotics(2023)
摘要
In recent years, Federal Learning has received much attention becourse it can train models by updating gradients without contacting users’ true data. However, adversaries also can track users’ privacy from the shared gradient. In this paper, we aim to solve three major issues during the process of federated learning: 1) how to protect users’ privacy during training; 2) How to verify the correctness of the aggregation results returned from the server; 3) How to reduce communication costs while ensuring training security. So we propose a verifiable aggregation scheme that can effectively verify the results of server aggregation. Specifically, we follow the classic double mask aggregation scheme, and use Paillier homomorphic encryption algorithm to implement the message authentication code with additive homomorphic property. Users can compare their local codes with server’s aggregation results to verify the correctness of the aggregation results and improve model’s accuracy. In our framework, we adopt a Top-k gradient selection scheme to reduce models’ communication and computing overhead. Experimental results indicate that our training framework is feasible and efficient.
更多查看译文
AI 理解论文
溯源树
样例
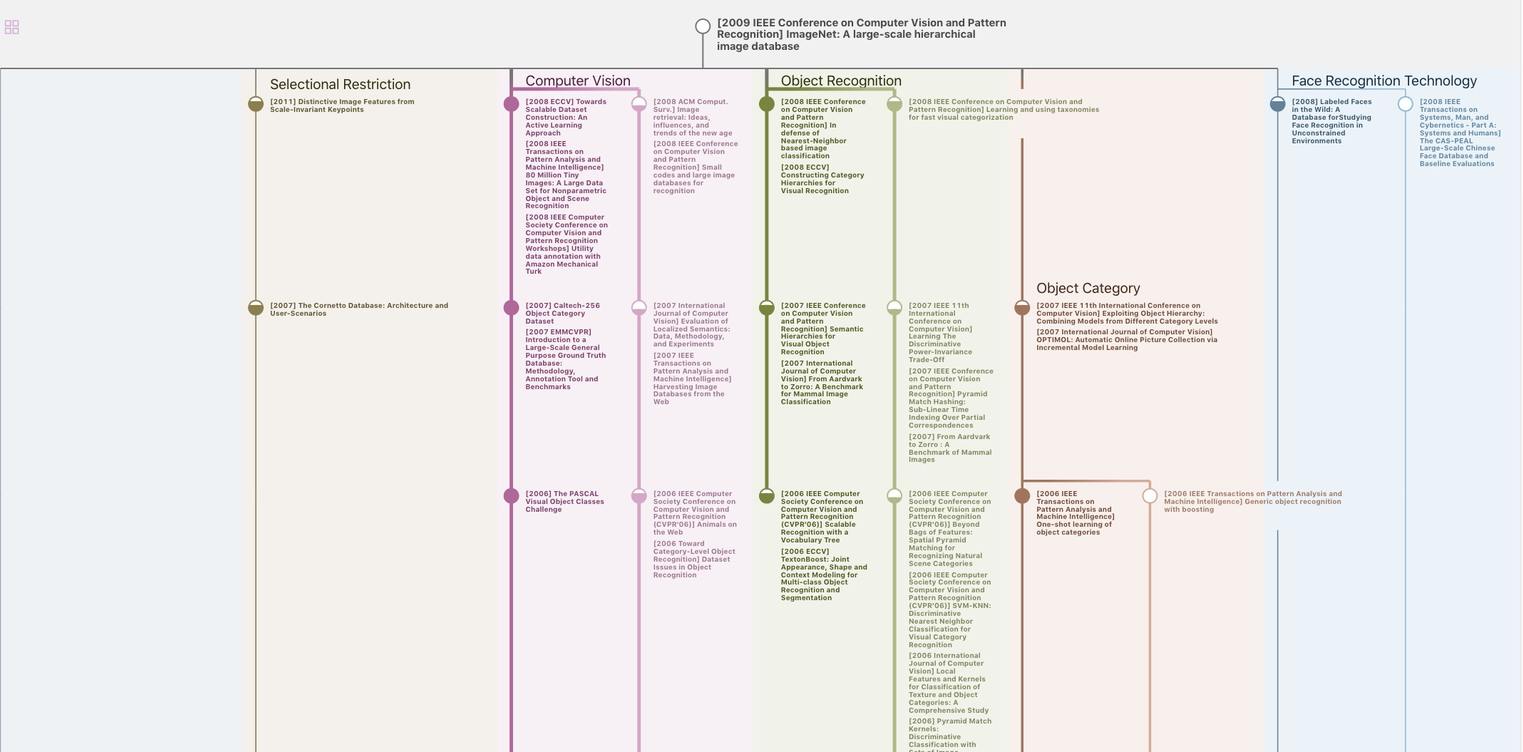
生成溯源树,研究论文发展脉络
Chat Paper
正在生成论文摘要